Sina Fazelpour is an Assistant Professor of Philosophy and Computer Science in the Department of Philosophy and in the Khoury College of Computer Sciences at Northeastern University. He is also a visiting AI fellow at the US Government’s National Institute of Standards and Technology.
Fazelpour’s research on artificial intelligence examines how these systems impact justice and diversity. He is also concerned with issues of reliability and the potential consequences of deploying systems that are not perfect. His work addresses Hard Problem 2 (addressing AI shortcomings that may cause harm or erode public trust of AI systems) and Hard Problem 7 (related to the responsible development and deployment of AI).
“If AI tools are collaborating with humans, the primary unit of evaluation should be the human-AI team, and not just its individual members,” says Fazelpour.
It’s important to consider the team as a whole, says Fazelpour. Human teams typically perform differently than the individual people who make up a team, just as crowds behave differently than people do in isolation. Thus, it’s reasonable to expect that humans and AI systems teamed together will similarly behave differently than either by themselves.
Learn more about Sina Fazelpour:
Much of your work is focused on the impact of AI on vulnerable populations. Why has this aspect captured your interest?
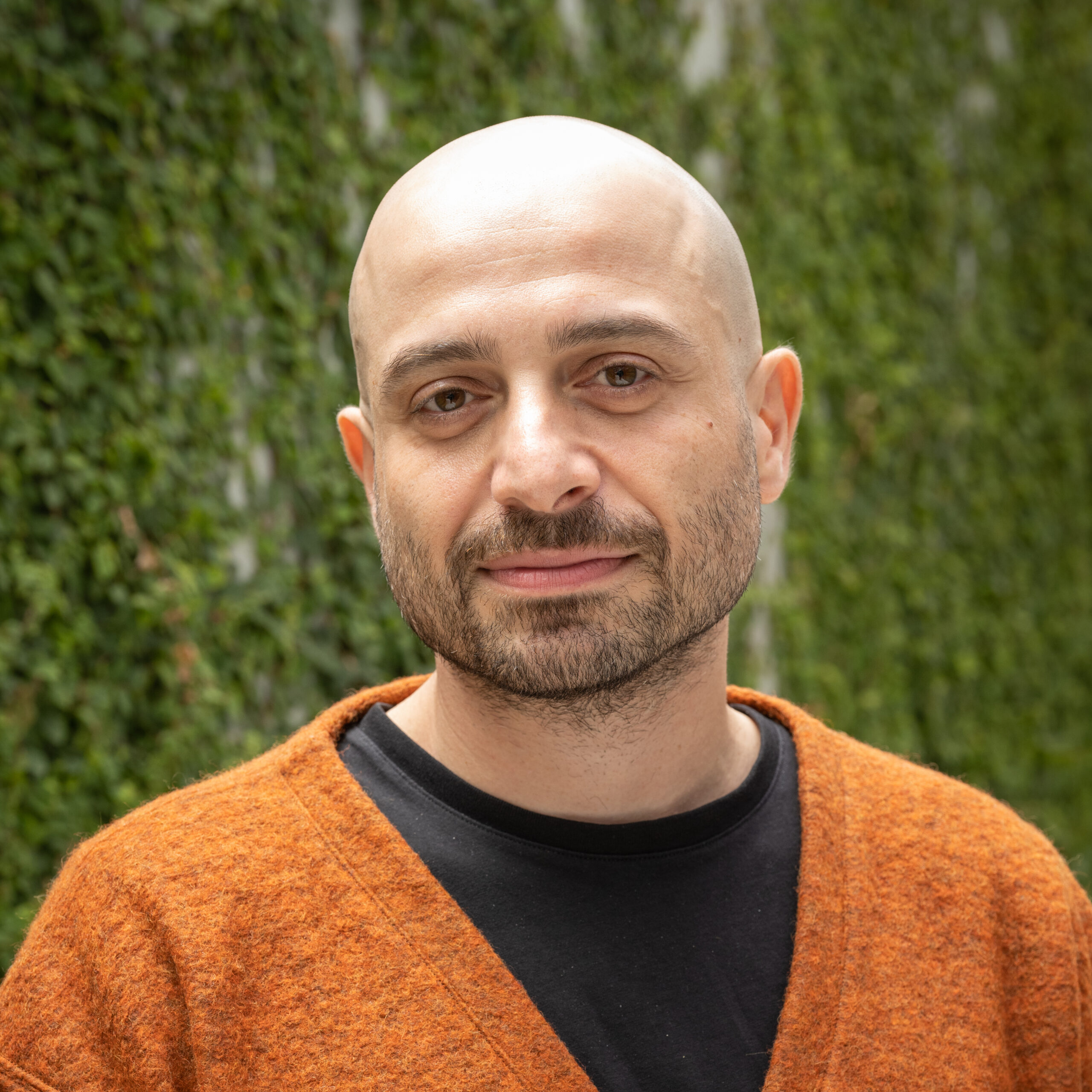
Much of my work is motivated by questions about justice and inclusion. It probably has something to do with my experience of growing up in Iran, and then that of immigration to a multicultural country like Canada during the early 2000s.
In the context of AI systems, the risks posed by these tools are not equitably distributed in our society, and their [use] can exacerbate unjust harms against already vulnerable communities. What concerned me even more was that some prevalent technical proposals for mitigating these AI-driven injustices amounted to little more than ethics washing.
As I argue in my work, some of today’s proposed solutions [for example, the use of machine learning for criminal justice or hiring decisions] focus on hiding some correlated symptom of the problem, without addressing the underlying cause. And they do so in ways that could worsen those harms particularly in our complex social systems. [As Fazelpour explains in a 2020 paper, ‘While race-blind policies are often argued on the basis that they accord with the ideal,’ it is impossible to achieve perfect ‘fairness’ in the real world, and ‘matching the ideal in some respect may only be possible at the expense of widening gaps in others’.]
I believe that it is better to carefully examine the sources of the problem, and then propose appropriate solutions. I hope to contribute to the community of research and practice that tries to face the challenge at a more fundamental level.
In my mind, this is important not only for reasons of justice and democratic legitimacy, but also because, in many cases, ethically designed AI tools can also be functionally better and offer novel opportunities.
There’s a common narrative that bias and unfairness is a result of unequal representation in training data. For example, some companies have claimed that they solved the problem of bias in face recognition by adding more photographs of non-white people to the training data. Do you think that most of the bias problems we are seeing are a result of data, or is there something else going on?
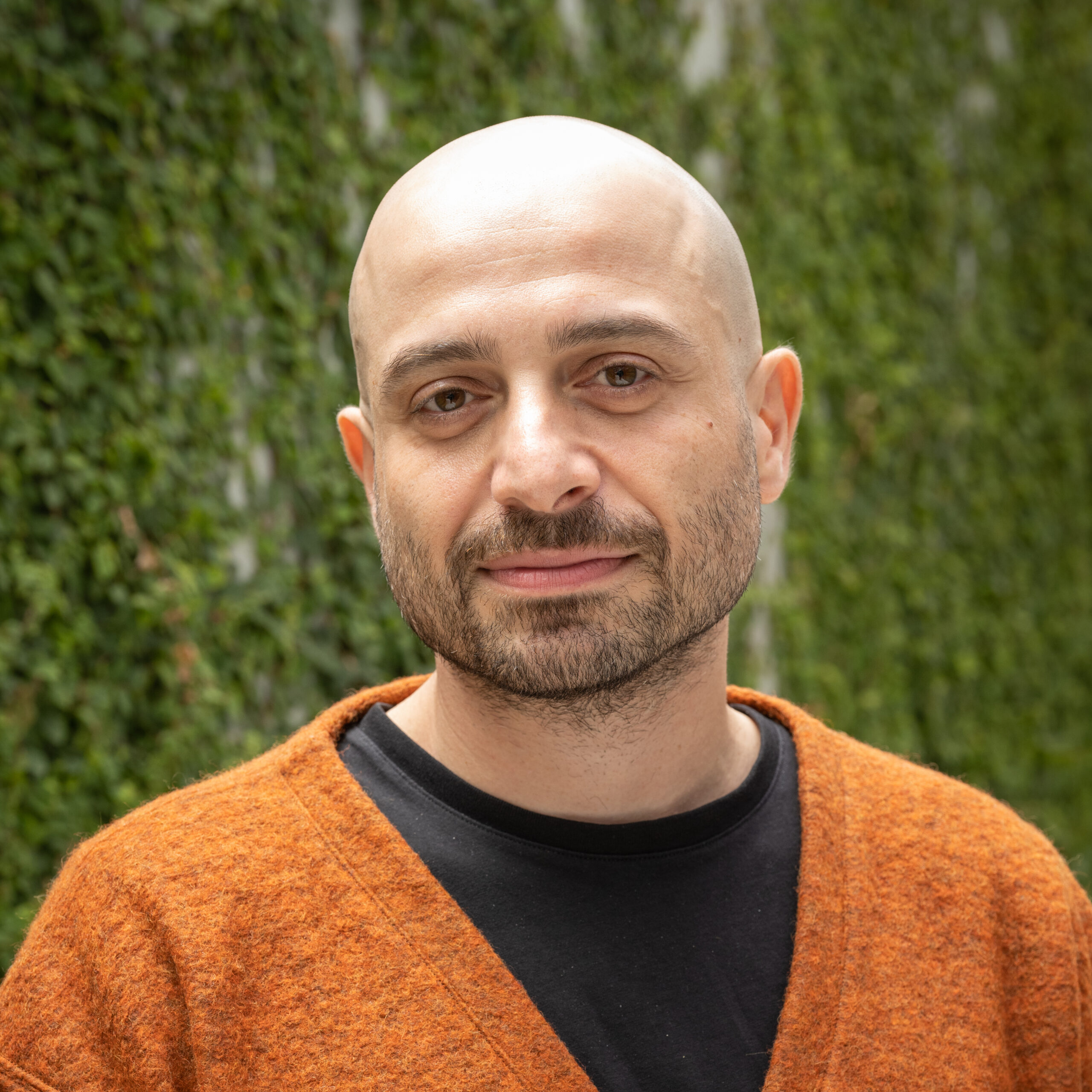
This is a good example of why we need to think carefully about the many sources of biases and devise our responses in ways that reflect that understanding. There are many choices and assumptions involved in the construction and selection of datasets.
Sampling and curation are just one part of the story; there are also choices about construct and variable creation (e.g., what do we mean by a patient in “severe pain”; by a “good” student; by “toxic” content), measurement and estimation (e.g., how are we measuring all of these; how are we estimating counterfactual quantities like whether a student, who was not admitted, would have been a good student) and values encoded in human assessments (e.g., in radiologists’ evaluation of radiographic measures of severity, in content moderators’ assessment of toxicity), dealing with disagreements that often emerge in all of these, and more.
Going beyond data, the impacts of AI systems are also shaped by many other considerations: how we formulate and justify the problem that the AI tool is supposed to solve; our choices in modeling and validation; how, given relevant human factors (e.g., users, institutional norms, social context), we structure the deployment setting such as to promote appropriate use, and how we take existing and emergent social dynamics into account.
In previous works, my collaborators and I mapped these choice points and showed how harmful biases can emerge because of how any of these choices are made. So, while those types of efforts to “diversify” datasets can address some concerns, they are by no means sufficient or even appropriate for addressing many other concerns. For example, when existing institutional dynamics makes it the case that the potential harms from misuse and abuse of an AI tool (even one trained on a representationally “diverse” dataset) disproportionately falls on vulnerable populations, then the solution is to change the organizational dynamics (e.g., via designing appropriate accountability mechanisms) or, if we’re unable to do so, not design or deploy the AI tool.
Your AI2050 project aims to create a framework that takes the human-AI team as the primary unit of evaluation. What does that mean?
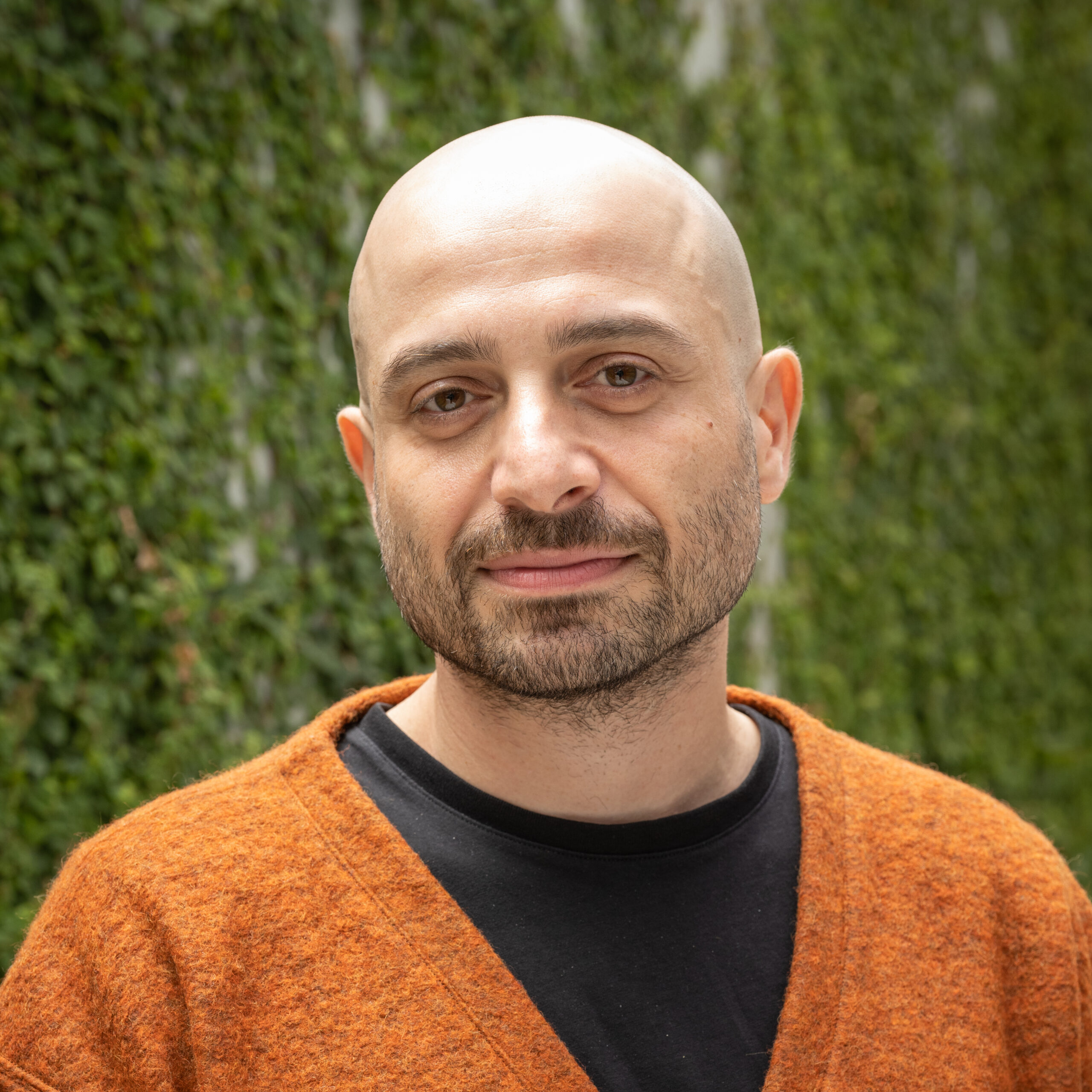
The typical ways of evaluating AI tools tend to be quite individualistic. That is, they focus on properties of algorithms (e.g., some measure of predictive performance or potential disparities in that measure across different demographic groups), use those algorithm-level properties as the basis for anticipating what the impacts in deployment would be along some dimension of interest (e.g., accuracy or fairness), and select the best individual performer (e.g., the most “accurate” or “fair”).
For reasons I have already mentioned, this approach which primarily focuses on one aspect of the AI lifecycle in isolation from other upstream and downstream decisions can turn out to be critically flawed. Particularly important, for the purposes of the current project, is the fact that in many applications of social concern, AI tools are not standalone decision-makers. Instead, their outputs are meant to assist human experts who often make the ultimate decisions.
In these cases, I believe that the unit of analysis should be the human-AI team (or AI-assisted human team) and how the distinct informational resources and capabilities of AI tools and human experts interact and combine to shape decision quality. This change of unit of evaluation has fundamental ramifications in terms of how we conceive of relevant norms that should guide choices made throughout the design, development, and deployment process. Ultimately, the aim is to provide guidance about whether and how the adoption of algorithmic tools in our social institutions can enhance functional and ethical decision quality, particularly in ways that leverage the distinct capabilities of AI tools and human experts, while keeping in focus other values such as trust, expert autonomy and organizational accountability.
Much of the work that you do seems highly theoretical. How do you make this work relevant for policymakers?
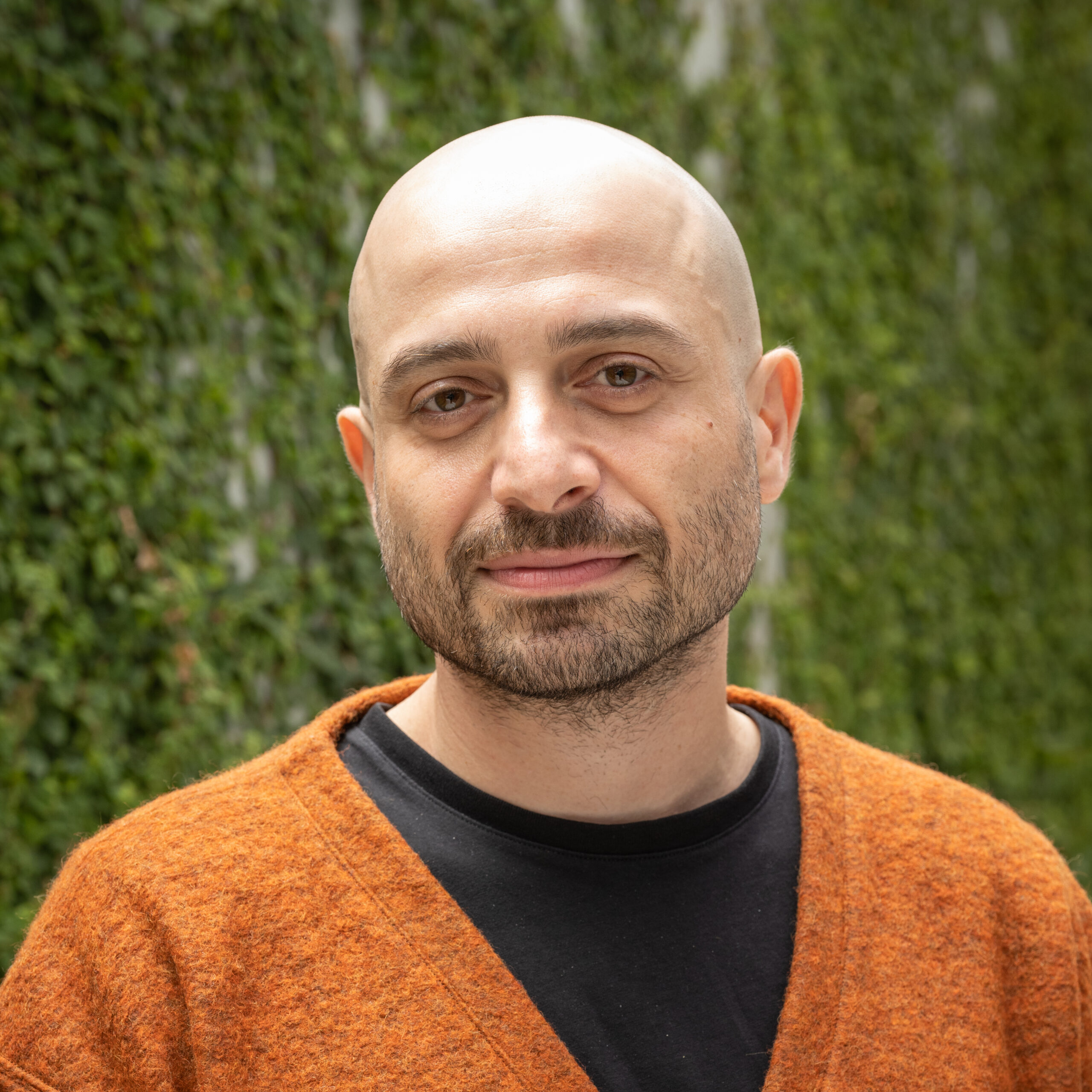
When we start thinking about how and in what capacity we can responsibly integrate AI tools into our institutions and societies, we often have to engage in work that is quite theoretical. Take, for example, some of the central concepts in responsible AI: “privacy”, “justice”, “fairness”, “diversity”, and “inclusion”. Specifying what these concepts mean, what distinct epistemic, political, or ethical rationales animate them, how they can be operationalized in context, and how they might interact with other values that are at stake are all tasks that require quite a bit of theoretical engagement.
Like any other field, not all such theoretical work will be of immediate interest to policymakers. But some will. And one way that I make the relevance of this type of work salient is to demonstrate how the work is necessary for robustly connecting our motivations and justifications for pursuing some values with measures and policies that are supposed to implement those values; or alternately, showing how lack of attention to this type of work will result in detrimental disconnects between intentions and implementations.
Looking forward, what’s your biggest challenge?
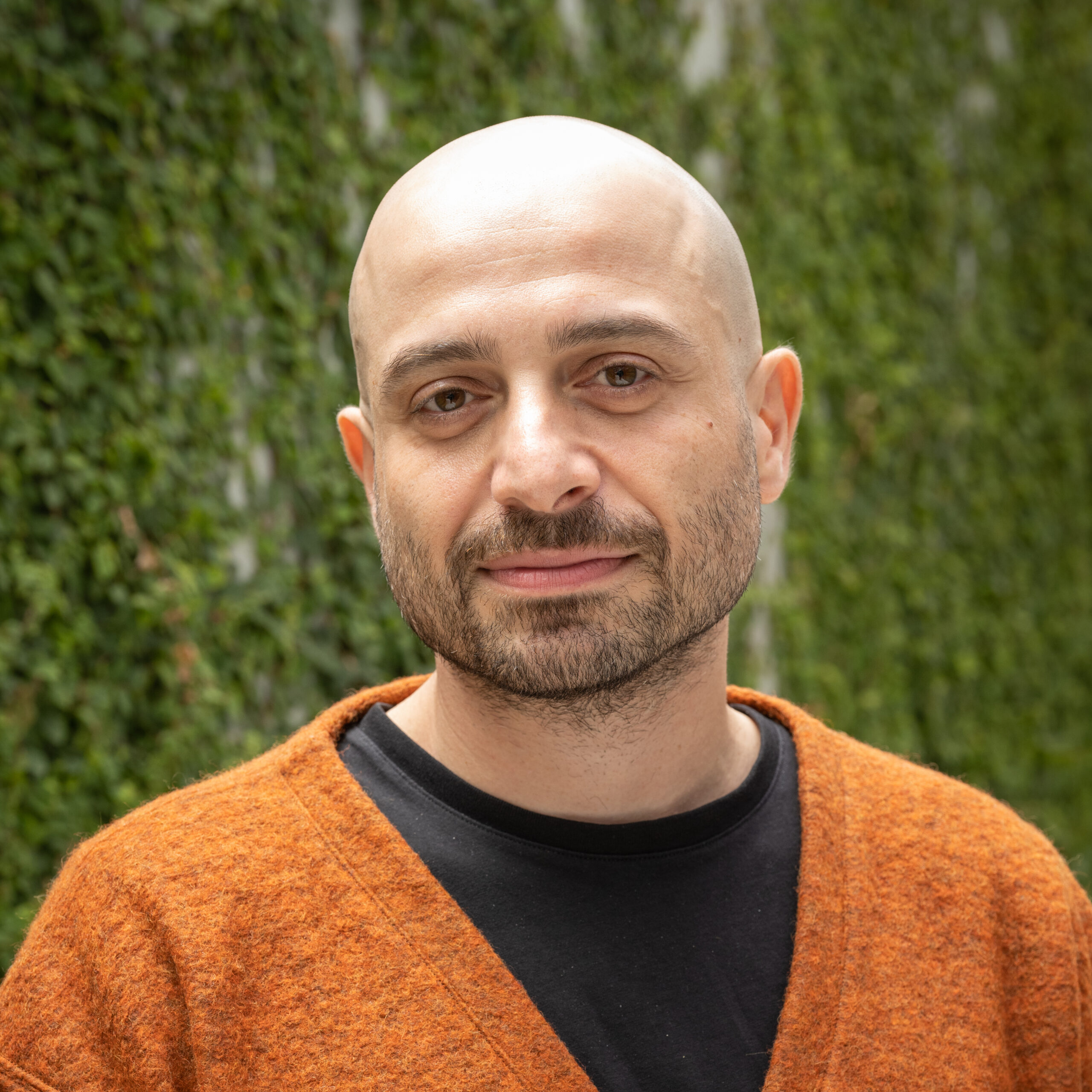
I ultimately would like to see the work that comes out of this project implemented in a domain where human-AI collaboration or AI-assisted decisions can be fruitful (e.g., healthcare). This requires finding partners with domain expertise, who also have better understanding of what is feasible given organizational constraints.