Since 2013, the intellectual home of AI2050 Early Career Fellow Jennifer Ngadiuba’s research has been at CERN, the European Organization for Nuclear Research. Her participation at CERN started with her PhD studies at the University of Zurich and continued more recently through a postdoctoral appointment at Caltech and a research fellowship at Fermilab, where she is an associate scientist on the particle physics team.
Ngadiuba’s expertise is analyzing data produced by high energy particle collisions. These fantastically complex experiments produce far too much data to capture it all. Instead, high-speed electronics and computers evaluate and reduce the data in real-time, deciding what to keep and what to throw away.
The problem with this approach, says Ngadiuba, is that experiments on the particle accelerators are designed to find or measure a specific particle or interaction, and as a result they may be discarding data that does not agree with the “standard model” of particle physics that has dominated the field for the past fifty years.
This is where Ngadiuba’s AI2050 project comes in, which is developing novel techniques and algorithms based on deep learning to separate signals from noise—signals that might open a window onto the next big advance in particle physics. This is Hard Problem #4, which is realizing the great potential of AI for scientific discovery.
“The idea here is to perform real-time inference (few microseconds) of deep neural networks on AI-oriented electronic devices to ensure that physics is preserved for new and exciting discoveries,” says Ngadiuba.
Ngadiuba is a member of the CMS Collaboration, which operates and collects data from the Compact Muon Solenoid, one of the general-purpose particle detectors at CERN’s Large Hadron Collider (the world’s largest and most powerful particle accelerator).
Learn more about Jennifer Ngadiuba:
Can you describe the CMS?
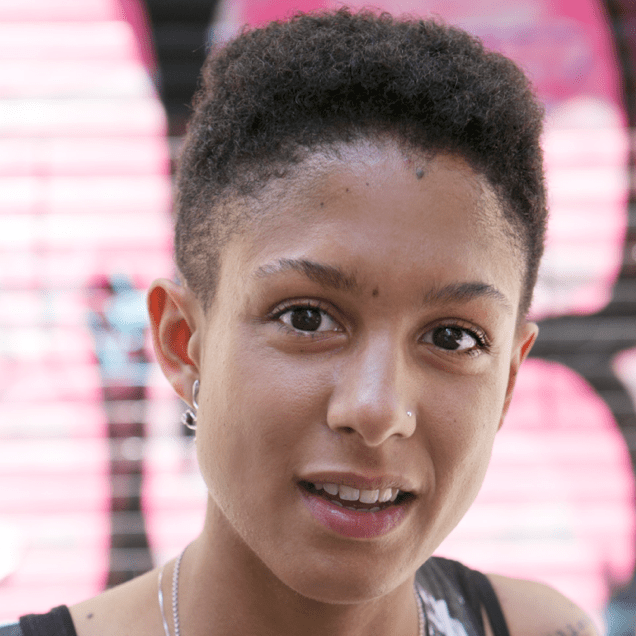
At CMS we have many groups dealing with very different topics. We have separate physics objects groups that deal with reconstruction and identification of jets, or electrons [or other elementary particles].
The experiments are extremely complex as well as the physics we can do by studying collisions. It’s impossible to follow everything, although one tries to be involved in a few different aspects simultaneously.
How did you first get interested in high energy physics?
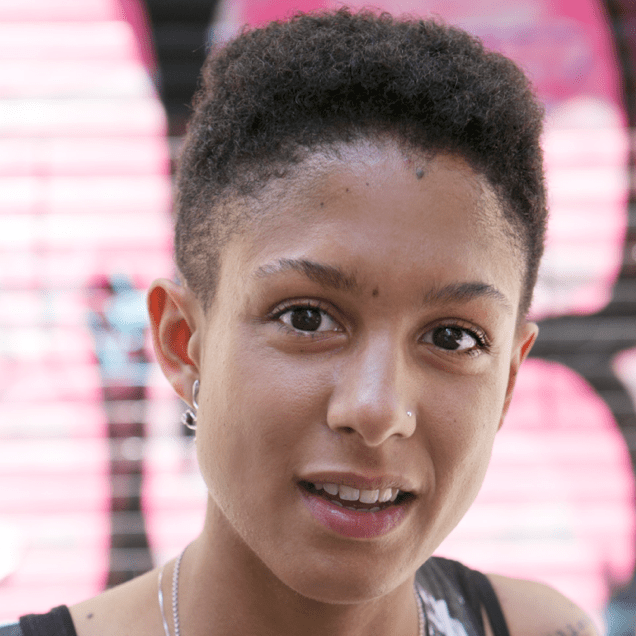
I developed my interest in scientific subjects already in high school. I guess what excited me particularly in physics was that it gives a rational and fundamental answer to what we see around us and mainly natural phenomena — from the movement of sky objects to the magnets attached to my fridge. It felt like a revelation.
How did you join the CMS Collaboration? Is everybody who works at CERN a member?
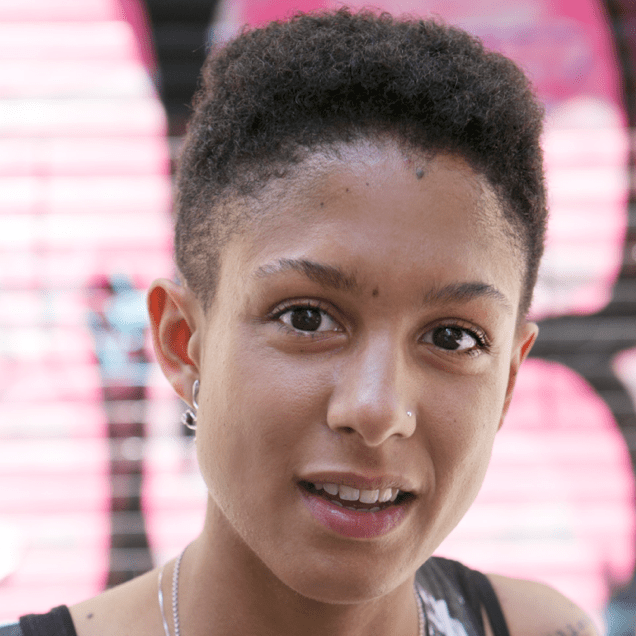
This was very spontaneous after I decided to study fundamental physics at university. During my studies I really enjoyed theory and math but also experimental aspects. Then I guess with time, I realized that I had more in common with the experimentalists than with theorists. In particular, for the instrumentation exam I had to present the functioning of an experiment of my choice and I picked up the most challenging and ambitious “on the market” at that time out of curiosity — it was the CMS experiment. It’s so complex that it’s like many experiments in one. If you understand that, then you understand everything else.
Where are the instruments physically located?
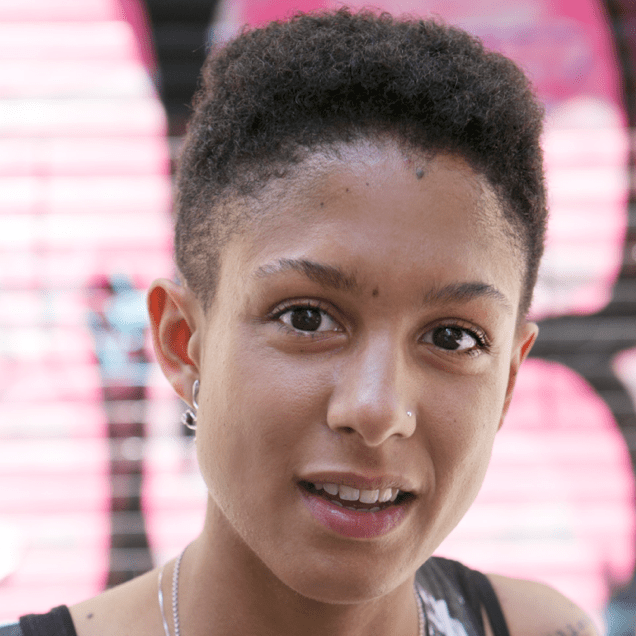
During testing and assembly of parts of the detector, a large fraction are located off-site. Right now when we are taking data, the experiment is fully assembled and running at its site on the LHC ring in a cavern that is ~100 meters underground. The electronics I am currently programming for real-time AI are also at that site. However, we also have spare boards or test stands replicating the CMS system in several places (also at Fermilab) and we can use such test stands for prototyping firmware without disrupting the normal CMS operations.
You said you are working on a new system now, while it is in design. Are you simulating it?
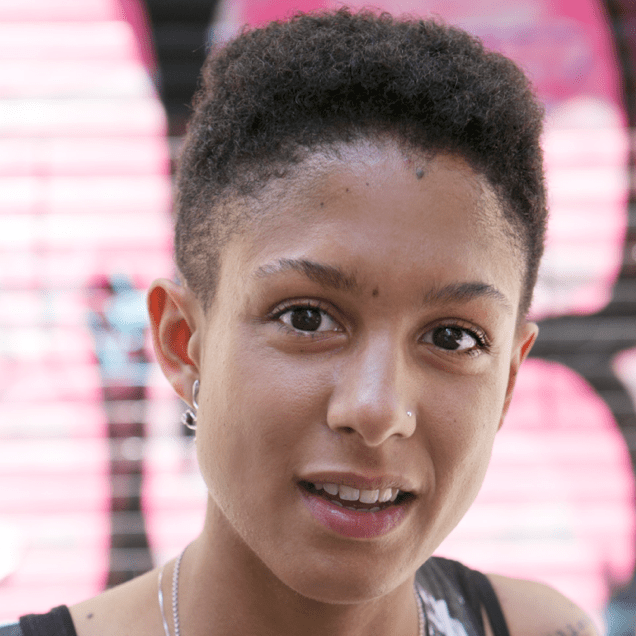
Yes, exactly. We are simulating it and we can use one of the test stands I mentioned above to test on dummy data. At least to check that everything is as the simulation tells us. However, the final demonstration is when the new algorithm is tested in the detector. In proton-proton [elementary particle] collisions, there are many nuisances not present in dummy data that could affect the performance and crash the data [collection]. That’s why even if we are now testing collisions, the data from the detector goes to a replica that allows us to monitor the behavior. [In other words, the new AI-integrated firmware (software embedded in hardware) is not in “production” yet but in “testing” mode].
How much data does the instrument produce a second, and how much is stored?
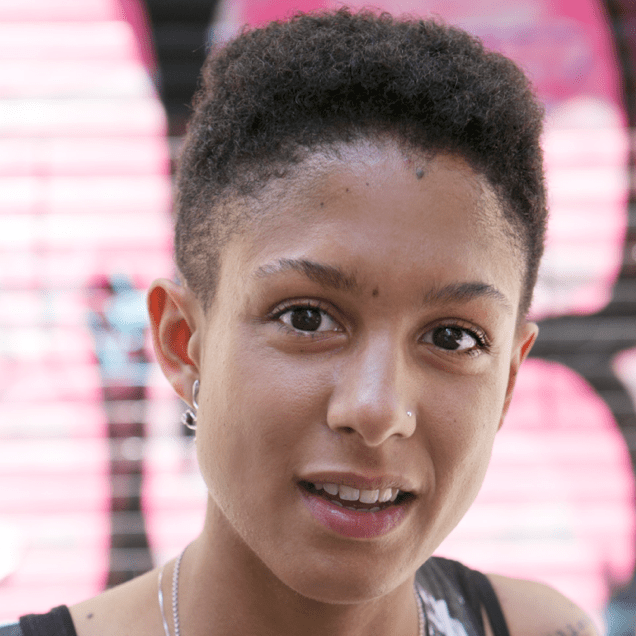
The detector produces ~1 petabyte per second, but we cannot process such rate downstream. The reconstruction of raw-level information from ~1 billion sensors to high-level information (e.g., electron, photon) is compute intensive, so at that rate it is impossible to accomplish without massive computational resources. The real time analysis system brings down the rate to ~10 gigabytes per second.