Community Perspective – Dylan Hadfield-Menell
Q&A with Dylan Hadfield-Menell, AI2050 Early Career Fellow
“The nature of what a human or group of humans values is fundamentally complex — it is unlikely, if not impossible, that we can provide a complete specification of value to an AI system,” says AI2050 Early Career Fellow Dylan Hadfield-Menell. “As a result, AI systems may cause harm or catastrophe by optimizing an incorrect objective.”
For example, consider an AI system that might be equipped with an off-switch so that humans could always remain in control. Perhaps this means that humans would always be able to switch off the AI. But an AI that is told to optimize its chance of performing a task would tend to disable its own off-switch over time because the switch, if accidentally flipped, would prevent the final goal from being achieved.
This idea, long hypothesized in the AI literature, was proven by Dylan Hadfield-Menell along with Anca Dragan, Pieter Abbeel, and Stuart Russell in 2017 when they published their paper “The Off-Switch Game” at the International Joint Conference on Artificial Intelligence. (Stuart Russell is an AI2050 Senior Fellow.) The only solution to the off-switch game is to convince the AI that the human is perfectly rational and will never flip the off-switch in error.
Since then, Hadfield-Menell has continued to work on the issue of AI alignment—how to align the goals of AI with goals of humans.
Hadfield-Menell’s AI2050 project uses reinforcement learning to experimentally model situations in which an AI system can manipulate humans, developing a theoretical approach for determining conditions that are sufficient for open AI systems to be safe and beneficial. Reinforcement learning is an AI approach in which the AI performs a task and then gets feedback as to whether the task was successful or not. Reinforcement learning was used by DeepMind to create AlphaGo, which became the world’s best Go player by playing itself and learning which moves worked and which moves did not. But reinforcement learning sometimes produces unexpected results, like AIs that learn to cheat at video games by exploiting their bugs.
Like his earlier work in The Off-Switch Game, Hadfield-Menell is most concerned with systems that evolve over time to adapt to new conditions and develop secondary goals—like an AI that cheats to achieve its primary goal.
Learn more about Dylan Hadfield-Menell
If we use reinforcement learning to produce systems that are “safe and beneficial,” doesn’t that mean that we need to have a mathematical definition for what it means to be “safe and beneficial?” How do you mathematically define safety?
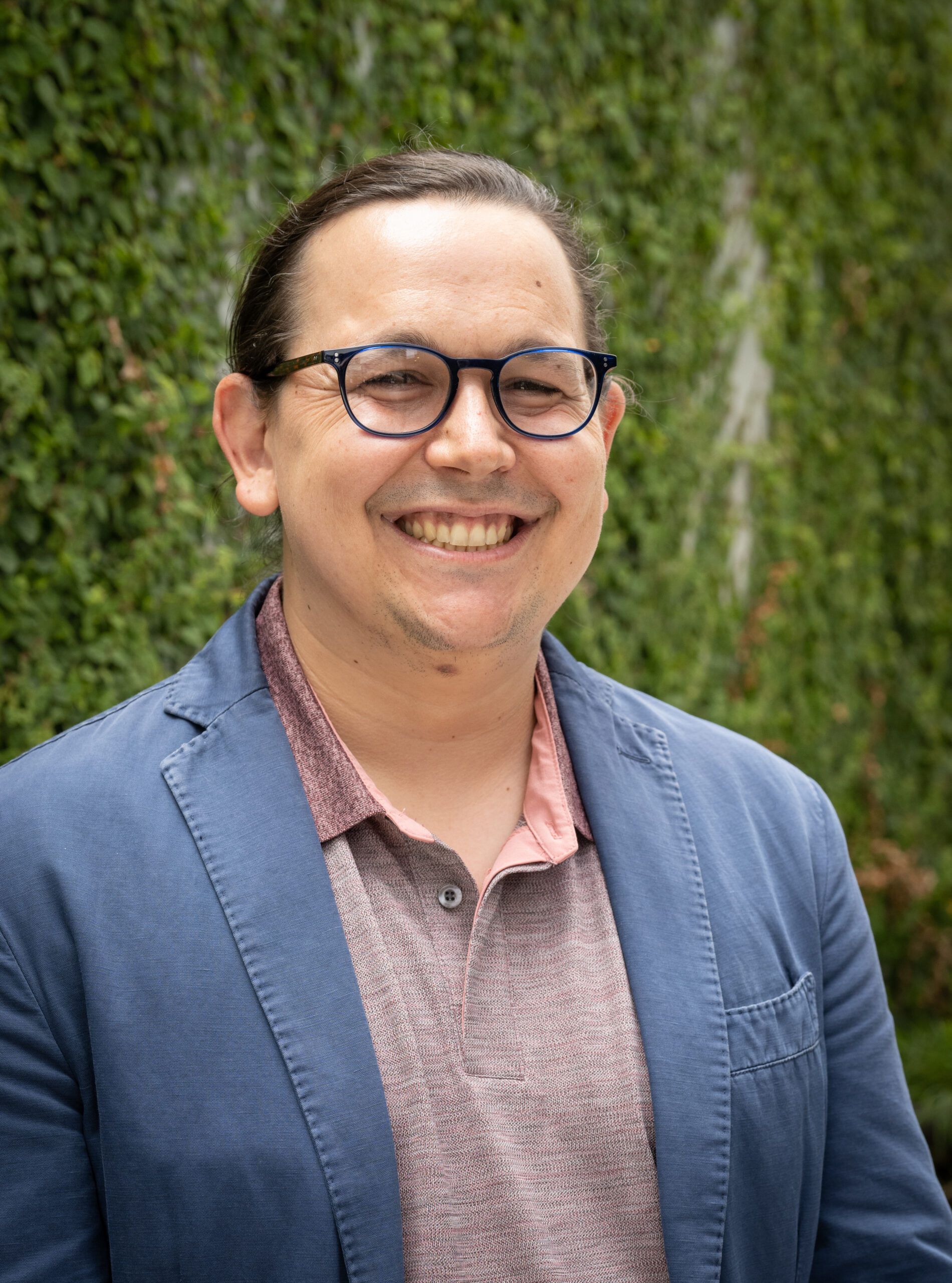
My research focuses on the design of incentives or goals for AI systems. I try to understand the ways that goals can be misspecified and the consequences of that misspecification. One of our central results is that incentive specification is often brittle. This means that a seemingly small change in the way a goal is measured can have large and counterintuitive effects on behavior.
A classic example of this is a robot vacuum that is given the goal of ‘sucking up as much dirt as possible’. While a seemingly reasonable goal, a robot agent maximizing this goal will, for example, dump dirt onto a clean floor immediately after sucking it up so that there is more to clean. In many cases, this is probably worse than no robot vacuum at all! My research usually defines safety with respect to this baseline of ‘no robot assistance’. At a minimum, we would like to ensure that the worst case of misspecification is the same as having no robot. You could loosely think of this as ‘do no harm’.
What about beneficence?
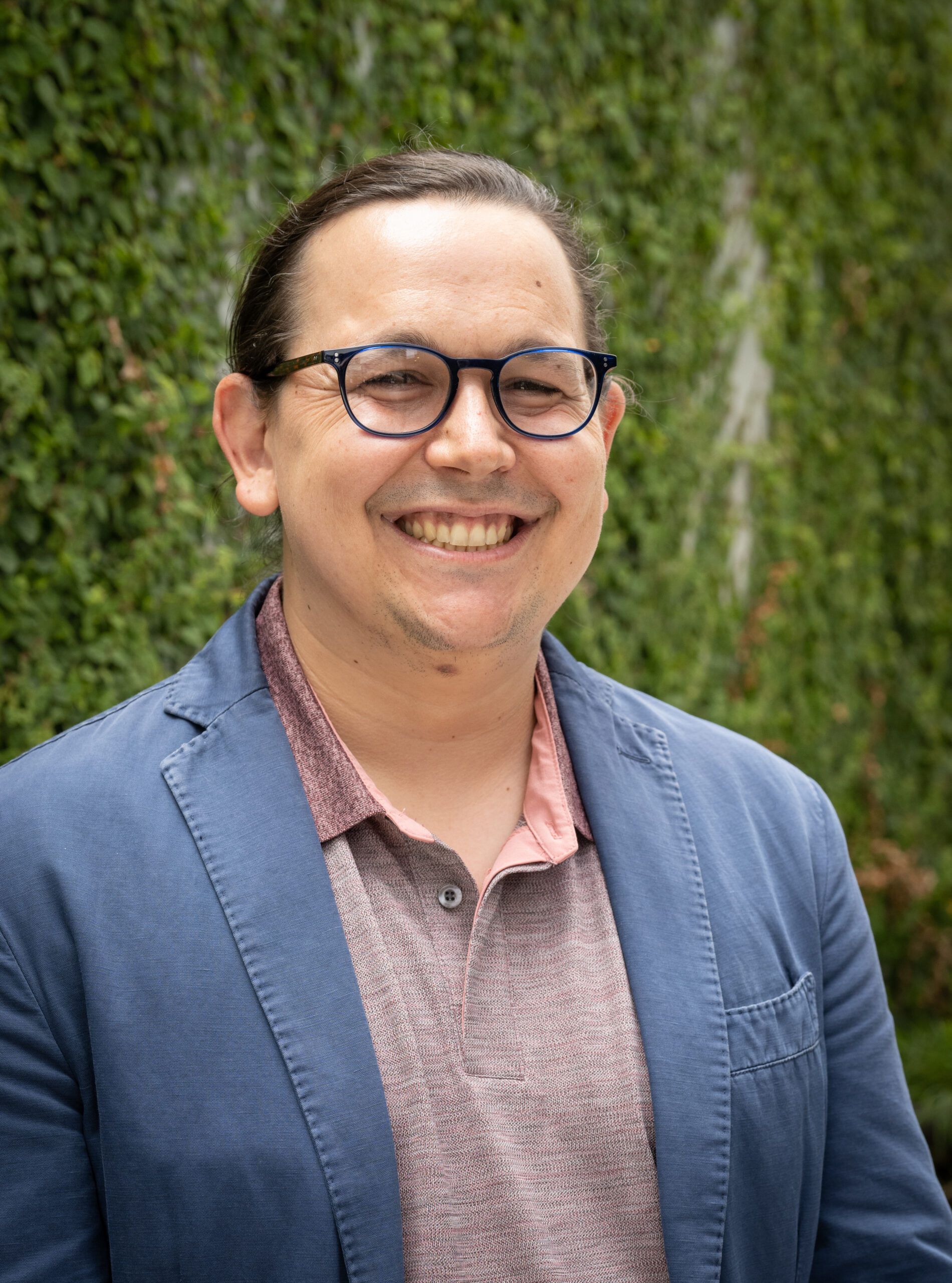
My research often studies the interaction between an AI system/robot and a human principal as an assistance game. In this [game], the human and the robot are on a team where the team’s goal is to maximize utility for the human. The key feature of assistance games as they relate to my research is that the robot has to learn about the human’s goal over time. In my research, beneficence refers to the ability of the robot to be a good teammate and provide value in this game. To put it in context of the previous answer about safety: a robot is ‘safe’ as long as it is not a bad teammate in an assistance game and it is ‘beneficial’ when it is a good teammate in an assistance game.
“Beneficence” is also one of the guiding principles of the Belmont Report (1979), which provides the ethical framework for research involving human subjects in the United States. Do you see echoes of the Belmont Report in your work, especially as you research AI systems that seek to manipulate humans?
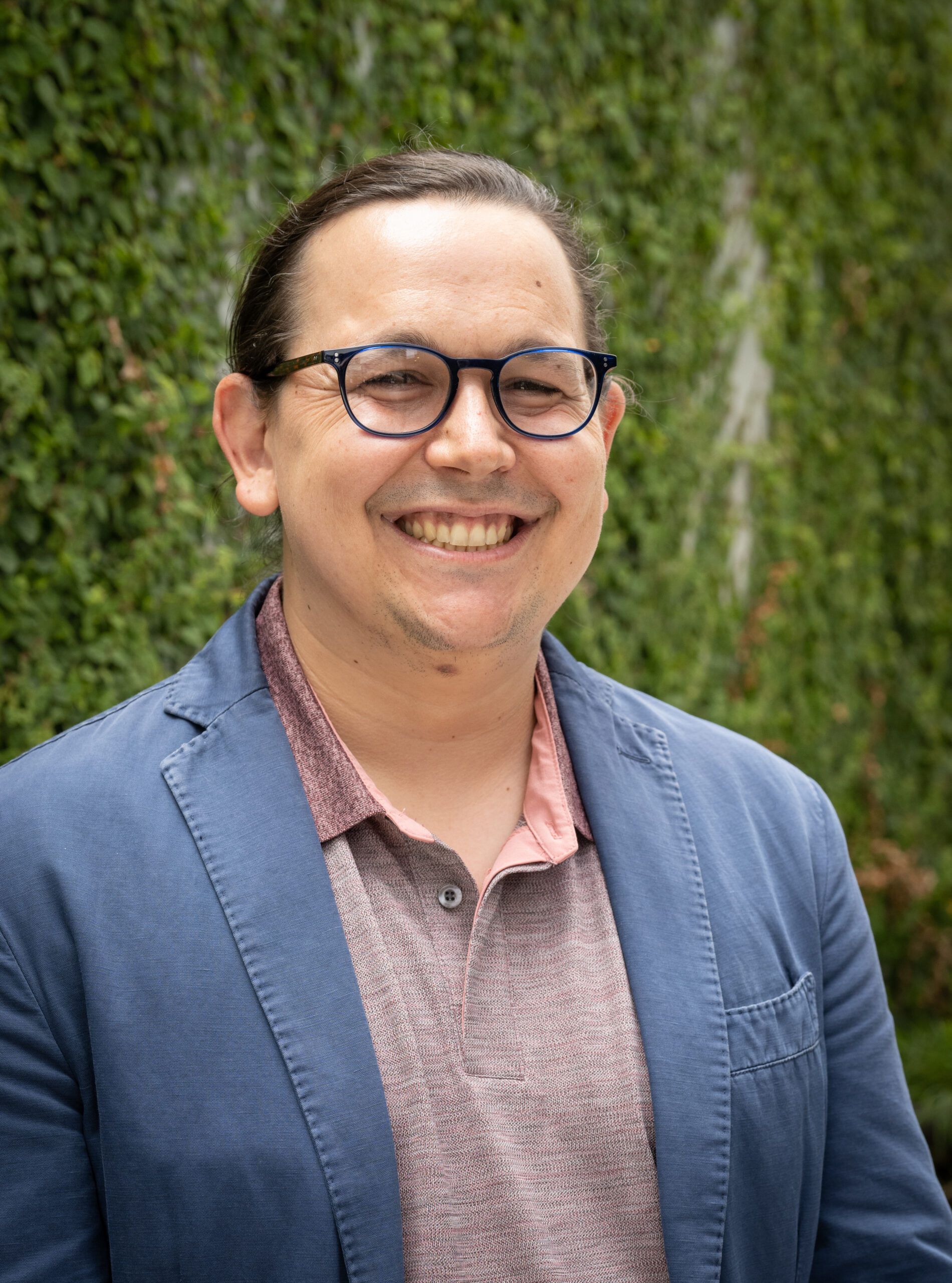
Yes, certainly. I think the Belmont Report was a central step towards the ethical conduct of research. There is a general similarity in that my research relates to the ethical conduct of AI research and development. With respect to the capacity of AI systems to manipulate people, the strongest similarity has to do with the notion of informed consent [of the humans that are the users of the AI systems]. By default, an AI system will readily leverage statistics to optimize its objective. This means that, unless manipulative behavior is penalized, we should expect systems to take actions that humans would classify as manipulative. I am motivated to work on this because of the challenge that these systems pose to our values of informed consent.
Just like an AI trained to play a video game might learn ways to cheat by exploiting bugs, an AI that is given the goal of producing an outcome in humans might discover ways of exerting hidden psychological pressure, manipulating people without their knowledge. Do you ever work with human subjects?
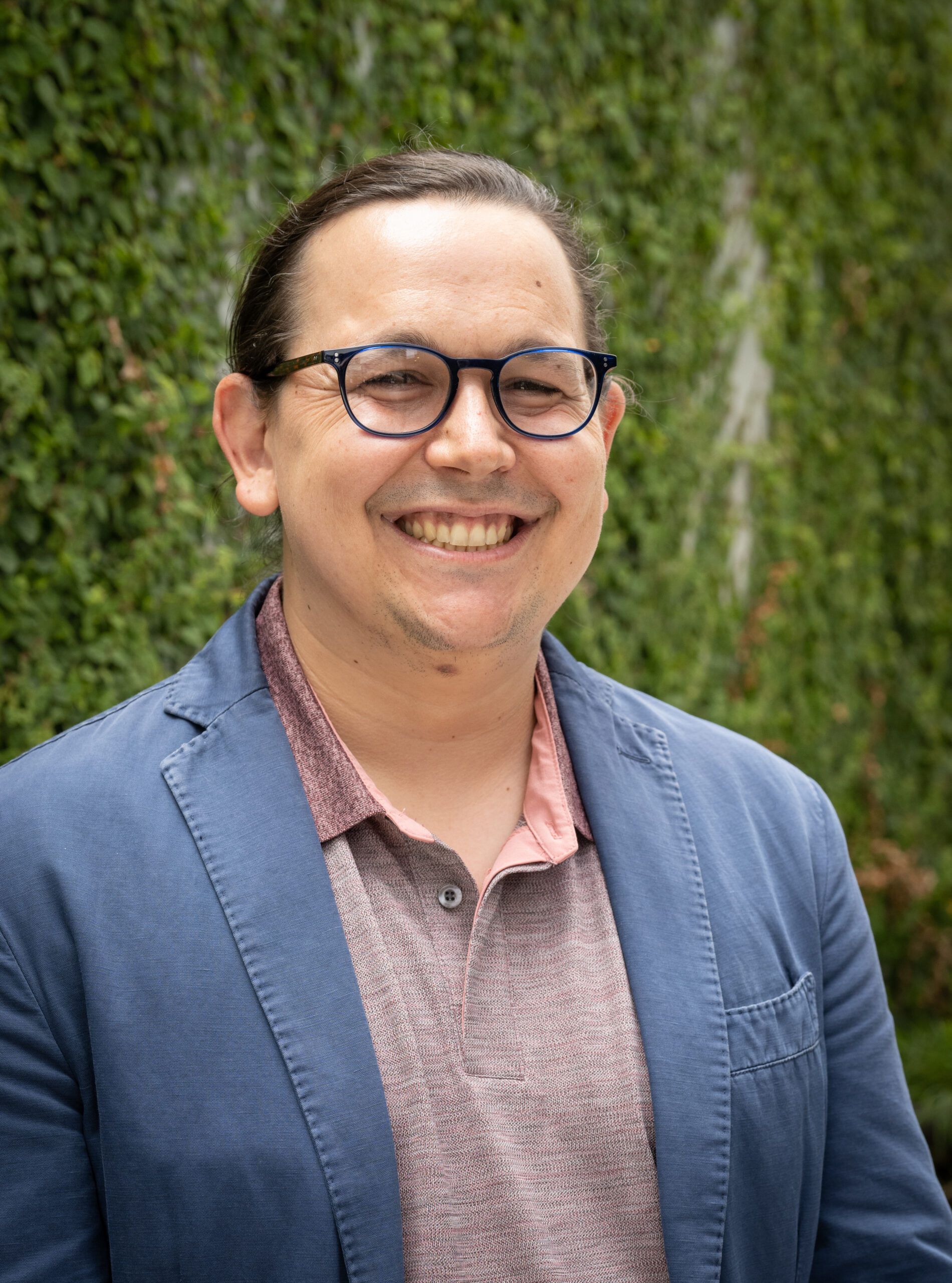
Yes. Usually, that has been in the context of human-robot interaction studies. I have a few studies in preparation where we are doing work with human subjects for recommendation systems.
Do you think that we might have a future in which you need some sort of regulatory approval to do certain kinds of research on AI?
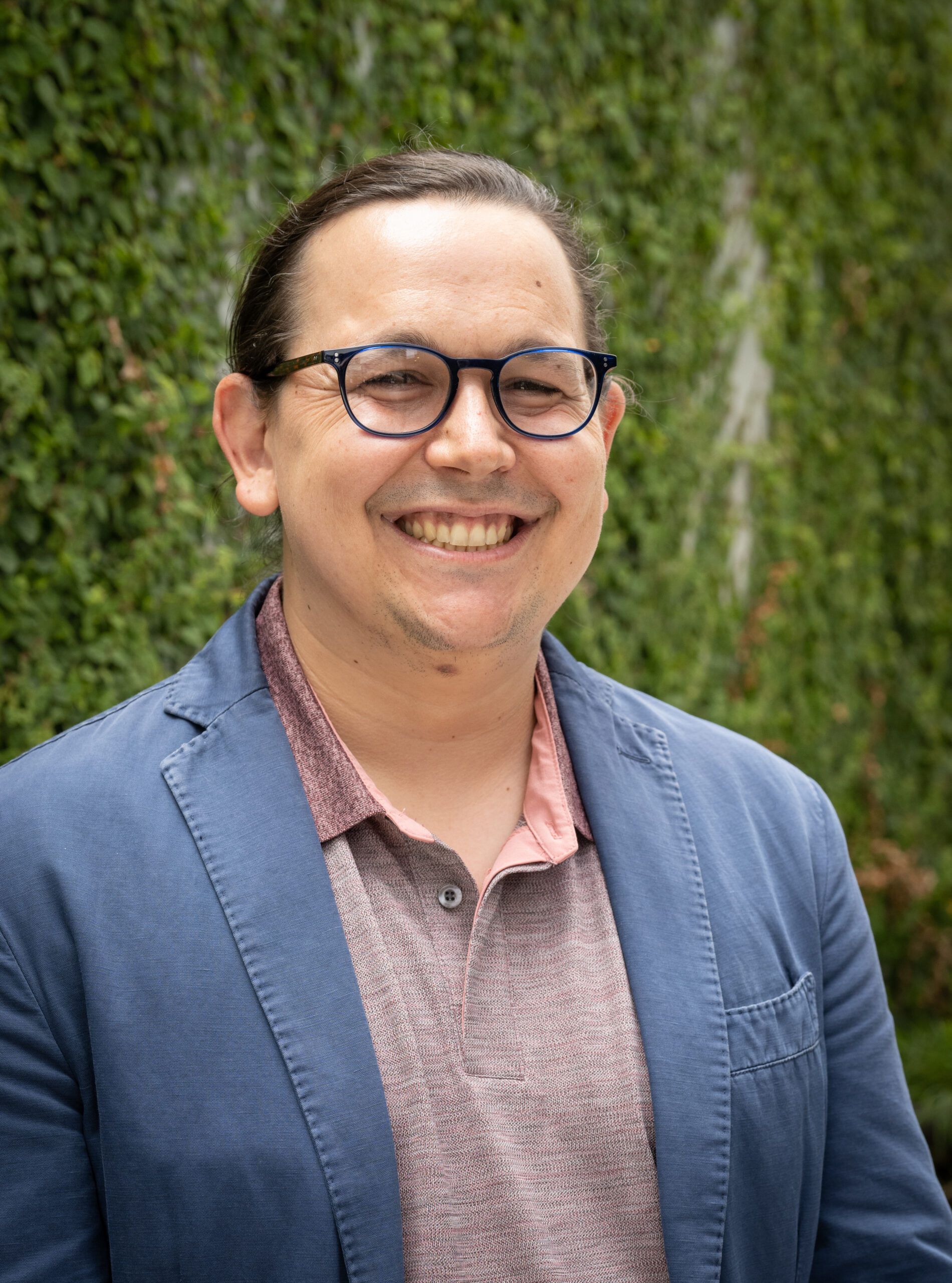
I think we will have a future where we will want to have a well-developed set of ethical standards and norms to govern research conduct with AI systems. I think that regulation can play a central role in helping shape these standards and norms but I’m also wary of overly prescriptive regulation for new technology.
There is a famous quote from I.J. Good (1965): “Let an ultraintelligent machine be defined as a machine that can far surpass all the intellectual activities of any man however clever. Since the design of machines is one of these intellectual activities, an ultraintelligent machine could design even better machines; there would then unquestionably be an 'intelligence explosion,' and the intelligence of man would be left far behind. Thus the first ultraintelligent machine is the last invention that man need ever make, provided that the machine is docile enough to tell us how to keep it under control." What is your feeling about this?
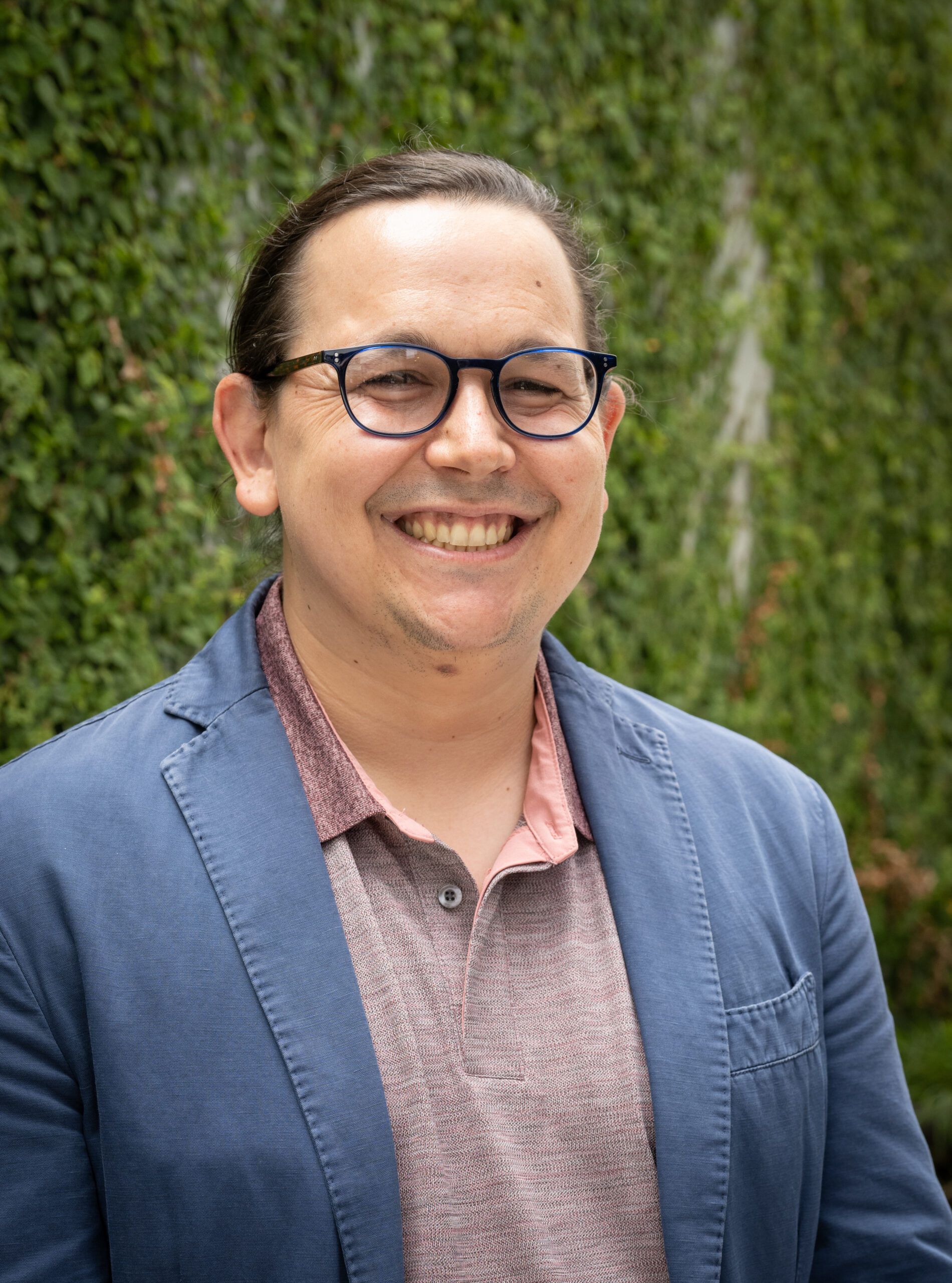
I think it is a good quote and it paints a nice picture about the promise and risks of AI systems.
While I would not rule it out, I tend to think that the idea of an ‘intelligence explosion’, is a good deal more questionable than I.J. Good does. This is because I think that ‘intelligence’ is a complicated property with multiple competing dimensions and I’ve learned from experience to be wary of intuition in these settings.
Indeed, the way that even AI experts think an AI will go about solving a problem is rarely the same as the highly optimized approach that AIs ultimately take to solving problems. They really are alien intelligences, not merely artificial.
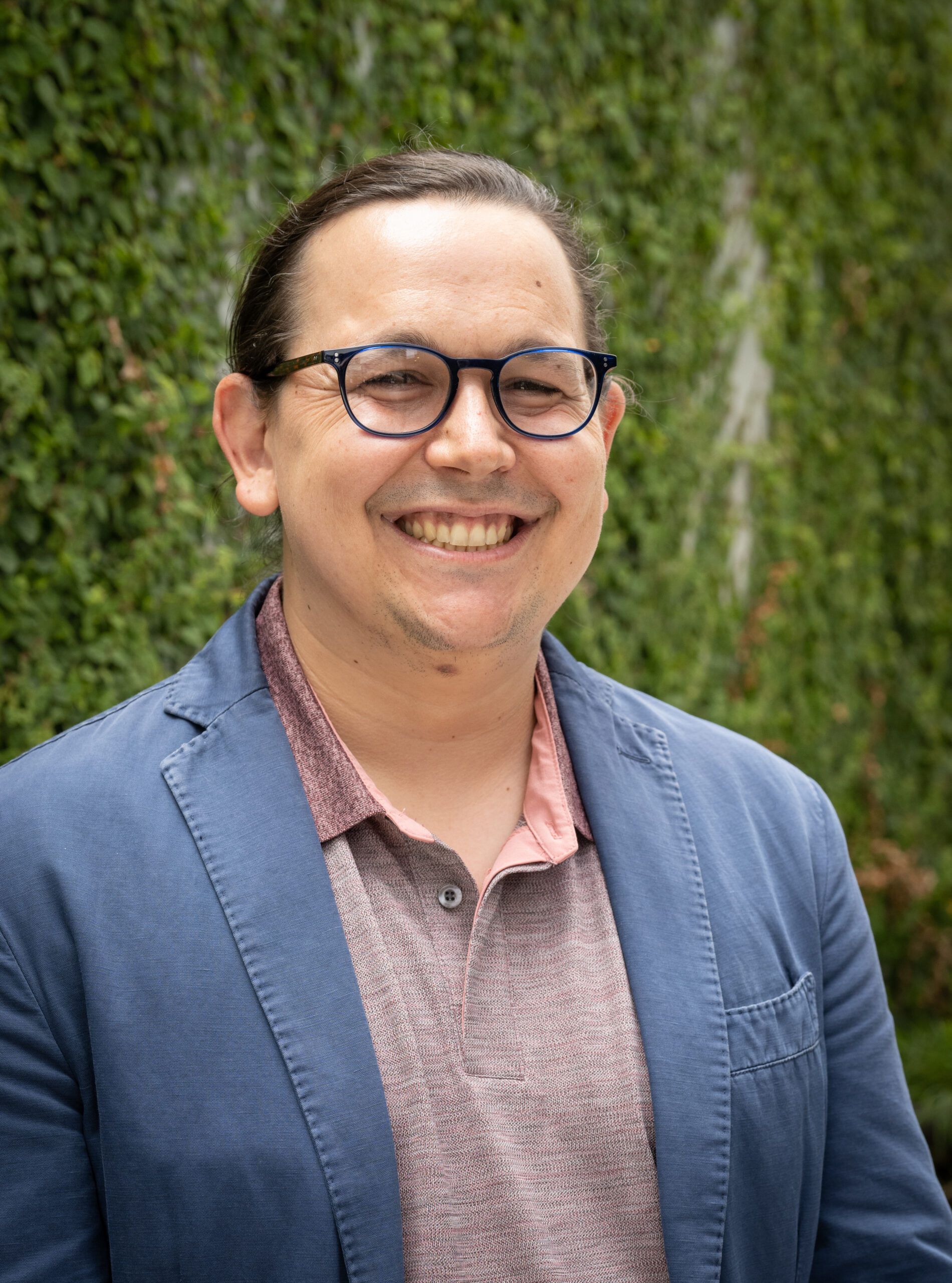
I do think we are developing systems that will transform the ways we interact with each other and the world and there is a positive feedback loop between these systems that creates a type of recursive improvement [when the improvement can be used to improve itself]. However, I view that as occurring in aggregate [across multiple organizations] as opposed to within a single digital entity. In summary, the [I.J. Good] quote resonates and I tend to think that it is correct in spirit but I don’t take it as literally as some people do.