Connor W. Coley has a shared appointment in the MIT Department of Chemical Engineering and the Department of Electrical Engineering and Computer Science. His AI2050 project aims to develop systems that can improve the ability of chemists to design and form new molecules with desirable properties, such as therapeutic drugs and pesticides. This work addresses Hard Problem 4 (having AI address one or more of humanity’s greatest challenges and opportunities, including in health and life sciences).
Today, says Connor, it can take chemists and chemical engineers more than a decade to take an idea for a new molecule from the lab into production. Molecules are collections of atoms connected together in a specific arrangement. Those connections determine its properties — how the molecule will function if used as a drug, insecticide, lubricant, adhesive, or anything else. The only way to know for sure what kind of properties a molecule will possess is to make it in the lab and test it, experimentally.
Connor is trying to short-circuit the process, using AI to design new molecules, and identify those which could be used in a chemical reaction to form new products.
Connor says he hopes that by 2050 we will be able to accurately predict the properties of a molecule from its structure, making it possible to design and then synthesize new chemicals for all kinds of applications in construction, healthcare, manufacturing, and pretty much any human activity that involves the creation of physical objects or the rearrangement of matter. A large part of the solution will be new techniques based on artificial intelligence. These techniques will decrease the time and cost required to discover a new drug ready for clinical trials, for example.
Learn more about Connor W. Coley:
We don’t hear much about using AI for chemistry. Why is that?
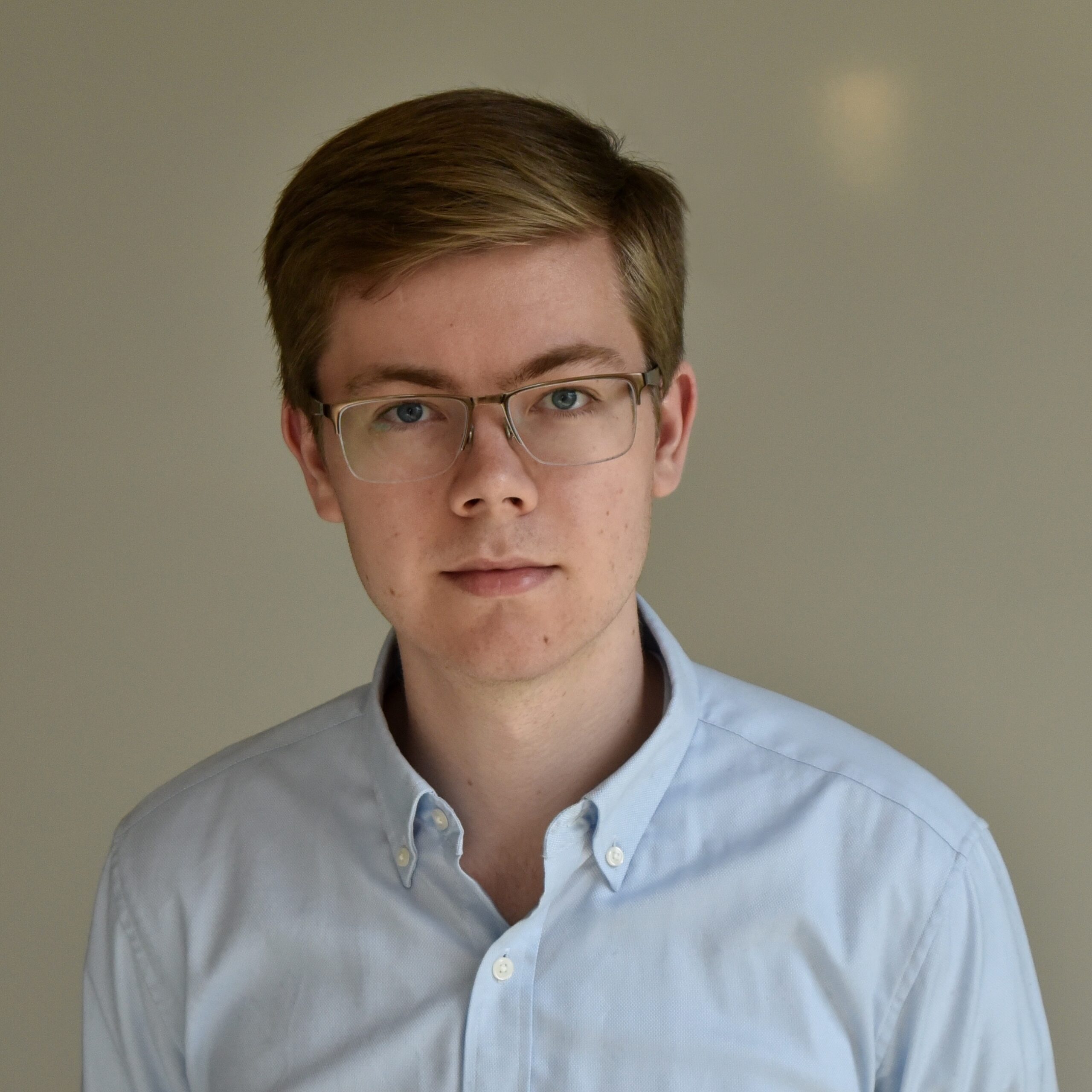
I think there are at least a couple reasons. Most chemistry research is done ‘behind the scenes’ by domain experts, so we don’t have the same opportunities to communicate advances to the broader community [via widely shared computer programs] the same way that we do for image generation (e.g., DALL-E), text generation (e.g. ChatGPT), or gameplay (e.g. AlphaGo). A second reason is that the types of problems AI can solve in chemistry are more narrow and typically rely on datasets that are specific to an individual researcher’s goals. Also, datasets in chemistry tend to be more tightly controlled or commercial, particularly when they contain information that might be pertinent to drug discovery.
Still, there are examples of AI for chemistry dating back to the 1960s if you know where to look!
In your vision, will humans be performing the experiments that the AI systems recommend, or do you envision that the entire lab will be automated?
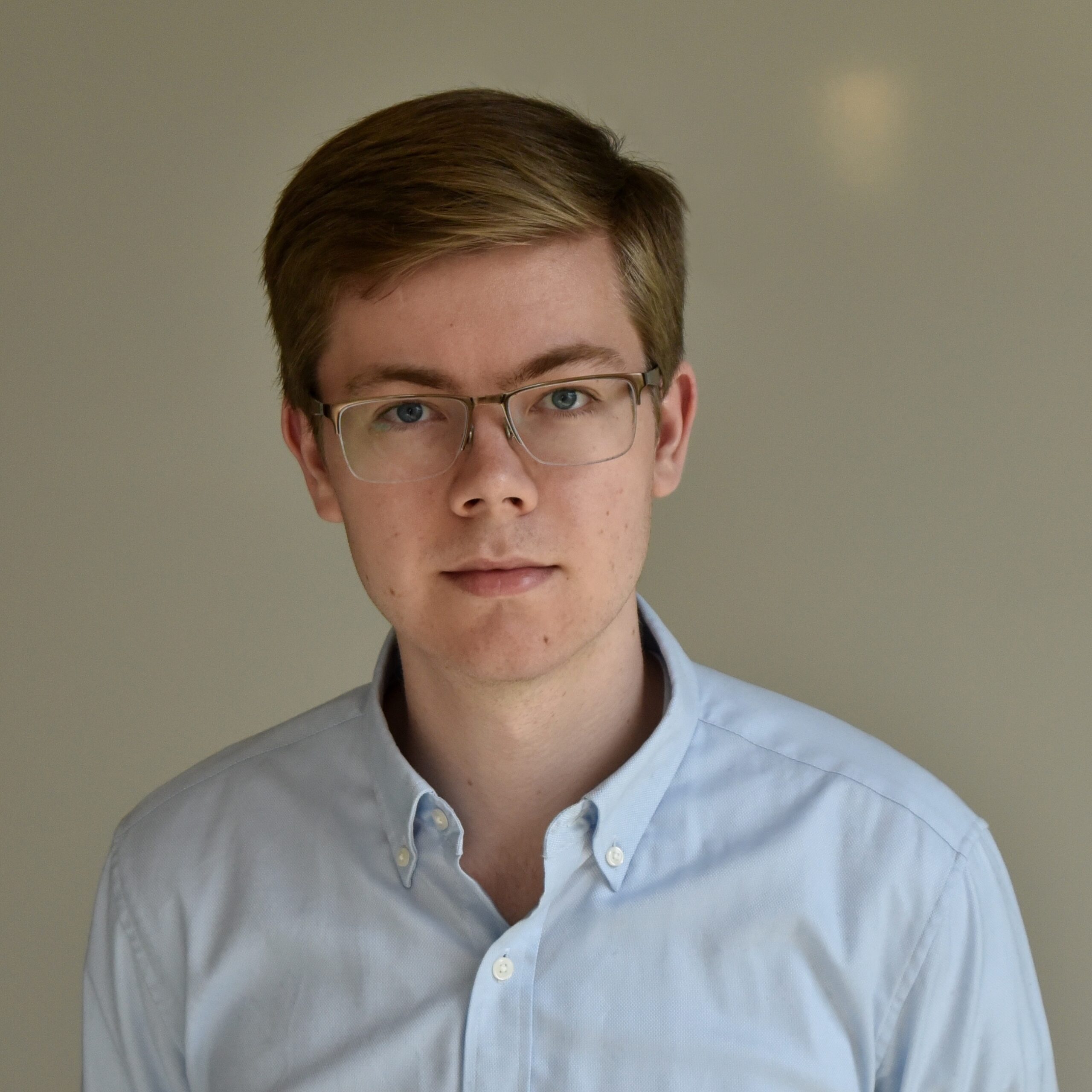
Both! There are still many physical operations we do in the lab that are hard to completely replace with robotics. Robotic labs don’t have the same adaptability in responding to unexpected situations, sometimes for seemingly-mundane things like [knowing] whether a powder it is measuring is sticky or clumpy. Our AI tools might not fully understand what physical operations are easy or hard to do; similarly, they do not fully understand what molecules are easy or hard to make. At the same time, automated laboratories excel at low-volume liquid handling and can often operate at much higher throughputs [rates of production] than manual experiments.
Today we can’t predict chemical properties using quantum mechanics because the math gets too complex. However, many people think that a special kind of quantum computer called a “quantum simulator” might be able to do this before the end of the decade. Do you think that AI is just a transitory technology, or do you see AI and quantum computers working together?
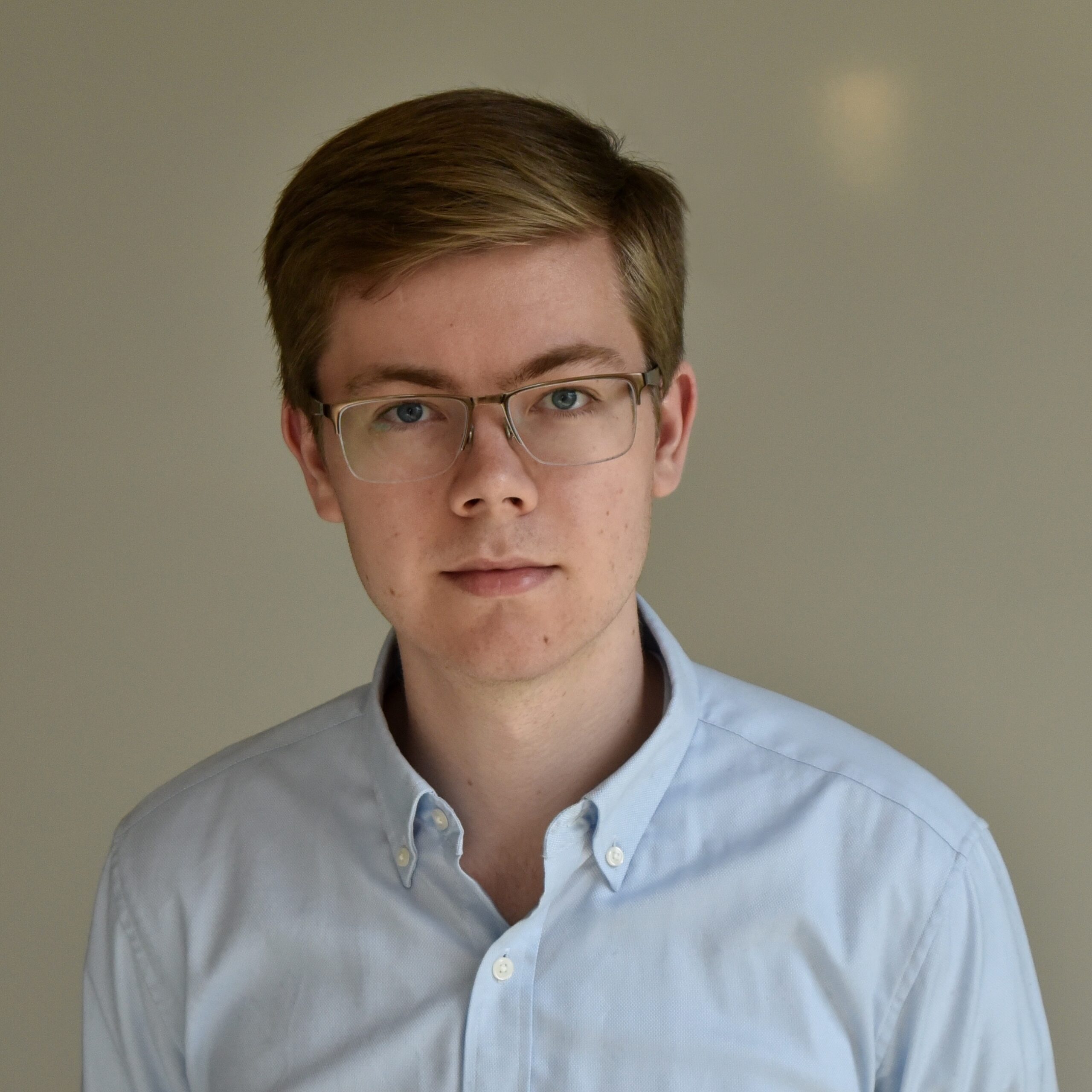
I don’t see it that way. The first thing to note is that AI can help us generate ideas and hypotheses, which is a use case beyond the prediction of properties. Even for property prediction, there will (likely) always be phenomena that are impossible or impractical to simulate with full accuracy. For example, a major challenge in drug discovery is the complexity of human physiology. The disconnect between what we’re able to understand about molecular behavior and function–even with [the help of] animal models–means that the majority of drug candidates fail in clinical trials. Even if quantum computers and quantum simulators are able to probe complex intermolecular interactions from first principles, the multi-scale nature of the problem means that this disconnect will persist. AI should still continue to be useful as a bridge between our first principles understanding and experimental observations.
What’s your advice for students who want to combine AI and chemistry?
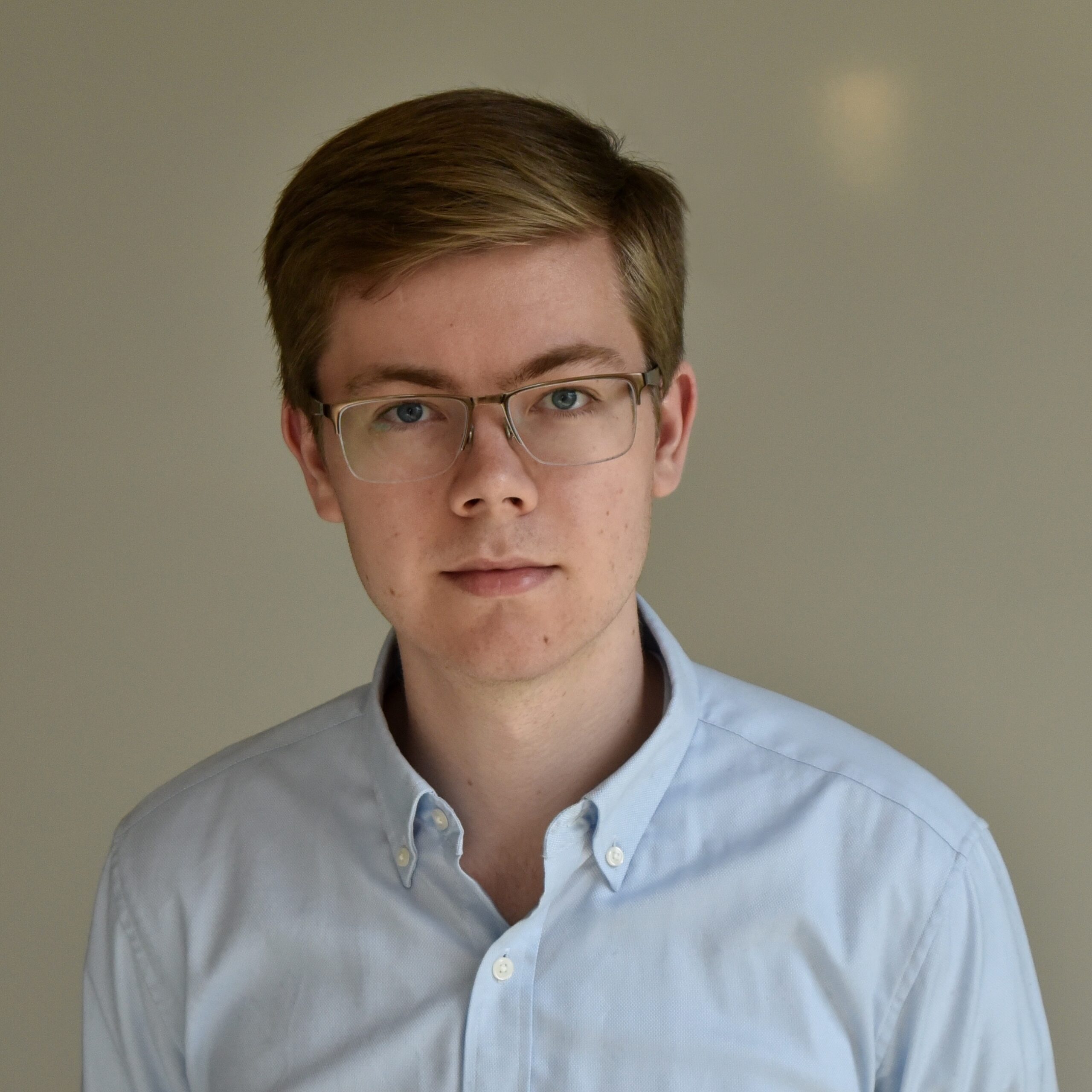
It’s never too early or late to start. There are terrific resources out there for learning AI fundamentals and how different algorithms work. Part of what makes the combination of AI and the physical sciences special is that there is a “virtuous cycle” between foundational and applied research, to borrow some language from the National Science Foundation. Students who are able to become fluent in AI methods and also use their domain expertise to understand what the highest value applications are will find themselves with a very unique and sought-after perspective. That’s what we need in order to use AI to address humanity’s greatest challenges and opportunities—one of the AI2050 hard problems that describes our goals.