Percy Liang is an Associate Professor of Computer Science and Statistics at Stanford University, where he is the director of the Center for Research on Foundation Models (CRFM). His research addresses Hard Problem 2 (Public Trust) by making machine learning more robust (able to adapt to new situations and avoid failure), fair, and interpretable; and making computers easier to communicate with through natural language.
According to Professor Liang, foundation models “redefine the way we conceive of AI and technology.” These are large, highly capable, general purpose machine learning models trained on broad data so that they can be adapted to a wide range of tasks. “The most famous examples include language models such as GPT-4, ChatGPT, PaLM, and LLaMA and vision models such as DALL-E and Stable Diffusion.”
While foundation models exploded into popular consciousness with the release of Chat-GPT, “there has been a lack of a systematic and unified approach to studying, measuring” and comparing foundation models,” he says. Measuring foundation models according to multiple metrics, such as accuracy and adaptability to new information will enhance our knowledge of their capabilities and limitations.
Learn more about Percy Liang:
Your AI2050 project involves measuring the capabilities and risks of foundation models like ChatGPT. How do you do that?
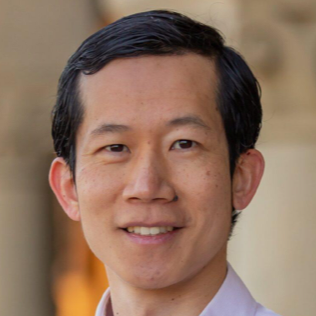
The main challenge is that foundation models are very broad, so it is difficult to determine an appropriate scope for their potential uses. Thus in 2022, we started with a project, Holistic Evaluation of Language Models (HELM), which attempts to be as principled and comprehensive as possible. We defined a set of 42 scenarios [including answering questions, summarization, sentiment analysis, toxic content detection and linguistic understanding] where language models could be applied. We defined 7 different types of metrics [including accuracy, fairness, and bias]. Finally, we obtained access to 30 state-of-the-art language models across 10+ organizations, and compared all of them consistently. The standardization that HELM provides makes it possible to compare all these language models for the first time side-by-side. Evaluation is more challenging and urgent than it ever has been in AI.
Early on, many AI commentators insisted that there is no actual intelligence or understanding in programs like ChatGPT and Bard. But more recently, philosophers like Nick Bostrom and AI researchers like Microsoft’s Sebastien Bubeck have said that the line between human-like intelligence and unthinking machinery is not so clear, and that these large language models demonstrate aspects of understanding, empathy and even wisdom. What is your opinion on this?
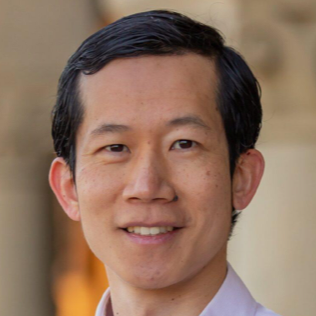
I’m not a philosopher, so I don’t have much to say directly to the “understanding” question. But I will say that it is clear that these models are capable of impressive feats. It is also clear that these models have glaring holes. If they have understanding, it’s an alien form of understanding. While humans used to be the paragon for general intelligence, I don’t think it is productive to use humans as the goal post anymore, as the standards for AI should be higher in some ways. Also, AIs can have a wild amount of impact on society even if they don’t understand (e.g., generating effective disinformation), and an excessive focus on understanding could detract from the most pressing problems in society. My view is that we ought to think of these systems as tools, characterize their capabilities, limitations, and risks rigorously, and develop them in a way so that these capabilities can be fruitfully applied without incurring too much risk.
Moving forward, do you think that these foundation models are just going to get bigger and bigger, or are they going to get smaller but more sophisticated?
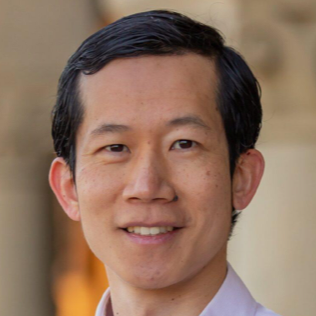
Fortunately researchers are increasingly shifting away from size. The amount of compute [processing power] and data [the two have a proportional relationship] matter much more, as does the quality of the data. Finally, deploying large foundation models is expensive, so there has been further pressure to try to do more with smaller models. For example, recently, we found that [we could] fine-tune Meta’s 7 billion parameter LLaMA model to produce a new model, Alpaca, that is surprisingly good.
Alpaca is much smaller than ChatGPT and Bard — in fact, it’s so small that you can download and run Alpaca on a Macintosh laptop, but it’s really slow. Do you see a future where people can be training and running their own foundation models on personal computers—not just as a demonstration, but to get real work done?
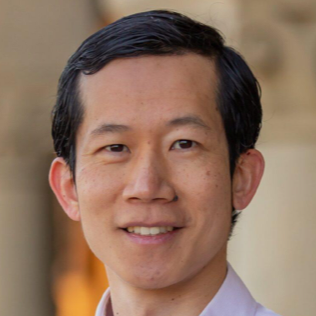
By the very nature of foundation models, I think base models will require substantial capital and be trained in the cloud. But I expect that it will be possible and desirable to fine-tune an Alpaca-sized model on consumer hardware for privacy reasons. Meanwhile, we will see innovations in hardware that make it possible to do more, as well as modeling innovations that allow us to reduce the hardware requirements. These two trends will make running powerful foundation models on consumer hardware increasingly feasible in the coming years.