For many of us, browsing videos on YouTube or scrolling through Instagram is an everyday experience. What we’re less familiar with, however, are the algorithms that shape our experience on online platforms. Haifeng Xu builds tools to understand how algorithms impact the way we interact with content on platforms — with a focus on how AI-generated content might be reshaping those interactions. As generative AI transforms the way we create and consume content, Xu foresees the need to design online platforms that facilitate this transformation sustainably, supporting users and creators on these platforms.
“The problem I saw when chatting to our partners in industry is that research teams are each responsible for one step in the pipeline,” says Xu. “The advantage we have in academia is to view the entire system from a global perspective, as an ecosystem.”
Haifeng Xu is a 2023 AI2050 Early-Career Fellow and an Assistant Professor in computer science at the University of Chicago. He directs the Strategic Intelligence for Machine Agents (SIGMA) lab which designs intelligent AI systems that can learn and act in complex scenarios. He has been recognized by multiple awards, including Best Paper Award at the Web Conference, an Early Career Spotlight at the International Joint Conference on Artificial Intelligence and a Google Faculty Research Award.
Xu studies the economics of machine learning, including the economic aspects of machine learning and the applications of machine learning algorithms to economic problems. His AI2050 project develops computational methods to understand how generative AI impacts online content creation. He hopes this insight can be used to design online platforms that facilitate a healthy content creation ecosystem This project addresses Hard Problem #5, which concerns solving economic concerns and challenges that arise as a result of AI technologies.
The AI2050 initiative gratefully acknowledges Fayth Tan for assistance in producing this community perspective.
Most people have watched a YouTube video or done a Google search before, but not many would know how machine learning plays a role in designing these platforms. How would you introduce your research to a general audience?
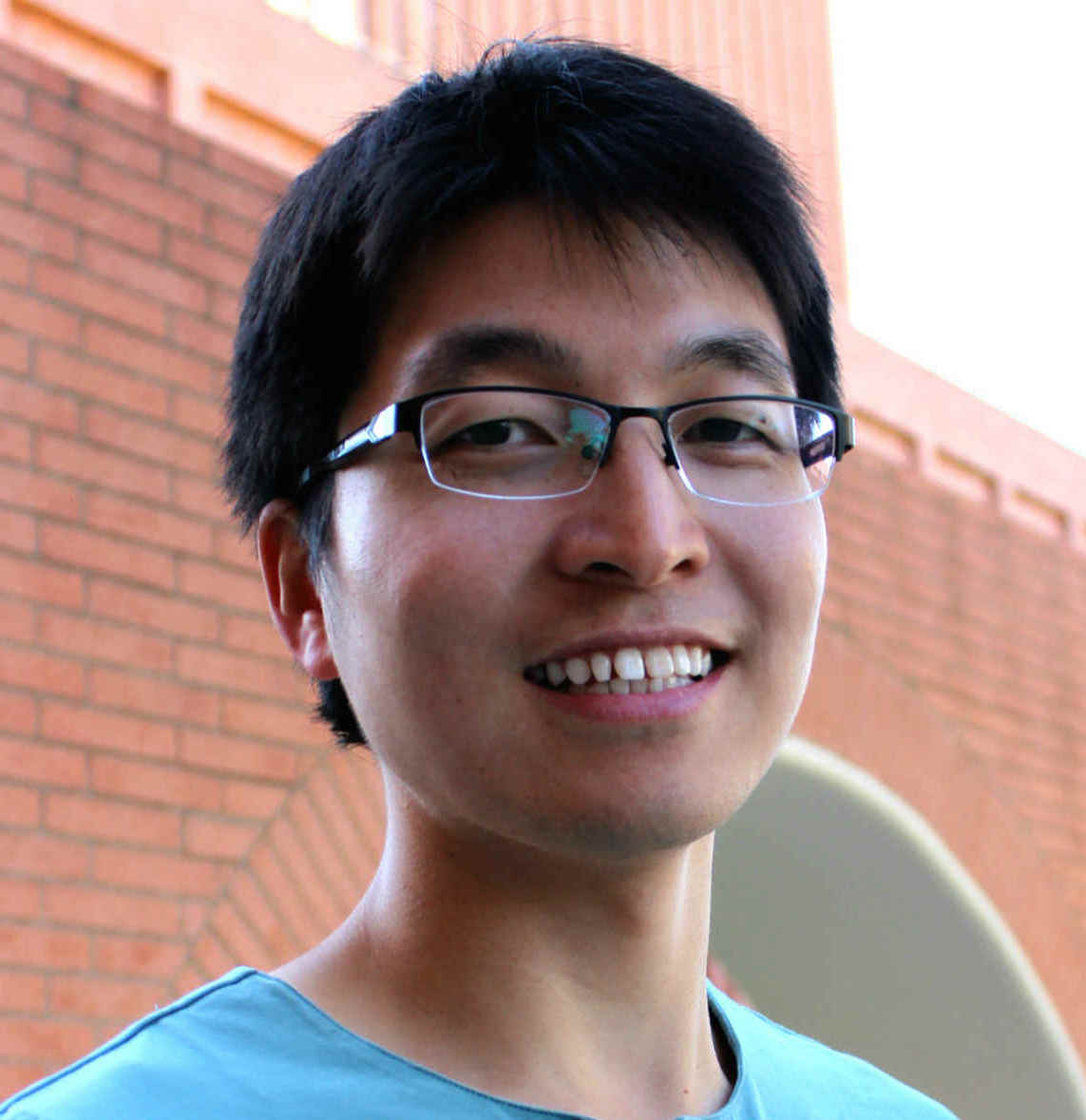
I study optimization and machine learning in a strategic or economic context. My research has shifted to online platforms, [as] I realized that many online platforms are designed to be functional, but not optimized. Currently, many social media platforms are highly skewed to “hot” topics like pop music or sports, instead of niche topics like classical music or history. There are a significant portion of users — those who are interested in niche topics — that are underserved. My research uses computational tools to study this optimization problem in an economic context. You need to account for different content and content creators’ incentives, and you need to look at the effect of their content on user happiness in order to optimize the welfare of the entire system.
In viewing platform design as an optimization problem, why is it important to take into account all those different factors and perspectives?
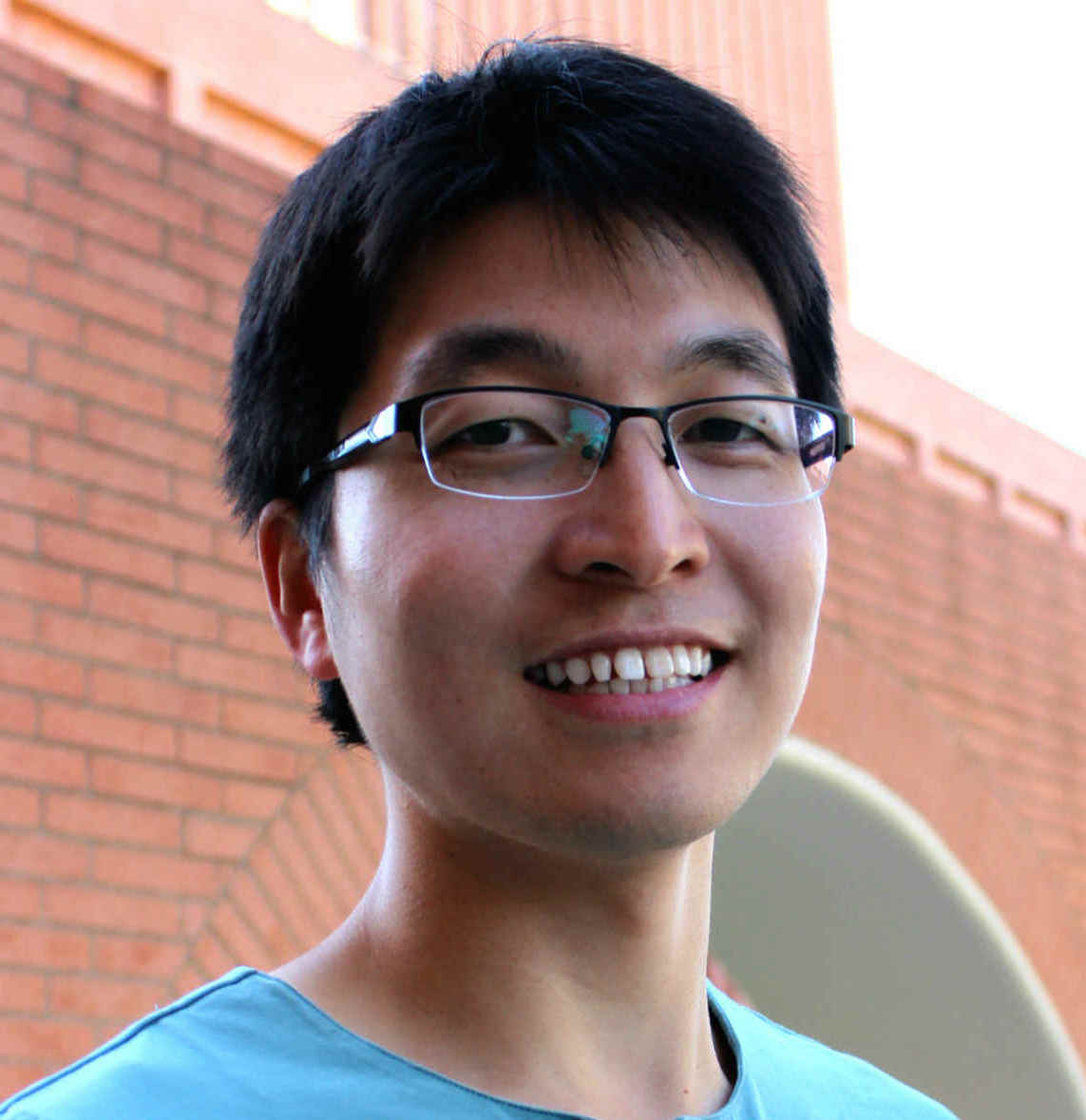
It helps us to understand the behavior of each stakeholder. Understanding those behaviors is important, especially if you want future predictions about their behavior—you want to understand the causal consequence for any change you’re making. If you take those consequential behaviors into account, that often makes your optimization much more effective. Otherwise, you might improve accuracy at the expense of people’s overall welfare. By taking long term behavior into account, you can avoid this kind of surprise, [when] you thought you’d developed a better technology, but [it] ends up actually making [the platform] worse.
Online content ecosystems are already complex — how might the advent of generative AI impact them in the future?
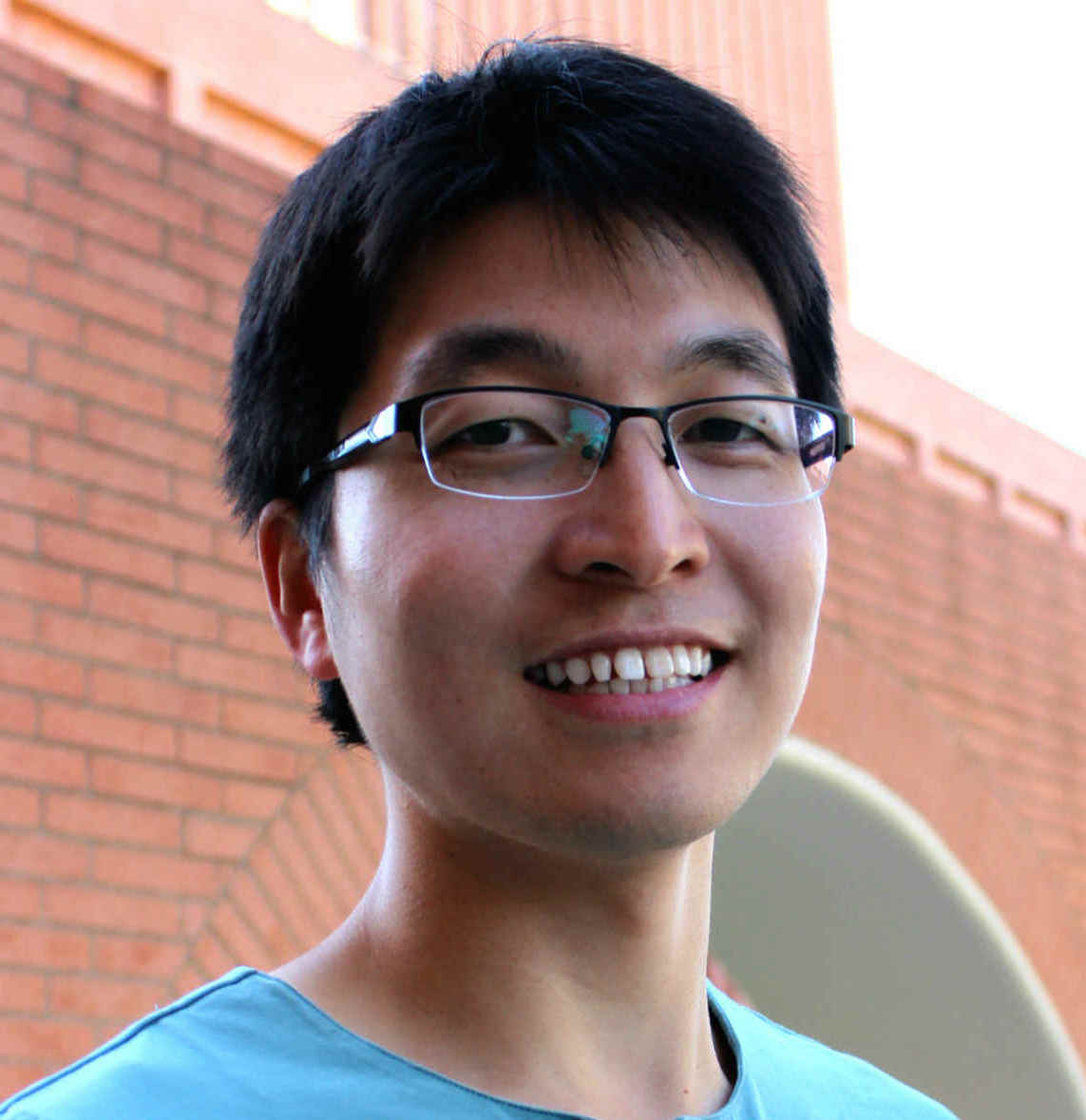
I think generative AI is going to significantly reshape this online content creation, which is why I think it’s a critical time to understand [its] impact, and more importantly, how we can drive this trend in a sustainable and healthy way. The fundamental reason is pretty simple: generative AI is an unprecedented way of creating content. It’s super fast—if a human wanted to take a photo, you’d need to go outside and pick the right angle, but with generative AI, that could take seconds.
Many people earn a living from content creation. If you suddenly need to compete with another tool that can create so much faster than you, how would that change your incentives? Maybe more and more people will be driven out of the system if we’re not designing rewarding mechanisms well, and people who know how to use generative AI technology will become more and more dominant in the platform. There’s also the worry that in the future only a few giant GenAI companies will be creating content on the entire Internet, and that’s something we probably don’t want to see.
Another concern is that once much of the content is created by AI, there will be less human-created content. Models need a lot of high-quality, human-created content to train, refine, and improve them. If humans don’t have any incentive to create content, who is going to provide the data for us to train those models? I think that that is why we are already starting to see a shift in the industry where companies like Google and OpenAI buy data from content creators. This seems to be how the ecosystem is being remade. Content creators like the New York Times get less revenue from people visiting their website because people will probably use AI to get a summary of their news, but get compensated from collaborating with these platforms [instead].
You model the dynamics of human creation versus AI creation. How do the two interact? Do the dynamics differ depending on the type of content created?
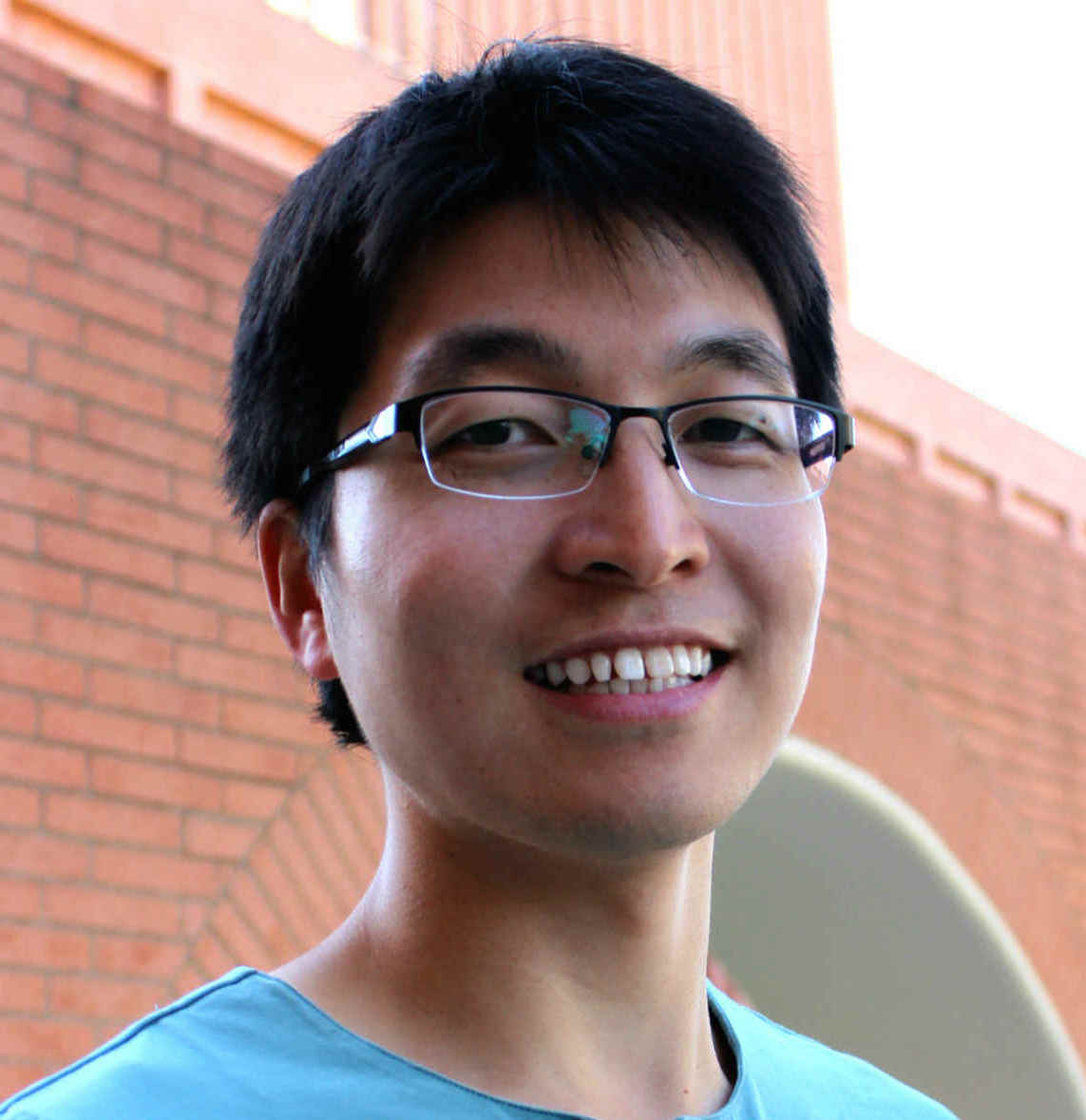
Our first paper was on a relatively simple system. We didn’t distinguish the different styles of content, the popularity of the content decayed [over time] at an equal speed, and the difficulty of creating the content was equal. In such a system, we found that AI would supplement human content creation, but it will never override it. We found that it may be a useful tool to augment our behavior, and that was a very encouraging finding.
In my more recent work, we [looked] at content about topics with different time sensitivity. For example, the value of news decays quickly over time, because news from one week ago might not be useful any more. But for other things, like historical facts, or basic knowledge about math — that’s going to be true for the next 100 years. For different kinds of knowledge, we’re trying to understand what kind of topics might be more easily substituted by AI, and what the pattern of the human content creation behavior will be in the future. Will we just shift our focus to less easily substituted topics? On the system level, or the platform level, how can we design the right incentives for different topics and content creation such that we can guarantee that every topic has the desired speed or renewal of content generation?
Another question we’re studying is about how generative AI is going to affect human creativity. I think there are two possibilities — one is generative AI somehow forces us to innovate, because whatever we have done, they can easily copy, so we’re just forced to create new things. That’s good for creativity or innovation. But another possibility is that the competition is so enhanced that people just lose any incentive to really create new things. We’re trying to see what we will do when faced with those possibilities.
How do you think generative AI will change how online content is made and distributed in the future?
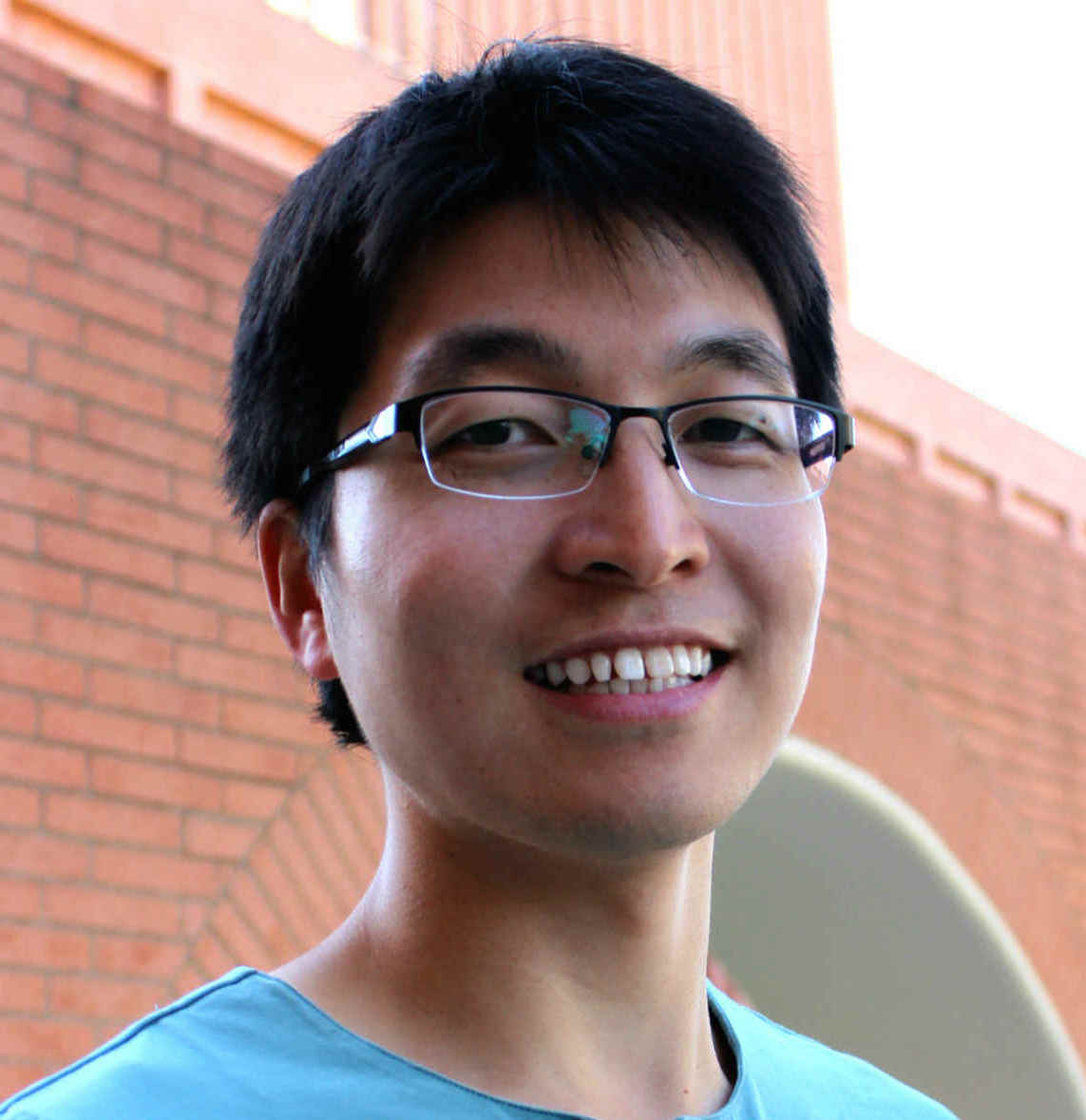
My guess would be that there will be more targeted content creation. For specific tasks, I imagine there will be more specialized data curation companies. Currently, content creators are mostly individuals, and individually, bargaining power and [the ability to] act on an agenda is very limited. I also imagine there will be data broker companies which hire a lot of content creators. The company could accumulate content, negotiate a price with a large AI company like Google or OpenAI, and redistribute the revenue to their creators. In fact, in some sense, media companies like the Wall Street Journal are already doing this — OpenAI recently announced a deal with them to access their news data.
In the content creation space, creators have expressed excitement or anxiety about the changes brought about by generative AI. How would you communicate to creators the ways in which their role or their livelihood might be in the future?
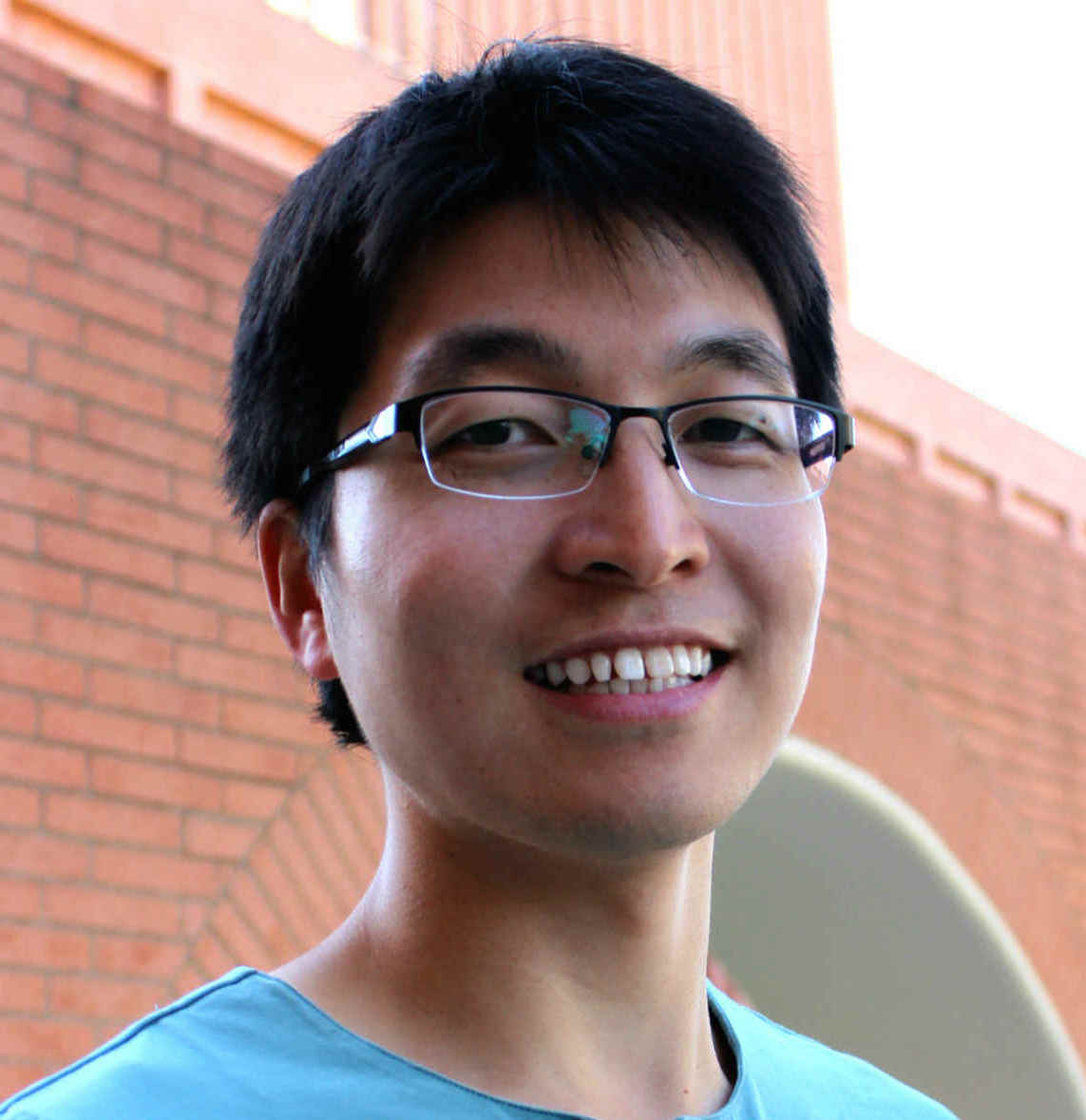
I think it’s a challenging time — there’s definitely a lot of opportunities but I do understand that there are worries as well. I believe that the general trend should be [that] high quality, authentic data will become more and more valuable. Perhaps the way that data is transmitted to a platform might be different — maybe you’d just give your data to an AI company directly. Your data will not directly be seen, but that doesn’t mean it’s not useful. It contributes a lot to improving the AI, and I think creators could also make a livelihood with it.
But of course, that requires the platforms like OpenAI and Google to design the correct incentives for content creators. That’s also challenging, but it’s happening already — Google is paying a lot to buy data from Reddit, and OpenAI is paying a lot to license material from news companies. We’re definitely seeing this kind of data purchase more and more often, so that’s a new path content creators can make use of. I think it’s a good time to think about how to navigate these challenges. One possible way is that [content creators] could group together to become a platform so that they have bigger bargaining power to benefit from their creation.