Many of the recent breakthroughs in AI have resulted from dramatic increases in computing and data available to address hard technical problems. Most prevalent are large synthetic “neural networks” like ChatGPT, that take inspiration from the architecture of the human brain – which consists of billions of neurons – to connect together billions of mathematical equations to produce a single result. Built with neither elegance nor finesse, these artificial neural networks rely on vast warehouses filled with computers and gigawatts of electricity to achieve their aims.
An alternative approach for constructing neural networks – called“Liquid Neural Networks” – is being developed by AI2050 Senior Fellow Daniela Rus. Instead of relying on millions, or even billions of mathematically modeled “neurons,” the liquid approach relies on just a handful— resulting in substantial efficiency improvements. This is done by applying sophisticated mathematical modeling to the way that the neurons connect to each other, their training, and how they model progress of time. Daniela’s work addresses Hard Problems #1 (develop more capable and more general AI, that is safe and earns public trust), #2 (AI safety and security), and #4 (having AI address one or more of humanity’s greatest challenges and opportunities, including in health and life sciences).
The “liquid” approach for building highly efficient machine learning systems is the result of a collaboration spanning several years and two continents. The approach was first put forth in Ramin Hasani’s 2020 PhD thesis at TU Wien in Austria; his advisors were Professor Radu Grosu (TU Wien), Daniela Rus (MIT), and Dieter Haerle at the Austrian research center KAI. Hasani then joined MIT’s Computer Science and Artificial Intelligence Lab (CSAIL) as a postdoctoral associate, where he continued to work on the liquid networks concept with AI2050 Senior Fellow Daniela Rus. It was at MIT that the team developed a solution to a century-old mathematics problem, allowing the networks to become dramatically more compact and efficient.
The results are impressive and might have far-reaching conclusions. In the lab, a liquid network trained on a dataset of 8000 patients admitted to an intensive care unit could make mortality predictions with 87% accuracy after just 6 seconds of training; by comparison, the best non-liquid approach achieved only 85% accuracy and required more than 8 minutes to train.
In 2022, MIT was one of three groups to win the HPC (High Performance Computing) Innovation Excellence Award, identifying achievements with significant potential for benefiting humanity, for the invention of liquid machine learning systems. Last year, Daniela and the team recently announced Liquid AI, a startup building foundation models using liquid neural networks with ~$38m in seed funding.
Learn more about Daniela Rus:
What’s the motivation for Liquid Networks?
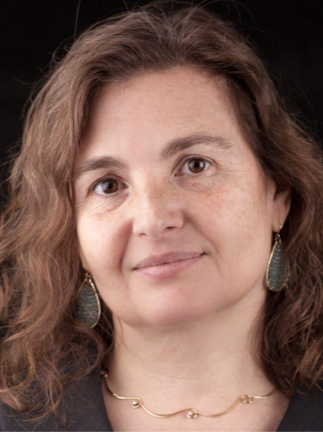
We began to develop this work as a way of addressing some of the technical challenges with today’s AI solutions. First among the AI challenges is the data itself — machine learning requires huge amounts of data for training immense models. The models have a huge computational and environmental cost. We also have an issue with data quality: if the data quality is not high, the model will not be good. Bad data means bad performance. Furthermore, we have black-box systems, where it is really impossible to find out how the system makes decisions. This is really problematic, especially for safety critical applications.
Some of the possibilities that you and your students have pioneered for liquid neural networks include controlling autonomous vehicles. In 2020, your students created a liquid neural network consisting of just 19 neurons that was able to steer a Lexus on a 1km long private test road with unlabelled lane markers “at a constant velocity of 30 km/h.” The liquid network performed better than a conventional “deep learning” neural network with over 100,000 artificial neurons. (These results and others were reported in the November 15, 2022 issue of Nature Machine Intelligence.) But many deep learning people say that all you need to do is add more neurons. Is there a significant advantage to liquid networks, other than their small size?
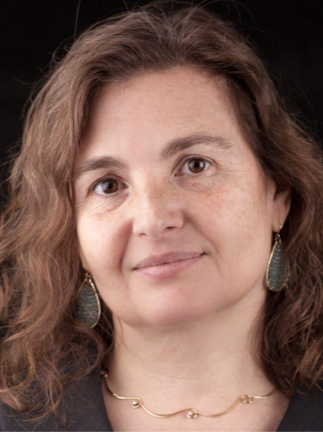
Liquid networks seem to understand their task better than deep networks. And because they are so compact, they have many other useful properties. In particular, we can take the output of the 19 neuron [autonomous vehicle] model and turn it into a decision tree which can show in a [human comprehensible] way how the networks make their decisions. This takes us closer to a world where we have machine learning that is auditable and certifiable.
We can also prove that our liquid networks are causal — that they connect cause and effect in ways that are consistent with the mathematical definitions of causality.
You have also used liquid networks to control a drone to fly to a target, like a person walking with a red backpack?
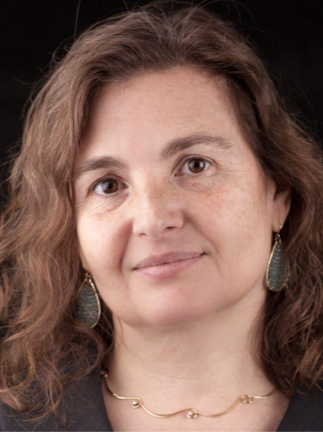
We are training the drone how to fly in the woods. We can then change the task entirely. We can put it in an urban environment and go from a static environment [a backpack hanging from a tree] to a dynamic object. It’s the same model trained in the woods! So this is again [possible] because we have a provably causal solution.
What’s the next step for liquid networks?
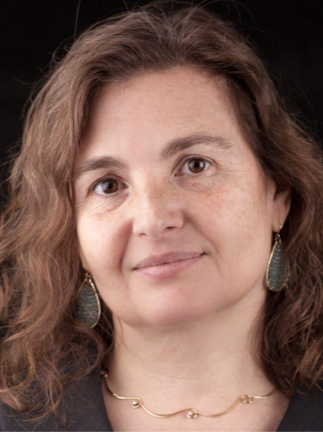
We have shown that Liquid Networks are causal and this opens the door to many possibilities. We are also working on the role of ‘attention’ in the function of liquid networks. [‘attention’ refers to where in an image that a network should focus for decision making] We are also working on learning in decentralized systems because complex tasks require the coordination of more than one agent.
Beyond the work on liquid networks, what else are you doing with your AI2050 fellowship?
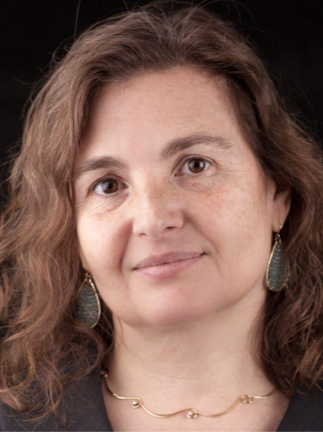
I am very interested in the certification and auditability of machine learning-based control for safety critical systems. This is a very important area for the broad deployment of machine learning. Understanding how such a system will behave is critical.