Dr. Gissella Bejarano is an Assistant Professor at Marist College. She received an AI2050 Early Career Fellowship in 2022 to help develop AI systems that can understand iconicity in American and Peruvian Sign Language. Iconicity is a property that some signs have, in which the sign looks like the concept that it represents, for example, “Eat,” which you can make by bunching the fingers in your hand and touching your mouth. Gissella’s work will advance Hard Problem 1 (Capabilities) by enabling AI to better understand sign language, and Hard Problem 6 (Access) by increasing access to and participation in AI for people who rely on sign language.
Learn more about Gissella Bejarano:
Can computers understand sign language today? Is it harder to understand than spoken language, or has there simply been less work done in the area?
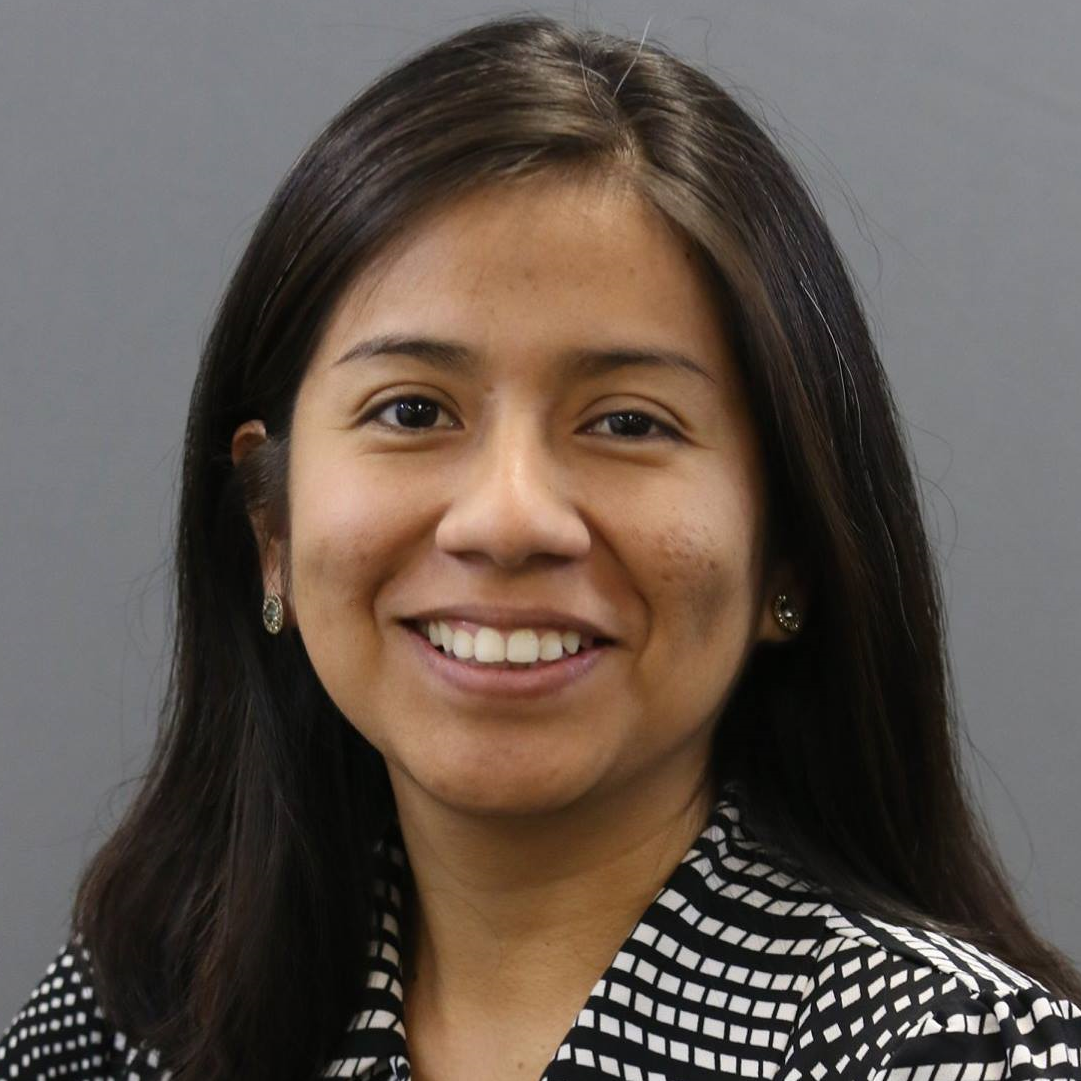
Today, computers understand few signs, signs performed with very controlled backgrounds or from very limited domains, such as weather forecasts.
Sign languages share similarities but have significant differences from spoken languages. Both have this sequential or time component that challenges us to save previously mentioned signs or words in sentences to infer the meaning of more recent ones. The difference is that the movement component of sign languages can only be acquired through computer vision, making it a multi-modal problem.
Can you share with us how you got interested in sign language?
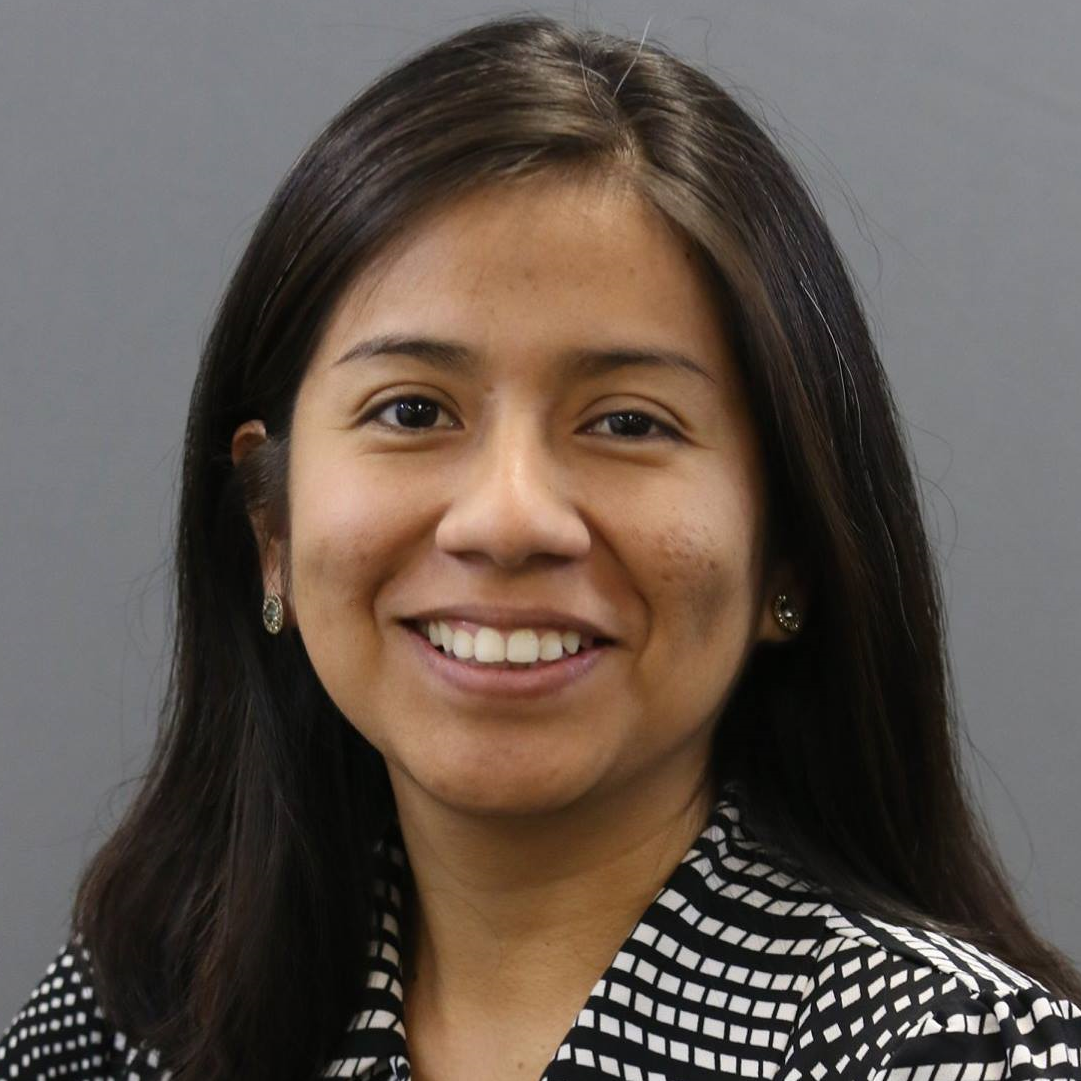
During my masters in 2016, I explored an Australian Sign Language dataset and applied deep learning AI techniques to recognize signs. That dataset came from a data glove, which captures how a person’s hand and fingers move. The problem with gloves is that they are invasive.
One day, I was watching the TV news and saw a sign language interpreter in a small white square at the bottom right of the screen. I realized that it might be possible to use the voice, the written transcript of the video, and the video of the interpreter to train a machine learning system to perform automatic recognition of sign language.
The COVID pandemic showed that deaf communities have very little access to health information, especially in developing countries. By then, I was nearly done with my Ph.D. at Binghamton University, so I decided to focus my postdoc at Baylor University on sign language processing.
How long did it take for you to learn sign language?
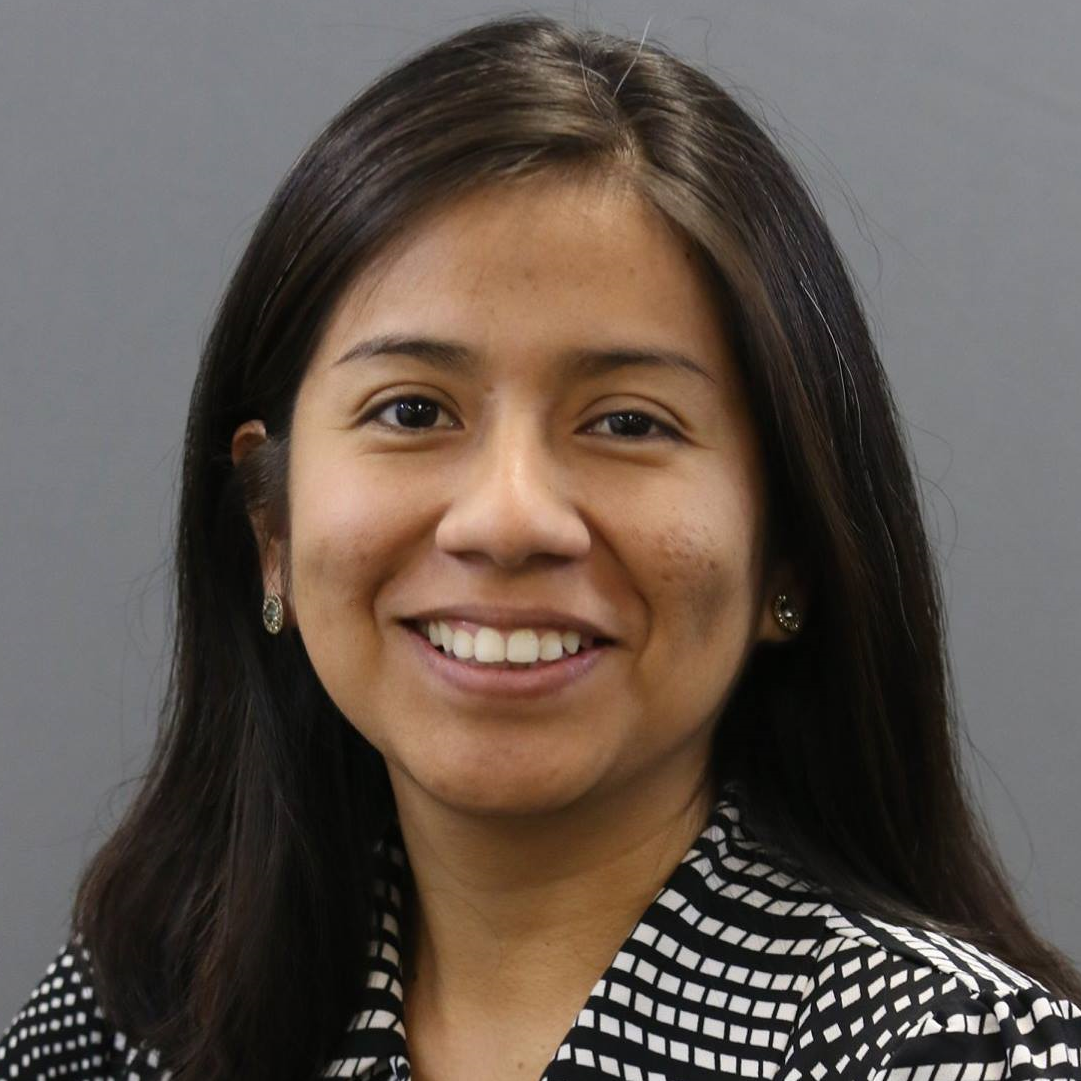
I have learned interesting facts about American and Peruvian Sign Language from Linguistics and Deaf studies researchers. However, I still do not speak any sign language; I just know a few signs!
Can you explain how American Sign Language and Peruvian Sign Language are different?
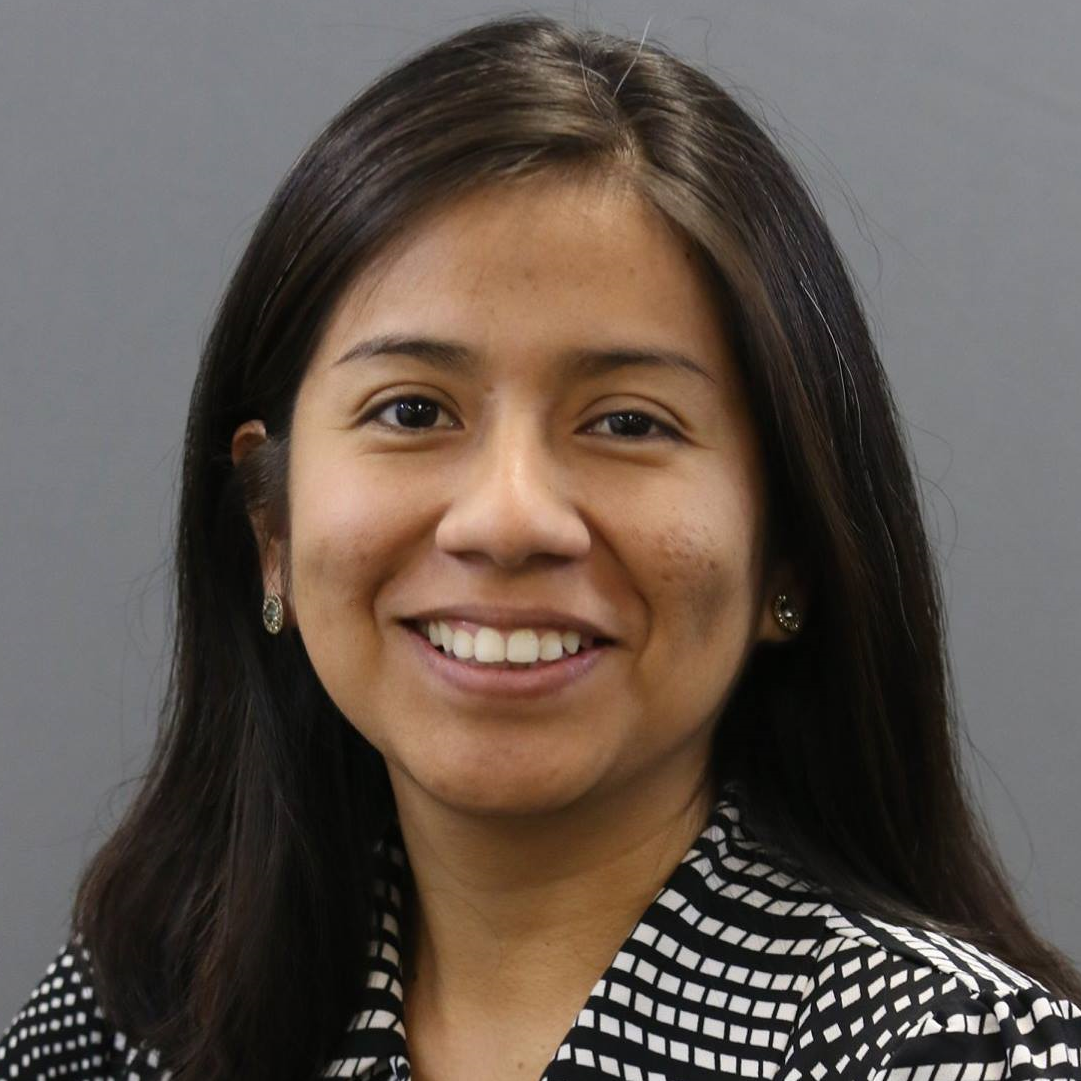
As oral languages, sign language similarities can be tracked due to grammatical, historical, and other analyses. A study by Brenda Clark in 2017 reports a 30% similarity between American Sign Language (ASL) and Peruvian Sign Language (LSP). However, these numbers should be interpreted carefully, given that some variants of LSP exist.
In the movie 2001 A Space Odyssey, the HAL-9000 computer can perform lip reading. Now, 2001 is fiction, but it is an idea that has captivated generations of researchers. Is lip reading harder or easier than understanding sign language for computers? Is high-fidelity lip reading even possible?
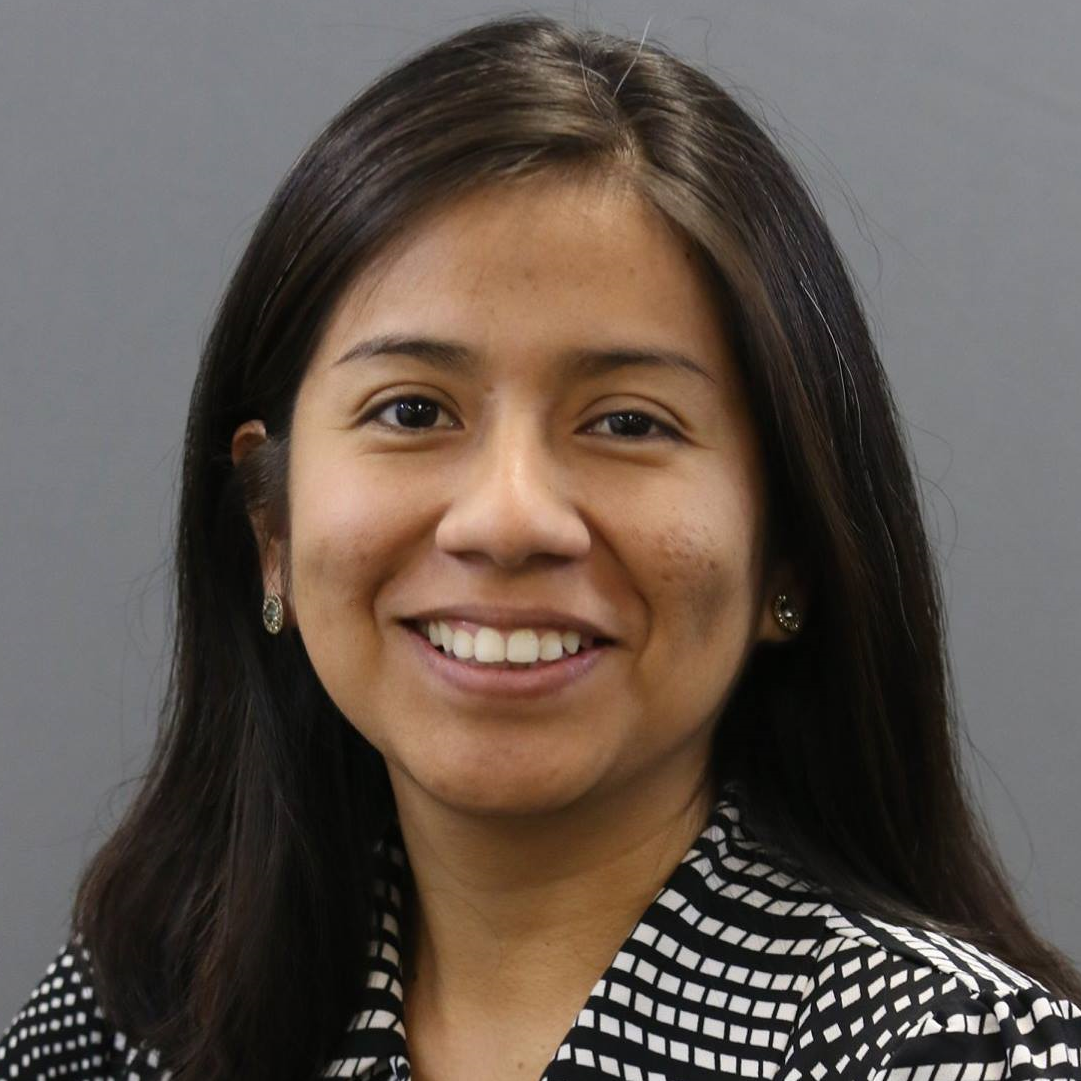
Deep learning models allow outstanding performance in any context where there are labels. In this case, videos from where we might get lip reading have also transcriptions or asynchronous labels in the captions of the videos. However, I do not know any high-fidelity lip-reading dataset. Suppose we have all these datasets, and along with voice recognition, we are still just solving part of the problem: translation from oral to sign languages. We need to allow native signers to communicate in their own language, so we are still unable to recognize signs and sign utterances.
How did the ECF grant from AI2050 help your research?
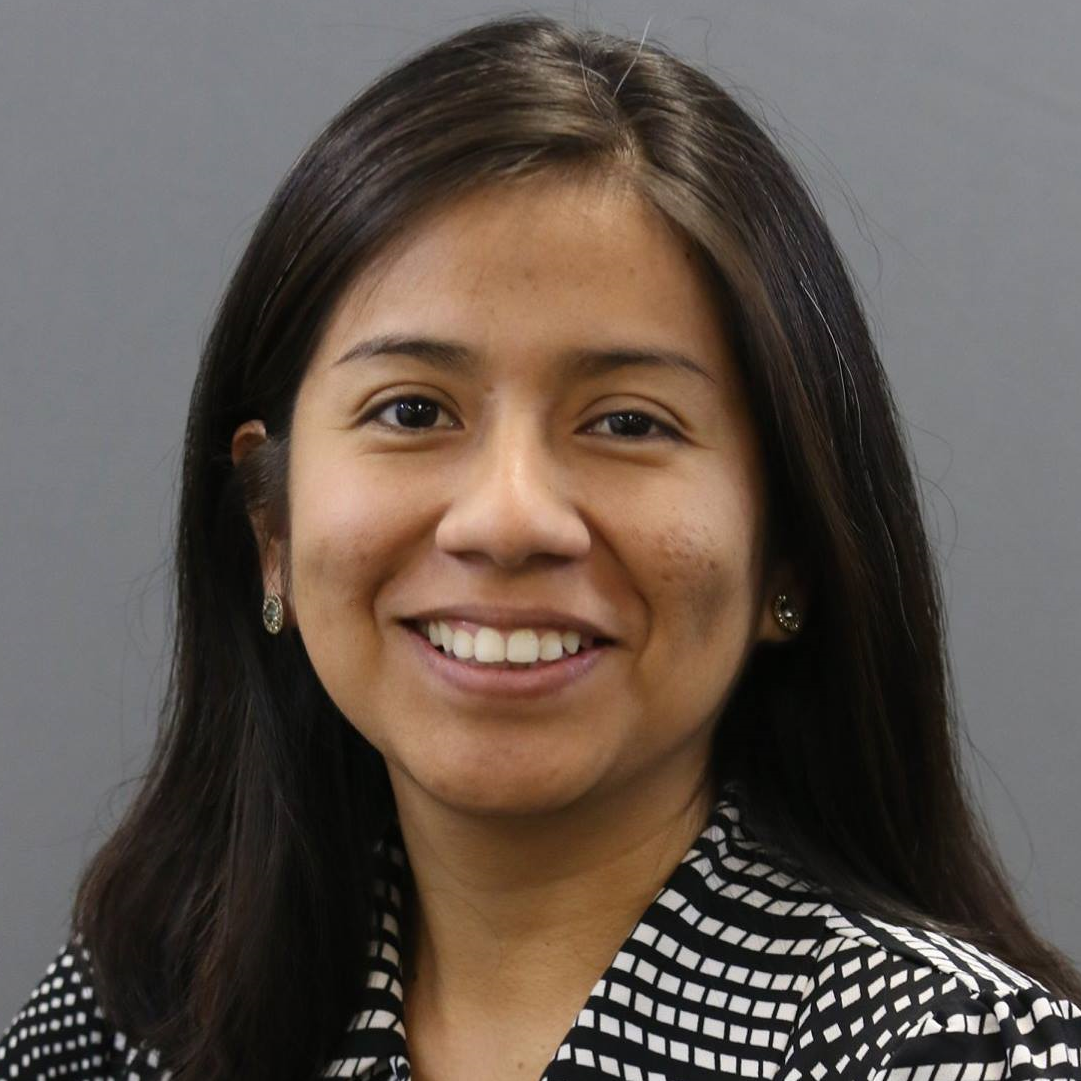
The ECF grant is helping my research team explore the recognition of iconicity in sign languages, motions, and gestures performed by deaf and hearing individuals. Iconicity is the property of a sign or gesture to match its meaning, and it plays a fundamental role in non-verbal communication. Findings in this area can pave the way to understanding analogical reasoning, other types of intelligence, the role of gesture in sign languages, and the origins of human expressions, motion, and culture. Moreover, an AI that recognizes and produces these features of human communication might become a new bar to testing a machine and considering it intelligent.
Wikipedia lists over 200 sign languages, and claims that there are more than 300 in existence. Are the approaches that you are working on extensible to sign languages other than ASL and PSL?
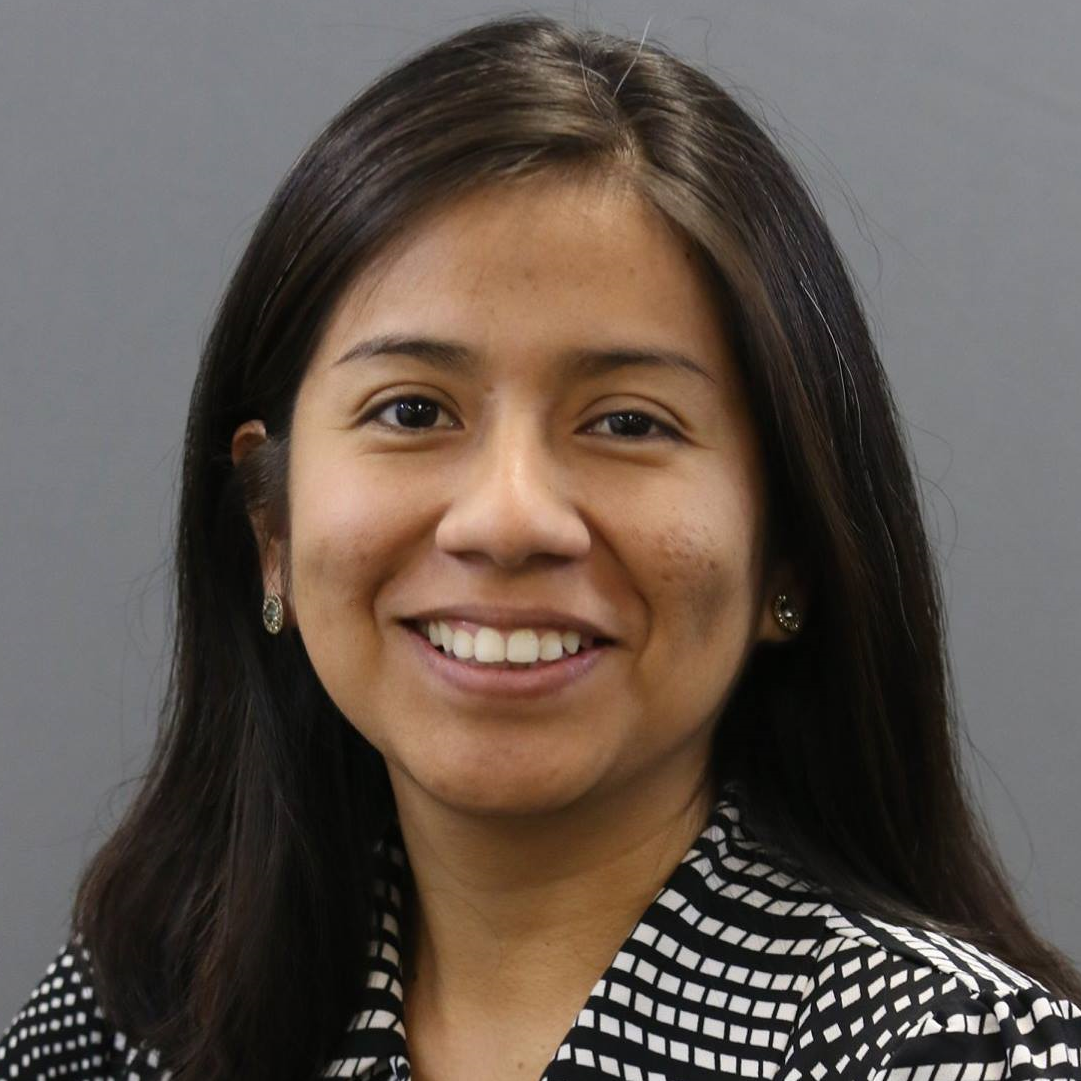
Yes. For example, we will train our model on these sign languages and test how well it recognizes gestures, or train it in one sign language and gestures performed by hearing people in one region and test how well it recognizes another sign language in another region. As all the signs and gestures are agnostic to words and oral languages, our model is supposed to learn specific iconicity features that can help understand other sign languages and gestures of different regions/cultures.
What are the next steps in your research?
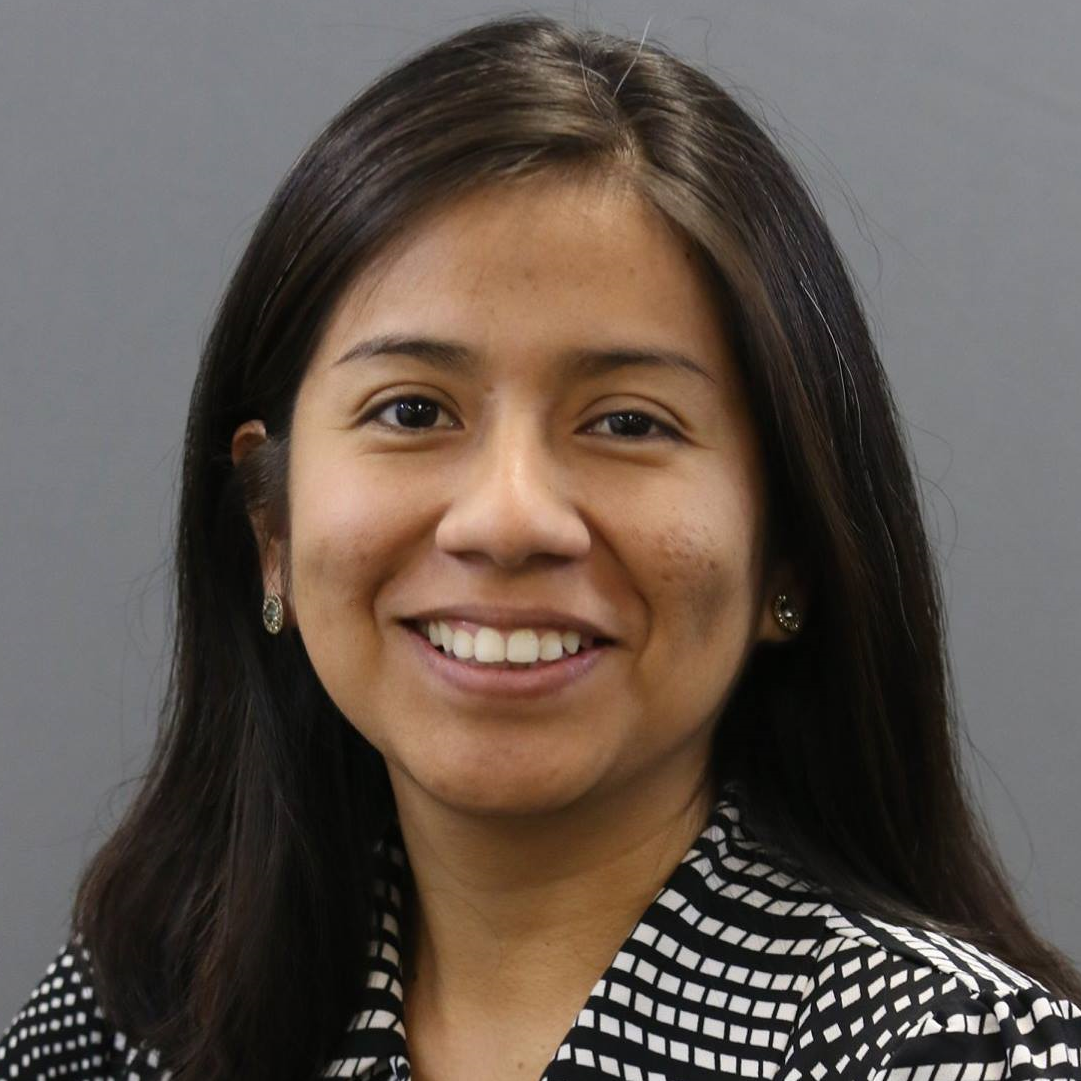
Achieve automatic multi-sign-language translation, like the ones for oral languages. We can rely on creating larger and cleaner datasets, building unsupervised methods for working with scarce resources of certain sign languages, or exploring more transfer learning. Ultimately our goal is to create sign language technology for and with the main stakeholders, deaf communities, so that they can communicate with computers using their native languages.