Elizaveta Semenova is a lecturer (assistant professor) at Imperial College London. With a PhD in epidemiology and experience in both mathematics and computer science, her AI2050 project seeks to revolutionize methods for disease mapping.
“Disease mapping has been used for analysis and communication in public health since the 19th century. However, its modern methodology is falling behind compared to other computational fields,” she says.
Learn more about Elizaveta Semenova:
Your research involves Bayesian hierarchical models. Can you briefly explain what these models are, and why they are a good match for disease mapping?
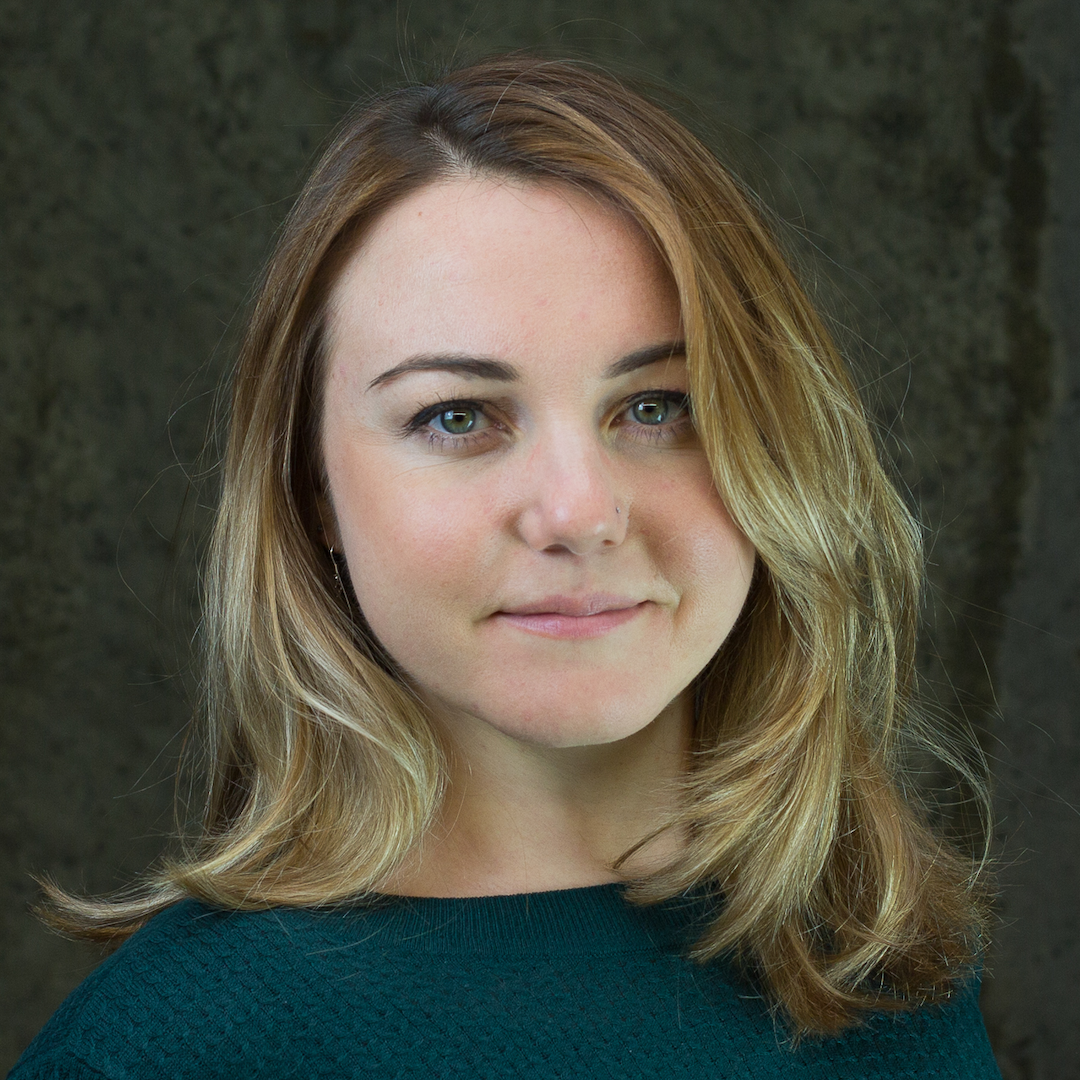
Bayesian hierarchical models are statistical models that estimate parameters using Bayesian methods across multiple nested layers. Each layer’s parameters may depend on those of another, allowing these models to integrate observed data and prior beliefs effectively. Such models are commonly used for disease mapping due to their ability to handle spatial dependence in disease data, characterize uncertainty of any produced estimates, and, when applicable, incorporate multiple sources of information.
Spatial dependence: Disease data often exhibit spatial correlation, meaning that neighboring locations are likely to have similar disease exposure and outcomes. Bayesian hierarchical models can explicitly account for this spatial dependence, allowing for more accurate estimation and prediction of disease risk across different geographical regions.
Borrowing strength: Disease mapping often involves estimating disease rates in areas with limited or no data. Bayesian hierarchical models can “borrow strength” across neighboring regions by sharing information that result in more informed predictions in geographies with sparse data.
Incorporating many covariates [factors]: Disease occurrence is influenced by various factors such as demographic characteristics, socioeconomic status, and environmental variables. Bayesian hierarchical models allow for the inclusion of [these] covariates, enabling researchers to explore the relationship between these factors and disease risk while accounting for spatial dependence [neighboring locations are likely to have similar disease exposure and outcomes].
Flexibility and uncertainty quantification: Bayesian models provide a flexible framework for incorporating background knowledge, expert opinions and different statistical assumptions. They also quantify uncertainty in the estimates and predictions, providing a more comprehensive understanding of disease patterns.
What diseases are you mapping, and where is the work being done?
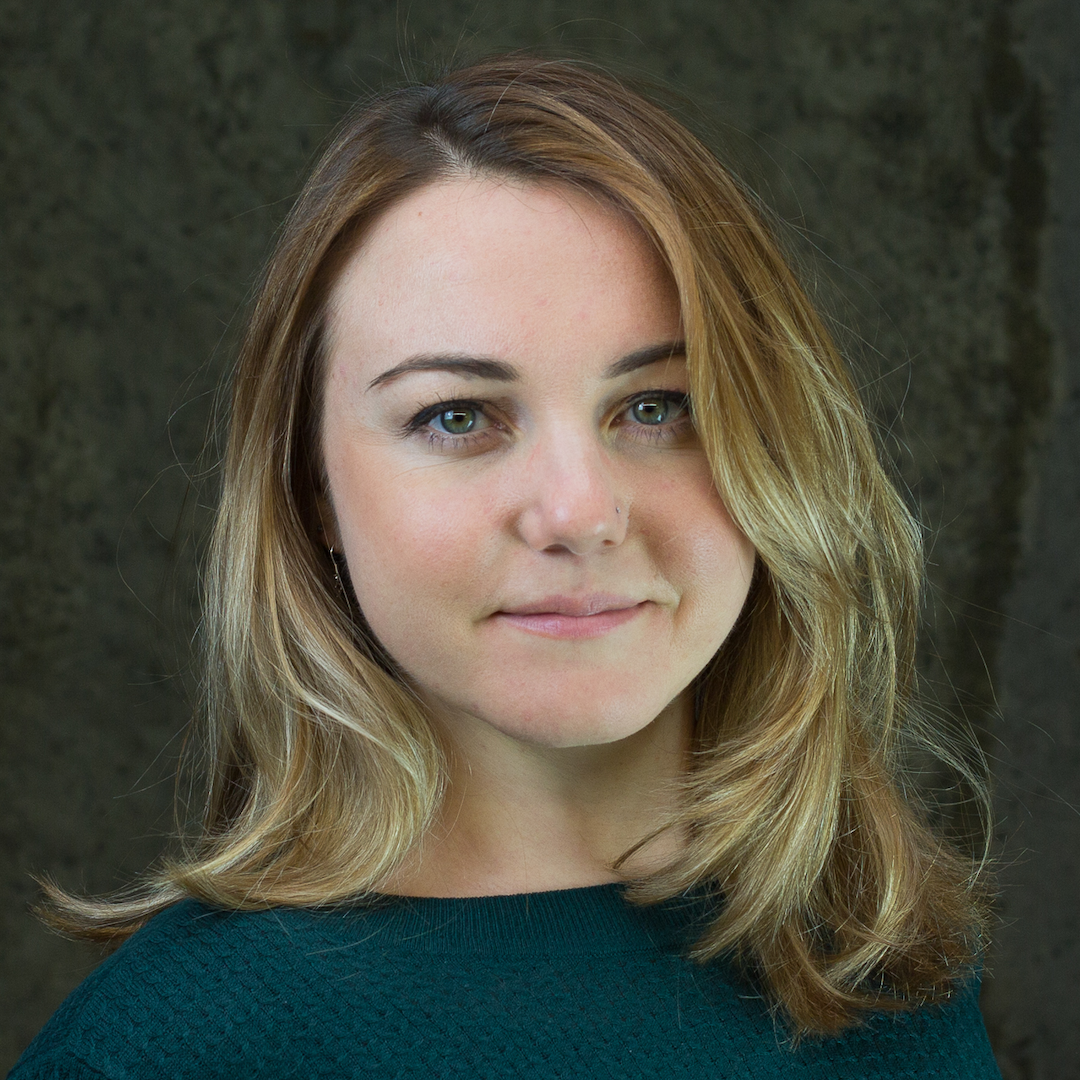
The spatial modeling method that I work on is disease-agnostic. So far, I have applied it to map HIV in Zimbabwe, and Covid-19 in the UK. Going forward, I am interested in collaborating with epidemiologists on mapping of resistance to antimalarials in Africa, which could become a public health disaster. Additionally, it turned out that the methods developed for disease mapping, can be applied to a much wider range of models, such as, for example disease transmission models.
How do you validate these models?
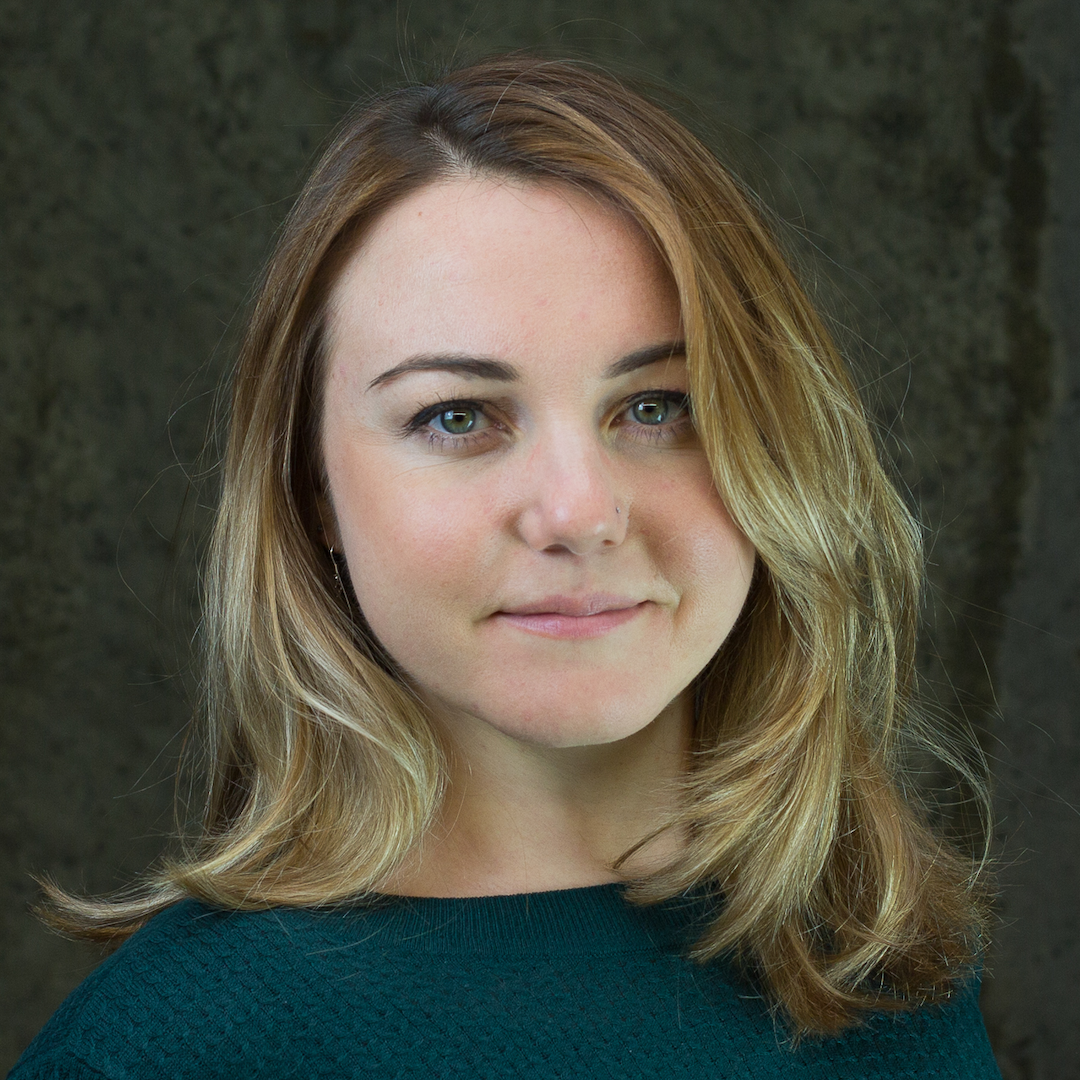
The main issue in Bayesian modeling for disease mapping is the spatial component. Modeling here is prohibitively computationally expensive, limiting the feasibility of the methods in real-world tasks. I work on alternative statistical and machine learning methods that allow these models to have much better computational properties. To validate such a model, I assess the original model, which is being held as the ground truth, against the one with the more computationally efficient method.
What are you using for data?
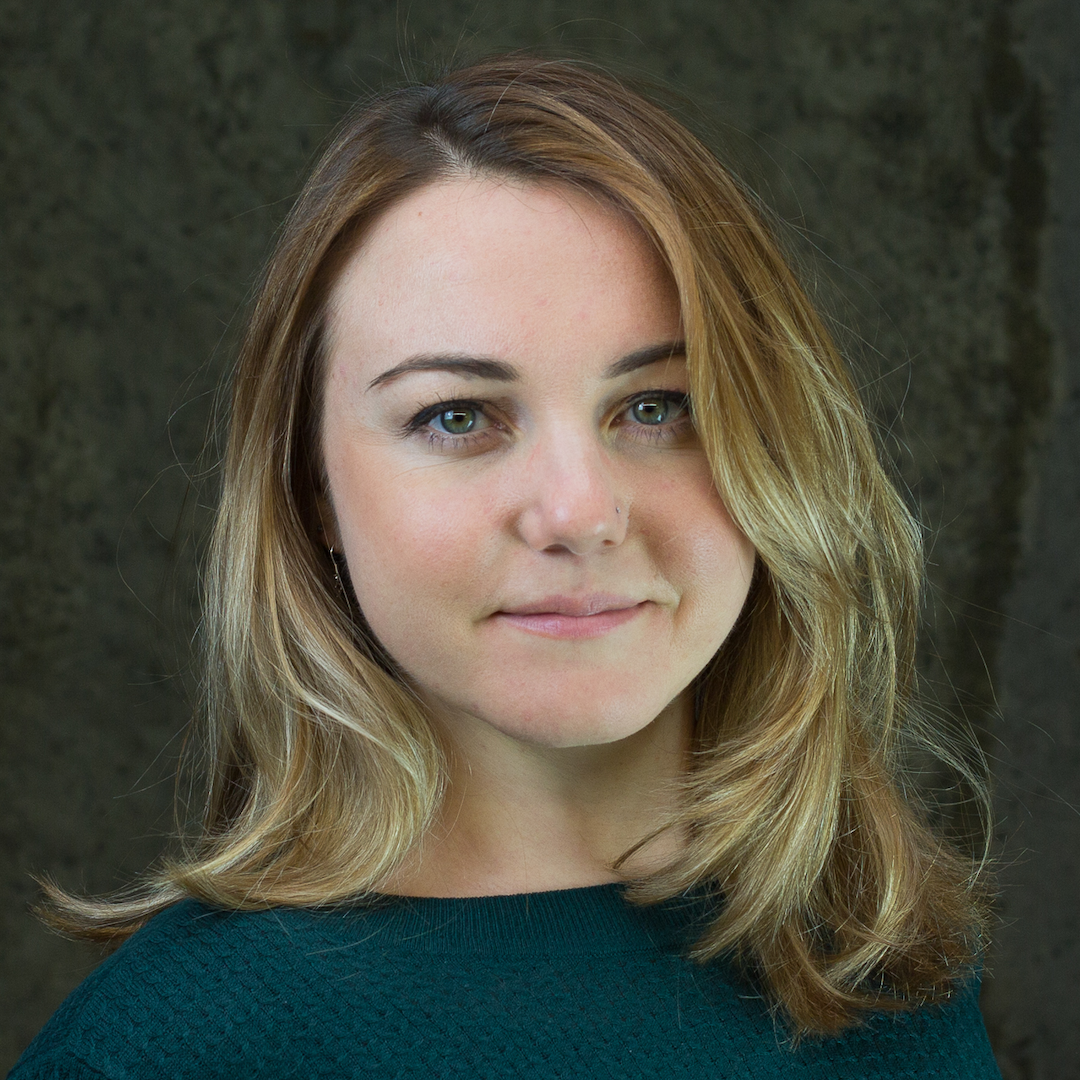
For disease data, I often rely either on publicly available sources, or data which can be obtained upon request from survey programs, such as DHS (Demographic & Health Survey).
Are there opportunities for improving data collection? Could it be automated?
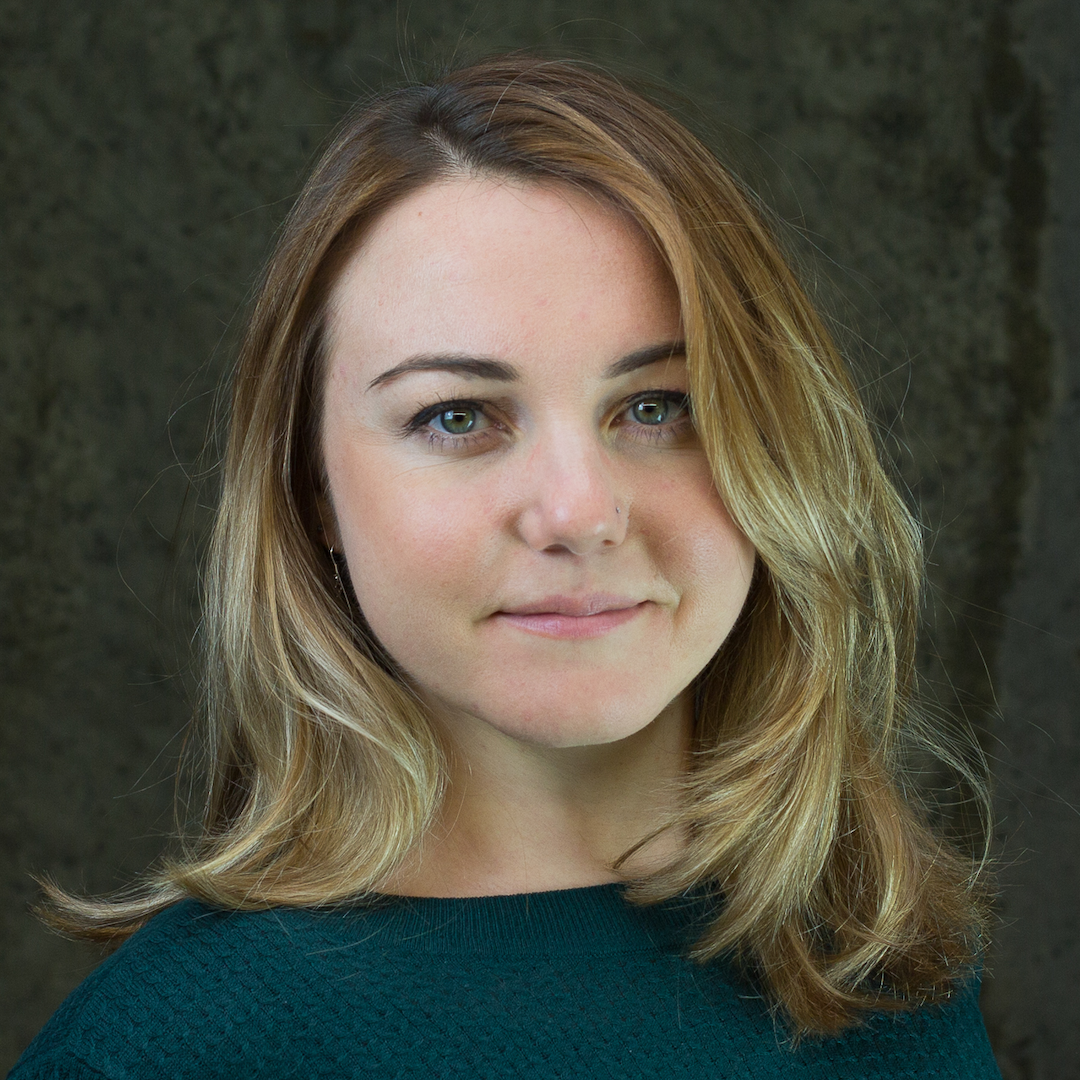
There are both opportunities and a necessity to improve data collection, especially in resource poor settings. Survey research is struggling with low response rates which can lead to biases in collected data, and a fragmented landscape for reaching potential respondents. With a group of collaborators we are working on a survey methodology, allowing the sampling to become adaptive to observed response patterns and analysis needs.
You previously applied Bayesian neural networks to predicting the toxicity of drugs in the human body. Do you think that Bayesian neural networks are under utilized in AI today, compared to other kinds of neural networks?
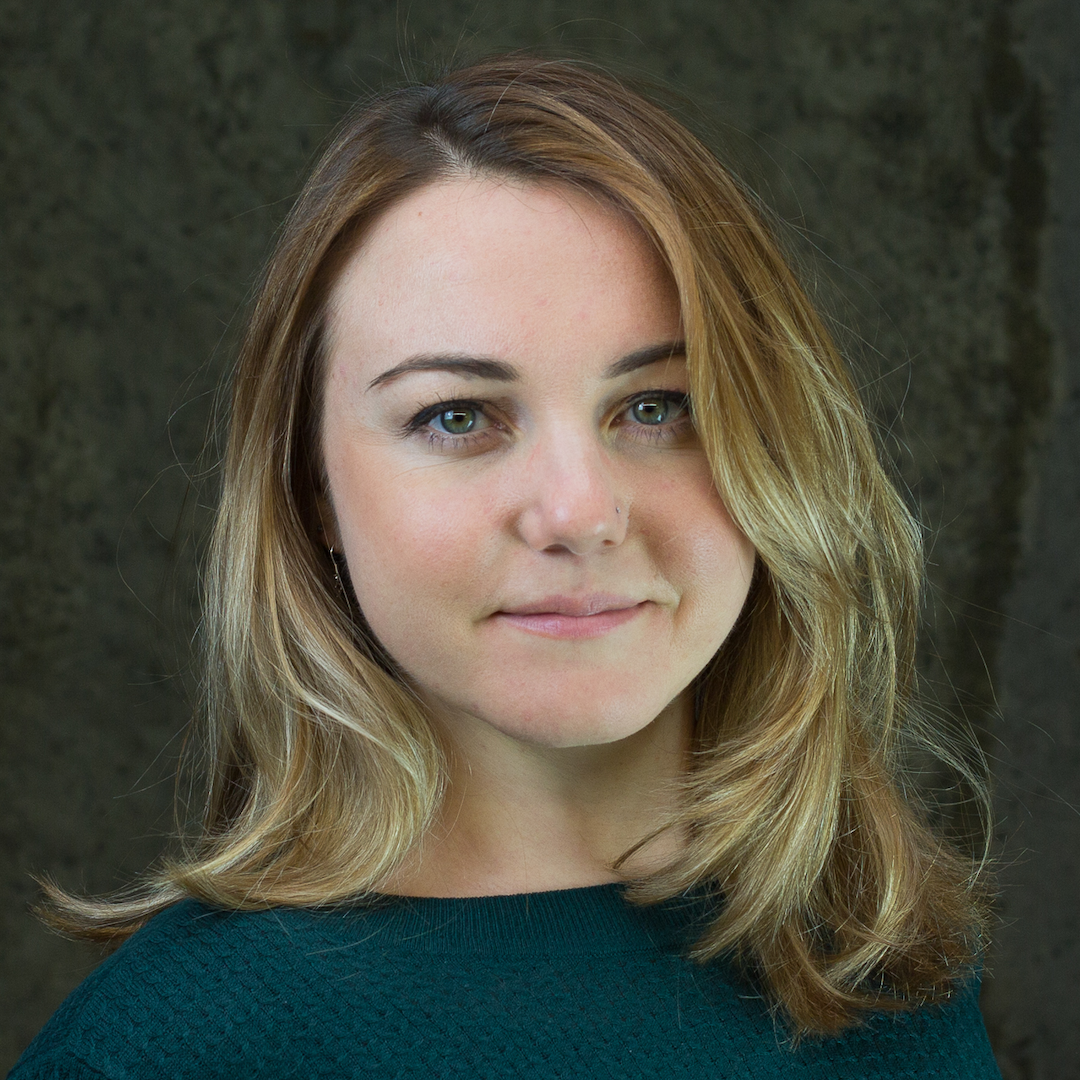
Bayesian neural networks, at the first glance, are a great tool because they combine advantages of Bayesian inference and neural networks. Neural networks allow us to construct very flexible models but are prone to overfitting; the Bayesian approach can incorporate prior knowledge, prevent overfitting, and propagate uncertainty from model parameters into model predictions. However, such models are computationally challenging. It is only possible to perform inference for such models with Markov Chain Monte Carlo for small datasets. In other scenarios, approximate methods can be used, such as Laplace approximation or variational inference approaches, and they constitute ongoing research.
What do you see as your hardest problem over the next two years?
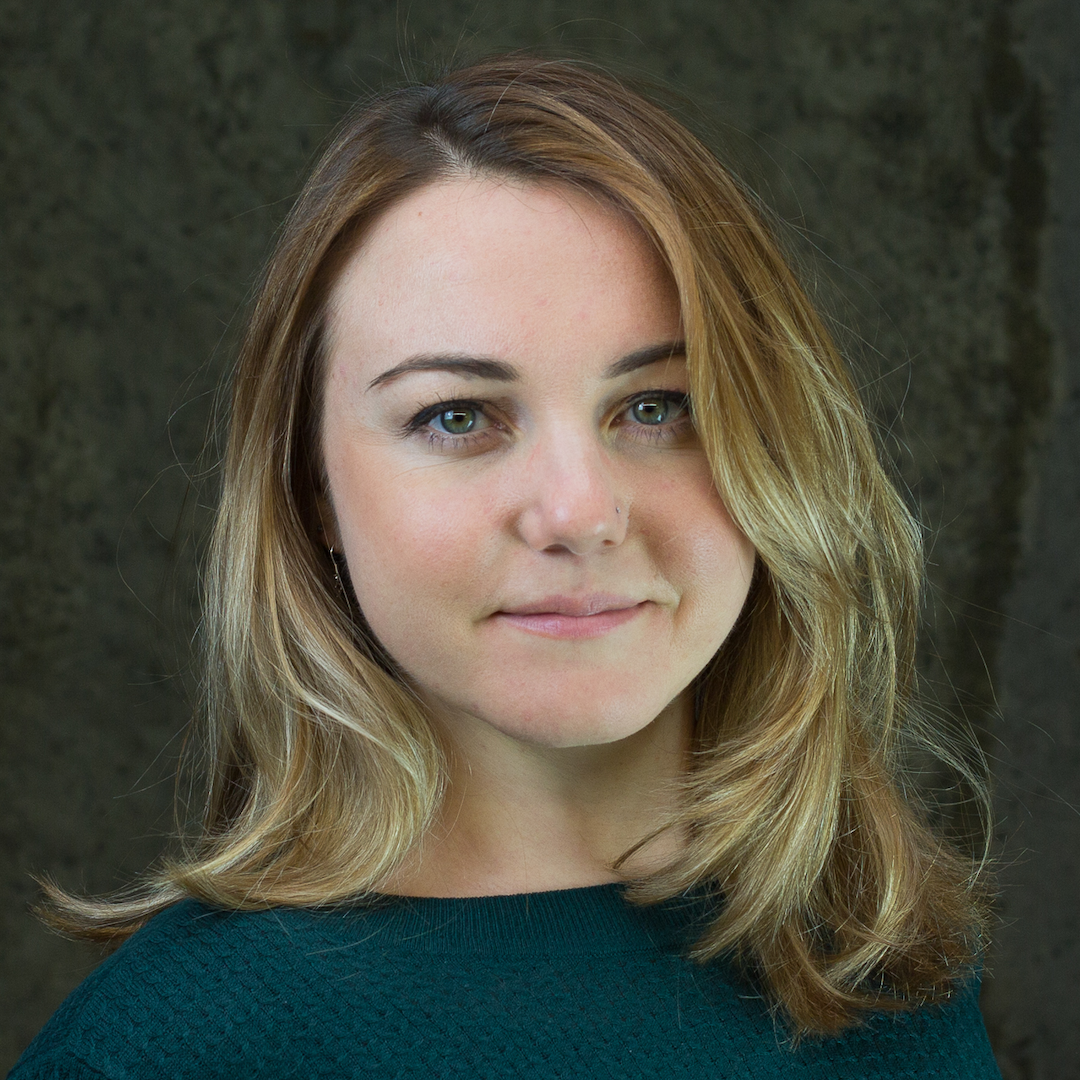
While obtaining initial proof-of-concept results for the main method in the fellowship was not too hard, further gains may not come as quickly. At the same time, the method uses deep generative modeling at its core and this area is evolving so rapidly. Hence, I’ll need to stay attentive to new advances while distinguishing which are relevant for my specific research goals.