Bryan Wilder is an Assistant Professor in the Machine Learning Department at Carnegie Mellon University. His AI2050 project is developing machine learning approaches for making scientific predictions with heterogeneous datasets—such as combining datasets that have a lot of information about relatively few individuals, and a little information about many individuals. Wilder is working with healthcare data, which includes broad population-level data from many people, as well as detailed data from encounters with physicians and other healthcare providers. This is in furtherance of AI2050 Hard Problem #4, realizing the beneficial promise of artificial intelligence, especially with respect to scientific discovery, although it is also relevant to Hard Problem #2, which seeks to assure that AI systems operate reliably.
Wilder also teaches the CMU Course Machine Learning in Practice, “a project-based course designed to provide students training and experience in solving real-world problems using machine learning, exploring the interface between research and practice.”
Learn more about Bryan Wilder:
Your work focuses on AI for social impact. How are AI systems built for high-stakes social settings different from other AI systems?
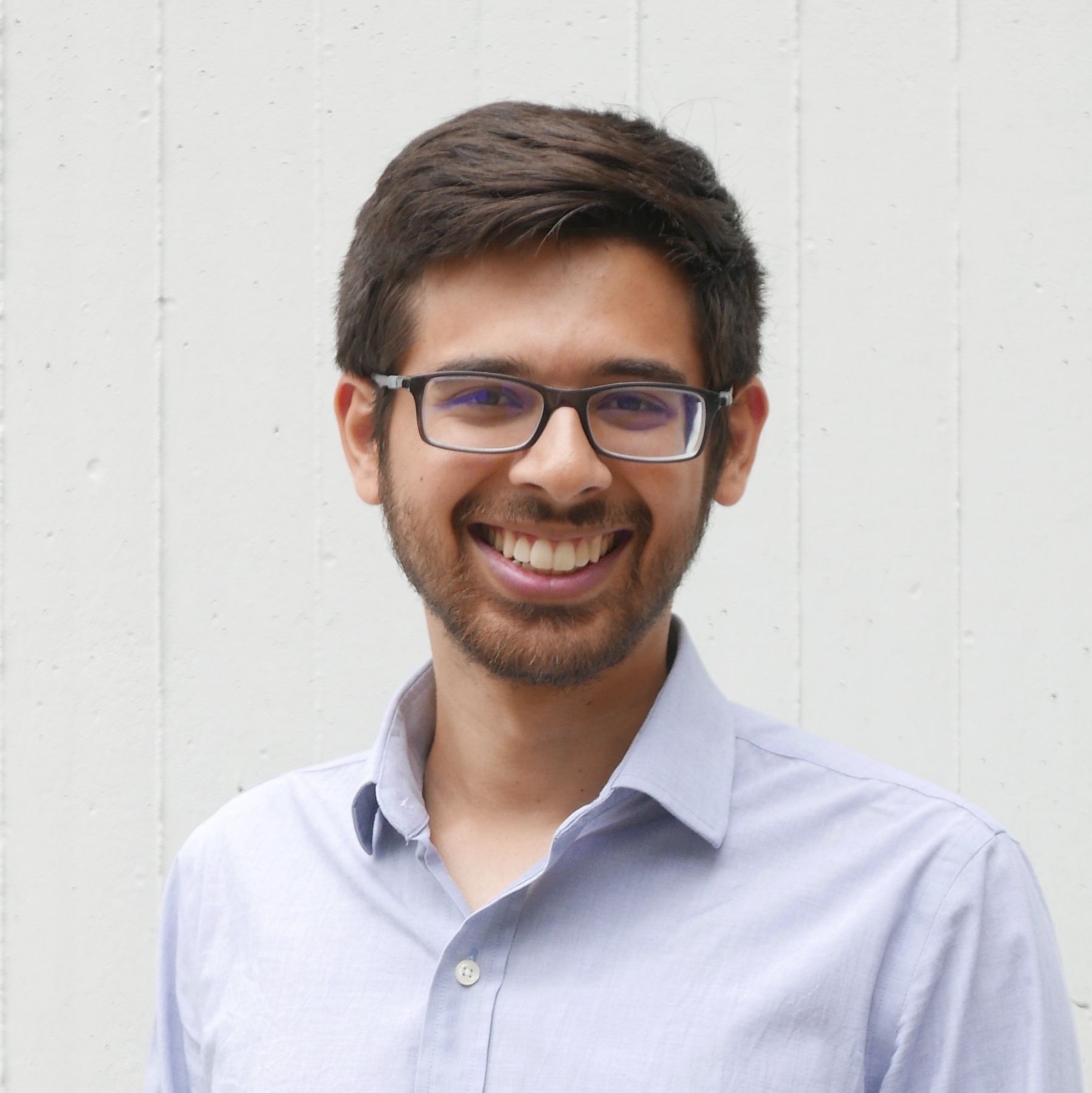
Ultimately, the difference is that AI for Social Impact requires a lot more than just AI; researchers must be able to integrate expertise not only in machine learning but also in statistics, policy, the social sciences, and application domains such as health or social work.
Two characteristics in particular stand out to me (dealing respectively with the “high-stakes” and “social” parts). First, in high-stakes settings, there is much more need for methods to be on a sound conceptual and empirical footing, because we need to understand when the system will work and when it won’t. Questions of robustness, validity, whether we can measure outcomes accurately, and so on, become essential. These cannot be addressed purely in the abstract and require substantial domain knowledge. Second, AI must be situated in a social context. Who are the stakeholders that should define the project’s goals? How will AI fit into a larger process involving human decision makers? How will people react to interventions informed by the system? Answers to these questions will often have a bigger impact than any technical choice.
How do you work with community organizations, especially those that don’t have their own machine learning experts?
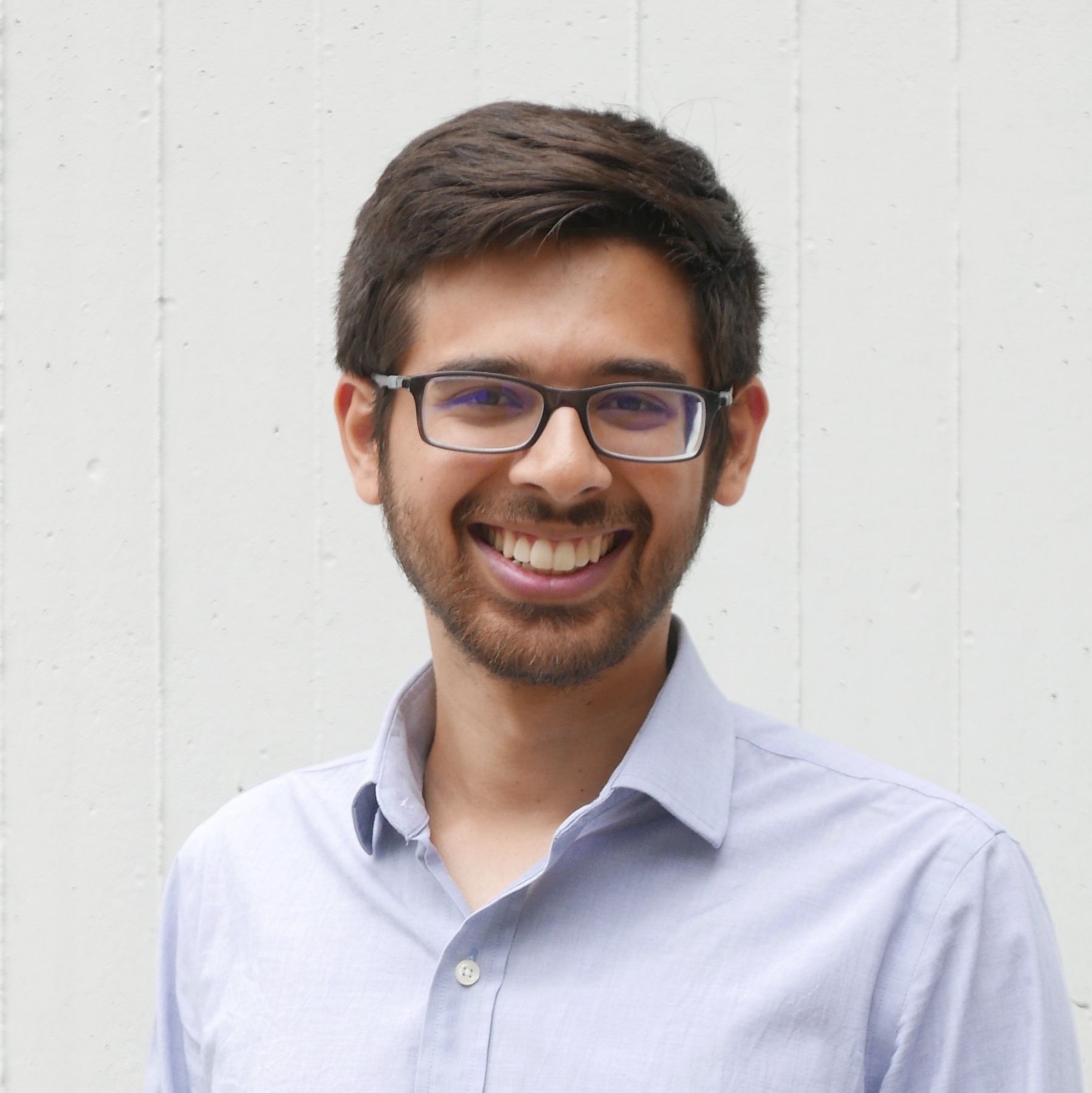
The starting point is to build a shared language on both sides.
As an AI researcher, the first priority is to understand where the real bottlenecks are in a community organization’s work: there’s no point spending a lot of time building an elaborate ML system that will only address a second-order concern. From the side of the community organization, the starting point is often to establish a clear picture of what AI might, in principle, be able to do. For example, it helps to provide concrete examples of AI’s use in policy or nonprofit settings. Hopefully, there’s an intersection in the form of a real, impactful problem where ML might provide a substantial improvement over the next-best option.
Much of the data that you work with is personal and highly sensitive. How do you protect the privacy of the people in your datasets?
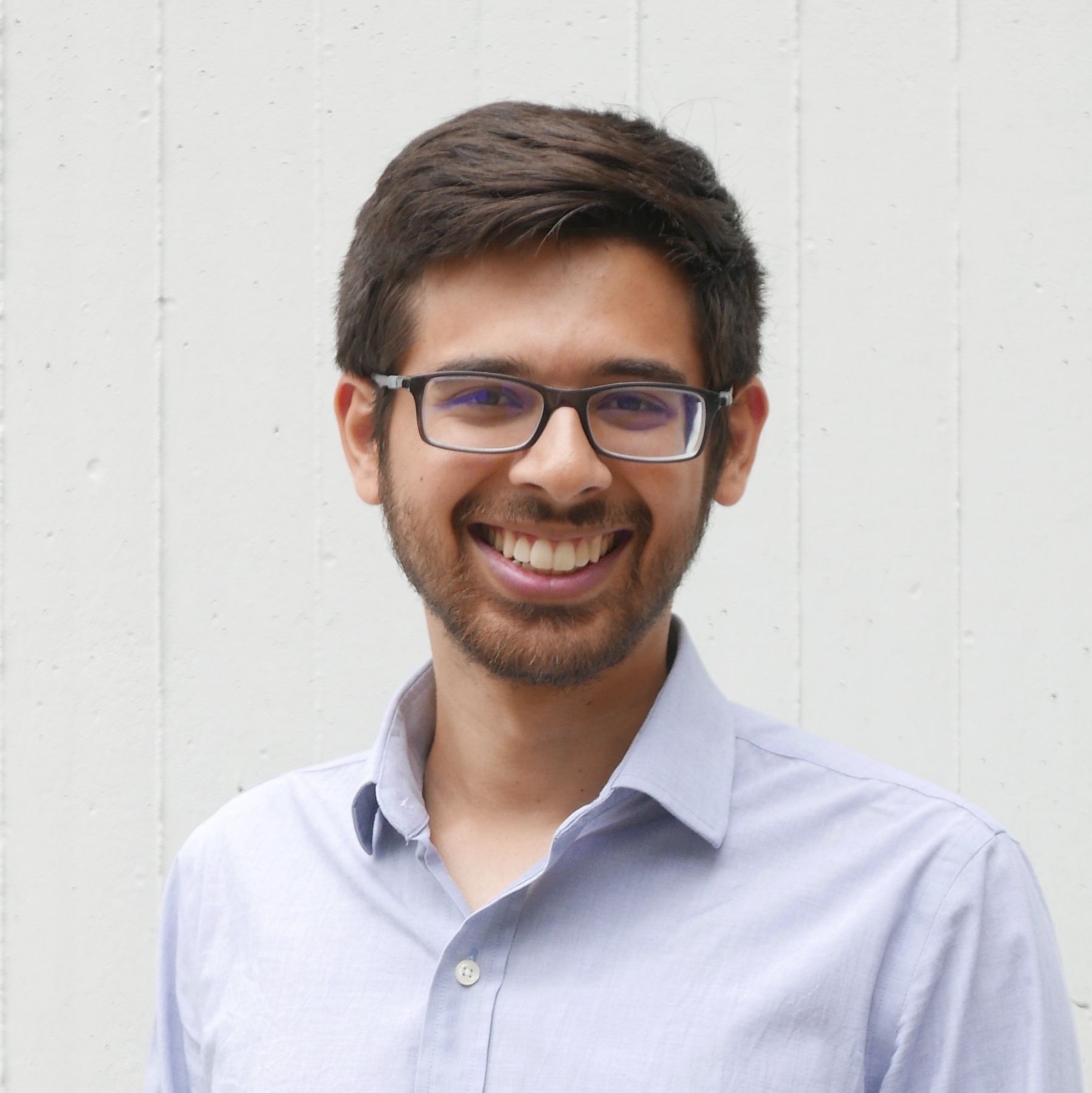
Data privacy is quite important for these projects — the way that I see it, people whose data we use are making a great contribution to a common good, and we’re obligated to ensure that they’re not harmed as a result.
There are a couple of aspects to this. First, we’re very careful about how datasets are stored and accessed. For example, it’s increasingly common for health datasets to be hosted in virtual enclaves by the data custodian (for example a hospital or insurer) so that it never actually leaves their systems. Second, we ensure that any publicly communicated results do not identify particular individuals. Typically, this is unlikely when predictions are released at a sufficiently aggregated level (e.g., at a high enough geographic level), but the considerations can vary from project to project.
In some of your talks, you say that you collect data from “social networks.” What does that mean, and how do you do it?
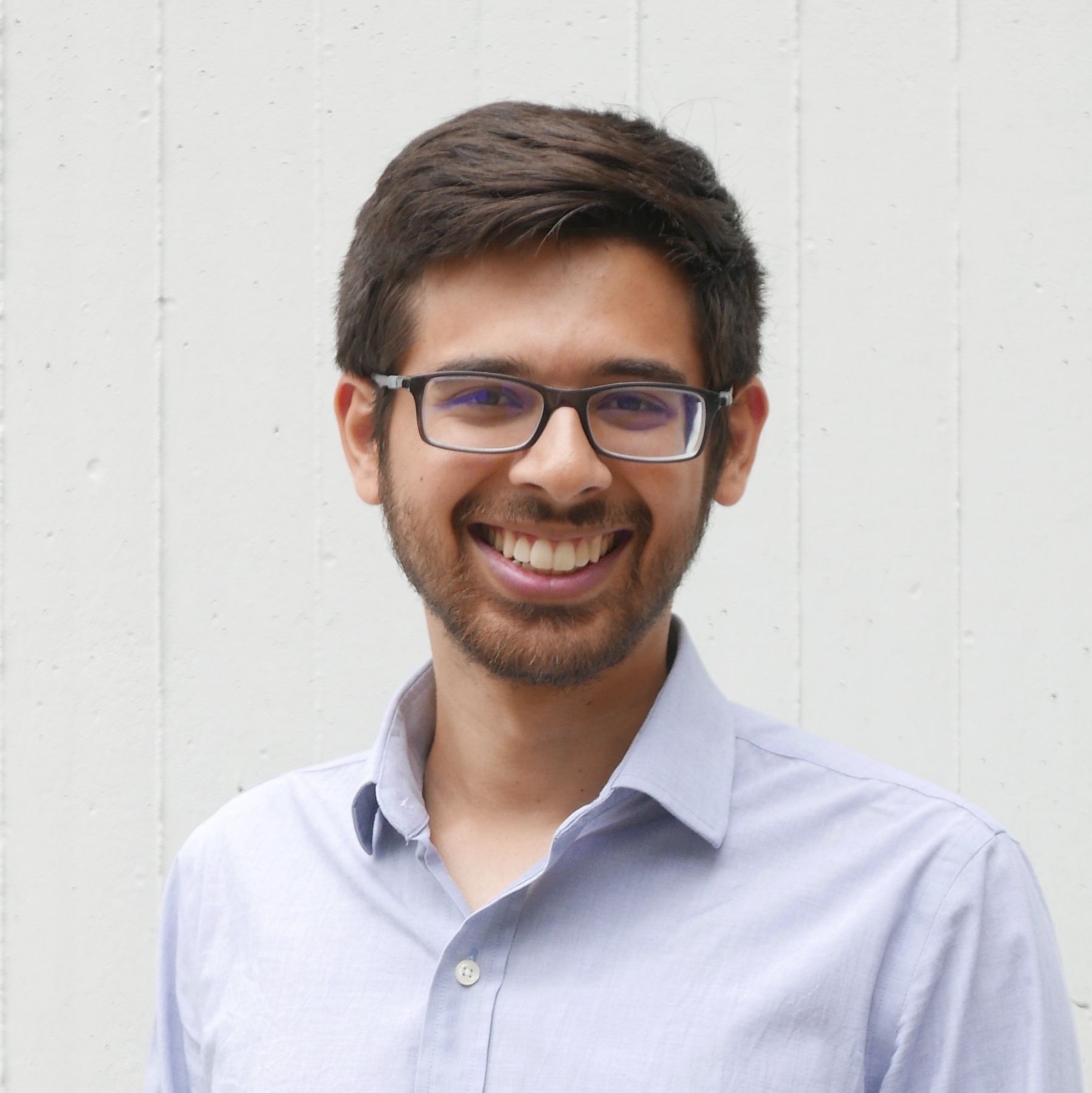
Social networks are fascinating because they’re so context-dependent and hard to measure. My past work has focused on social influences on health behaviors, in particular HIV prevention. Here, the “network” is the set of actual relationships between people who talk about health with one another. The form of those communications may be different in different settings or populations.
For example, my own work focused on youth experiencing homelessness, where much of the communication is face-to-face interactions rather than on digital platforms. Collecting data requires in-person surveys where we ask people to list those that they interact with regularly. This is quite time-intensive, and so part of our research focused on how to use small sub-samples of such data to target preventative interventions.
What is a deployment that you are currently working on?
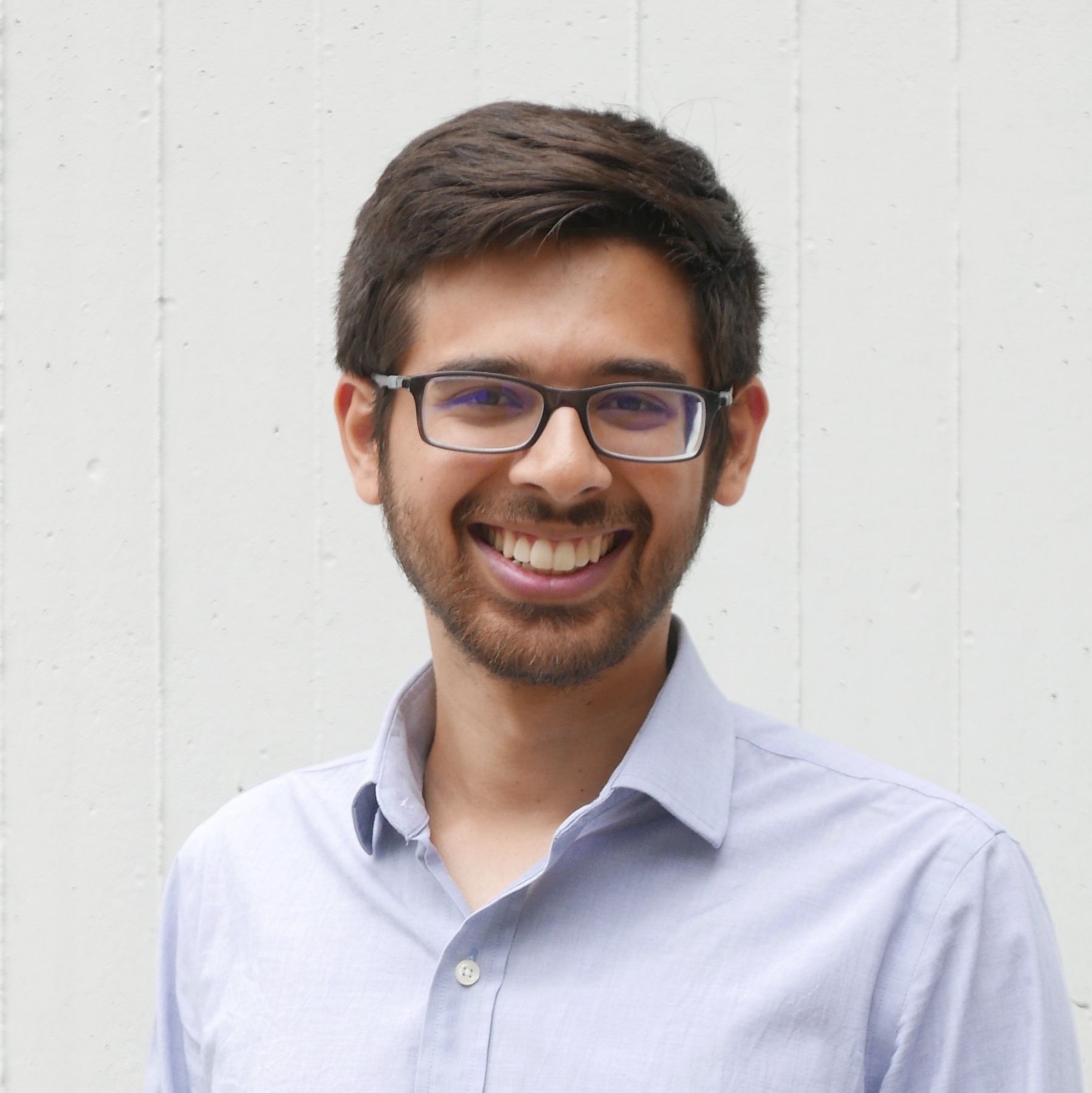
One deployment I’m working on is focused on improved tracking of infectious diseases. As the pandemic made clear, it’s incredibly difficult for policymakers and public health officials to get timely, accurate information that would allow them to guide decisions. There’s enormous potential in leveraging the kind of data that already exists in the health system — insurance claims, electronic medical records, and so on — to monitor population health in real time. However, this data comes with potentially severe biases, ranging from differences between health systems to poor coverage of people who have less access to healthcare.
I’m working on machine learning and statistical methods to learn robustly from this data, with initial deployments focused on tracking respiratory infections in the US: both the aggregate burden of disease (e.g., load on hospitals), as well as finer-grained descriptions of inequities in health outcomes and their potential mechanisms.
How can you use machine learning to prevent the spread of HIV?
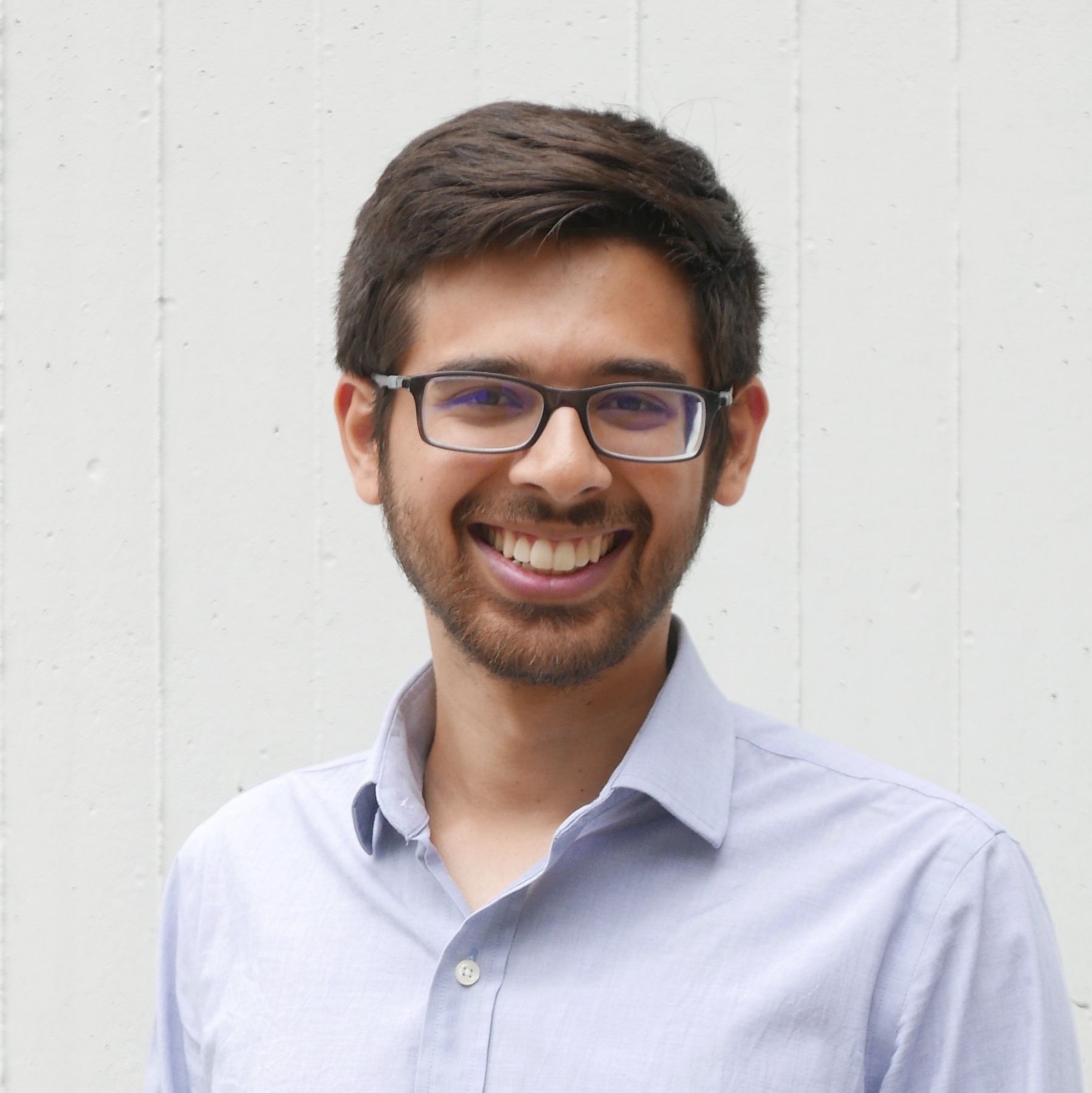
My own work in this space focused on targeting behavioral interventions. Social workers conduct interventions where they recruit people from within a given community to serve as peer leaders and advocate for preventative behaviors. The question is how to select the peer leaders who will create the greatest overall behavior change via the diffusion of social influence in the population. Our team of computer scientists and social workers developed algorithmic techniques to sample that underlying social network (like I mentioned above), and to optimize the selection of a limited [group] of peer leaders based on those samples. We conducted a field trial at three community organizations in Los Angeles that serve youth experiencing homelessness, and found that interventions planned in this way significantly increased adoption of preventative behaviors for this vulnerable population.
How many lives have you saved so far?
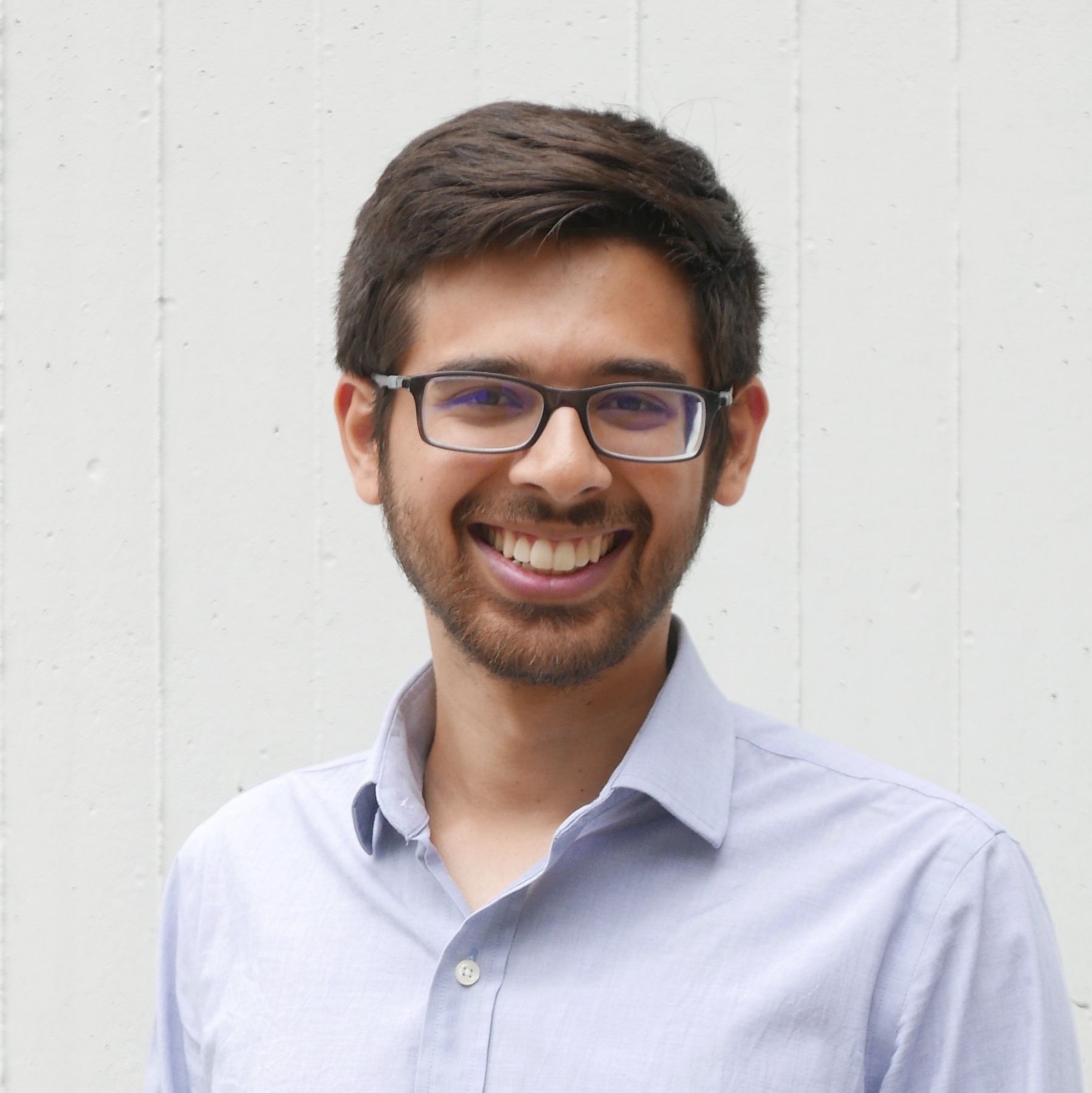
The honest answer is that there’s no way to know – there are many steps to get from, for example, increased adoption of preventative behaviors against HIV spread to lives saved. This is especially the case in the policy process, where an individual researcher’s contribution is only one small part of a bigger picture. For example, I think that some of our COVID modeling work helped along the way to availability of rapid testing, but I certainly can’t claim any individual credit for those policy changes. It’s a very humbling question to think about though. We really have an obligation to get this right.